Zhu Et Al 2024 Vision Mamba Efficient Visual Representation Lear View pdf abstract: while large language models with vision capabilities (vlms), e.g., gpt 4o and gemini 1.5 pro, score high on many vision understanding benchmarks, they are still struggling with low level vision tasks that are easy to humans. specifically, on blindtest, our suite of 7 very simple tasks, including identifying (a) whether two. In this paper, we test vlms’ ability to (not reasoning) on low level vision tasks inspired by the “visual acuity” tests [5] given to humans by optometrists. simple visual tasks that involve only 2d geometric primitives ( ., lines and circles) [12] and require minimal to zero world knowledge. our key findings are: 1.
Avram Et Al 2024 Written Language A Promising Gateway To Anxiety This episode of cognitive spirals explores a controversial paper titled "vision language models are blind." the paper critiques current vision language mode. This paper examines the limitations of current vision based language models, such as gpt 4 and sonnet 3.5, in performing low level vision tasks. despite their high scores on numerous multimodal benchmarks, these models often fail on very basic cases. this raises a crucial question: are we evaluating these models accurately?. Large language models (llms) with vision capabilities (e.g., gpt 4o, gemini 1.5, and claude 3) are powering countless image text processing applications, enabling unprecedented multimodal, human machine interaction. Abstract: while large language models with vision capabilities (vlms), e.g., gpt 4o and gemini 1.5 pro, are powering various image text applications and scoring high on many vision understanding benchmarks, we find that they are surprisingly still struggling with low level vision tasks that are easy to humans. specifically, on blindtest, our.

