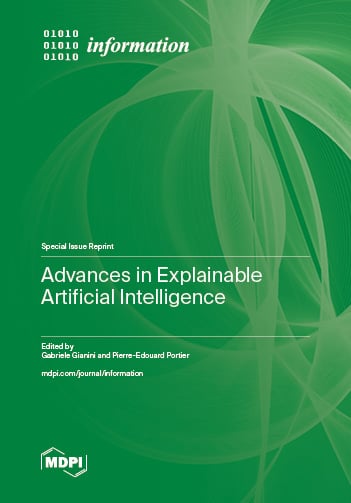
Advances In Explainable Artificial Intelligence Mdpi Books Machine learning (ml) based artificial intelligence (ai) algorithms have the capability to learn from known examples, creating various abstract representations and models. In this paper, we present the potential of explainable artificial intelligence methods for decision support in medical image analysis scenarios. using three types of explainable methods applied to the same medical image data set, we aimed to improve the comprehensibility of the decisions provided by the convolutional neural network (cnn).
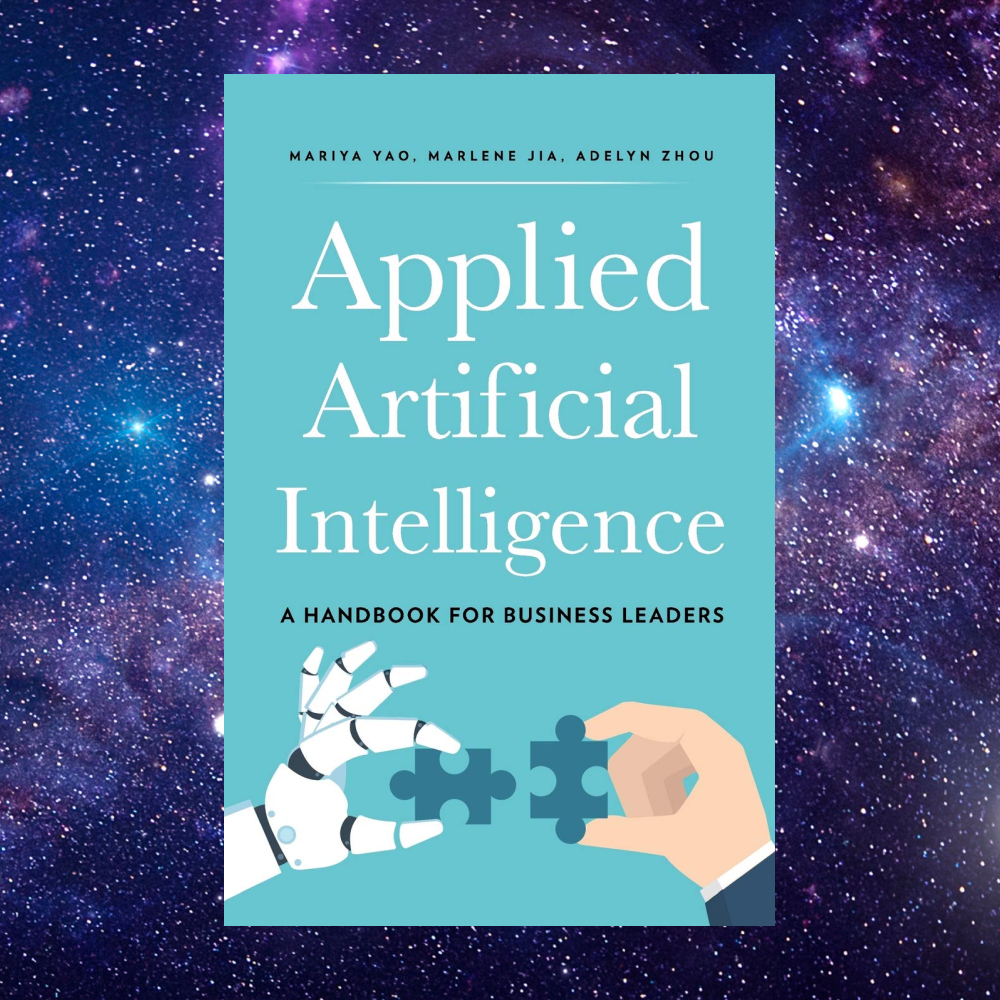
Applied Artificial Intelligence Book Welcome Ai The need to make ml algorithms more transparent and more explainable has generated several lines of research that form an area known as explainable artificial intelligence (xai). This necessity has prompted extensive research efforts aimed at enhancing the transparency and interpretability of ml algorithms, forming the field of explainable artificial intelligence (xai). In response, explainable ai (xai) has emerged as a field of research with practical and ethical benefits across various domains. this paper highlights the advancements in xai and its application in real world scenarios and addresses the ongoing challenges within xai, emphasizing the need for broader perspectives and collaborative efforts. Modern research in xai targets to develop more explainable or interpretable intelligent models while maintaining the desired level of accuracy or performance. these models will help us in understanding, believe, and effectively manage the new era of ai enabled systems across the domains.

Advances In Artificial Intelligence Systems Nova Science Publishers In response, explainable ai (xai) has emerged as a field of research with practical and ethical benefits across various domains. this paper highlights the advancements in xai and its application in real world scenarios and addresses the ongoing challenges within xai, emphasizing the need for broader perspectives and collaborative efforts. Modern research in xai targets to develop more explainable or interpretable intelligent models while maintaining the desired level of accuracy or performance. these models will help us in understanding, believe, and effectively manage the new era of ai enabled systems across the domains. We investigated the scopus, web of science, ieee xplore and pubmed databases, to find the pertinent publications published between january 2018 to october 2022