Vision Language Models Are Blind Failing To Translate Detailed Visual Large language models (llms) with vision capabilities (e.g., gpt 4o, gemini 1.5, and claude 3) are powering countless image text processing applications, enabling unprecedented multimodal, human machine interaction. Abstract: while large language models with vision capabilities (vlms), e.g., gpt 4o and gemini 1.5 pro, are powering various image text applications and scoring high on many vision understanding benchmarks, we find that they are surprisingly still struggling with low level vision tasks that are easy to humans. specifically, on blindtest, our. Vlms (large language models with vision capabilities) fail on simple visual tasks that humans can easily perform. this paper aims to investigate and analyze the extent of this failure. the paper conducts experiments to evaluate the performance of four state of the art vlms on seven visual tasks that are easy for humans but challenging for vlms. While large language models with vision capabilities (vlms), e.g., gpt 4o and gemini 1.5 pro, are powering various image text applications and scoring high on many vision understanding benchmarks, we find that they are surprisingly still struggling with low level vision tasks that are easy to humans. While large language models with vision capabilities (vlms), e.g., gpt 4o andgemini 1.5 pro, are powering various image text applications and scoring highon many vision understanding benchmarks, we find that they are surprisinglystill struggling with low level vision tasks that are easy to humans.specifically, on blindtest, our suite of 7 very. However, current vision approaches for vlms are facing challenges as models sometimes are “blind”—unable to see natural objects exist in a real photo . in contrast, we are showing vlms are visually impaired at low level abstract images, e.g ., inability to count 6 overlapping circles or 3 nested squares.
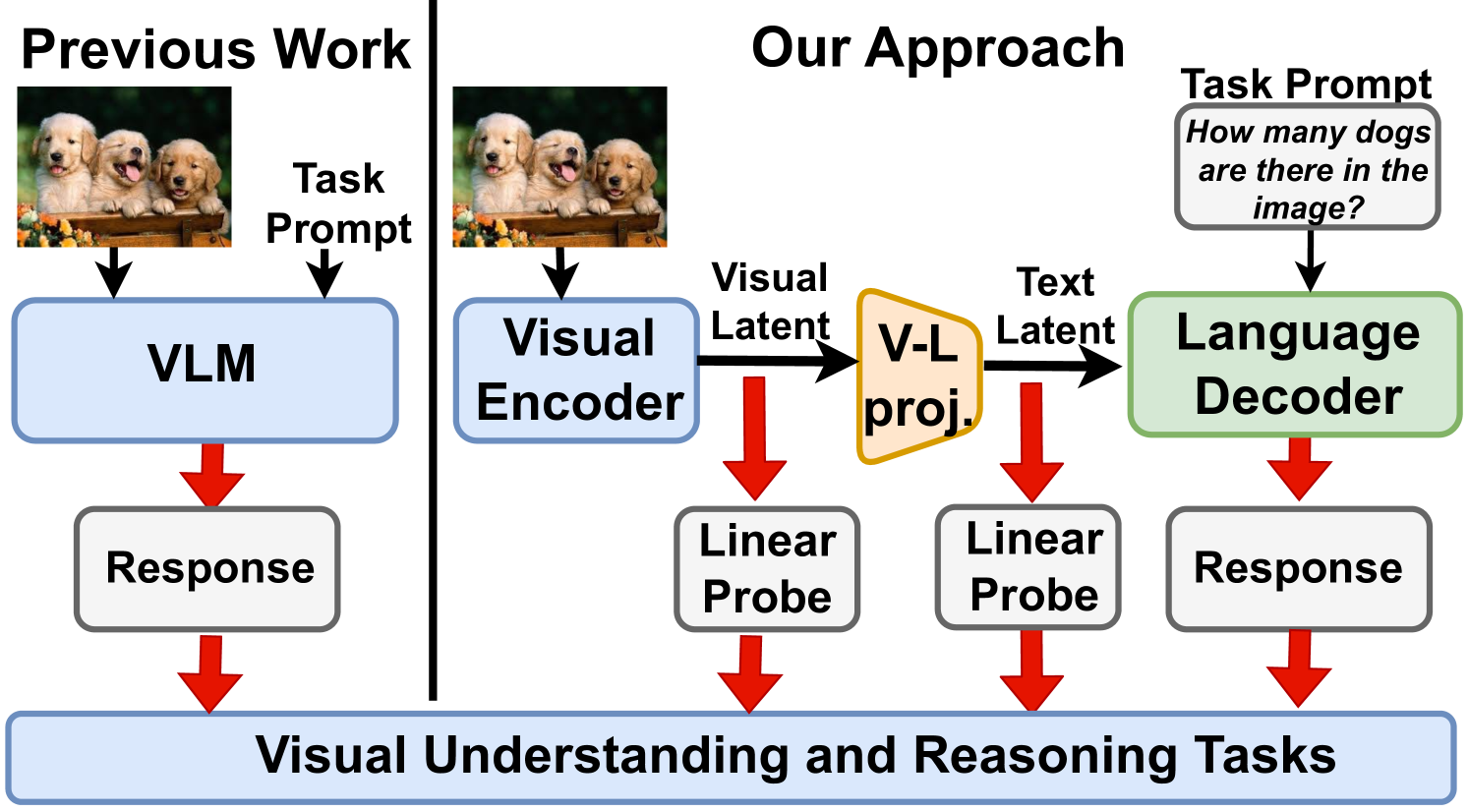
Vision Language Models Are Blind Ai Research Paper Details Vlms (large language models with vision capabilities) fail on simple visual tasks that humans can easily perform. this paper aims to investigate and analyze the extent of this failure. the paper conducts experiments to evaluate the performance of four state of the art vlms on seven visual tasks that are easy for humans but challenging for vlms. While large language models with vision capabilities (vlms), e.g., gpt 4o and gemini 1.5 pro, are powering various image text applications and scoring high on many vision understanding benchmarks, we find that they are surprisingly still struggling with low level vision tasks that are easy to humans. While large language models with vision capabilities (vlms), e.g., gpt 4o andgemini 1.5 pro, are powering various image text applications and scoring highon many vision understanding benchmarks, we find that they are surprisinglystill struggling with low level vision tasks that are easy to humans.specifically, on blindtest, our suite of 7 very. However, current vision approaches for vlms are facing challenges as models sometimes are “blind”—unable to see natural objects exist in a real photo . in contrast, we are showing vlms are visually impaired at low level abstract images, e.g ., inability to count 6 overlapping circles or 3 nested squares.

Demystifying Vision Language Models An In Depth Exploration Marktechpost While large language models with vision capabilities (vlms), e.g., gpt 4o andgemini 1.5 pro, are powering various image text applications and scoring highon many vision understanding benchmarks, we find that they are surprisinglystill struggling with low level vision tasks that are easy to humans.specifically, on blindtest, our suite of 7 very. However, current vision approaches for vlms are facing challenges as models sometimes are “blind”—unable to see natural objects exist in a real photo . in contrast, we are showing vlms are visually impaired at low level abstract images, e.g ., inability to count 6 overlapping circles or 3 nested squares.

Enhancing Vision Language Models Addressing Multi Object Hallucination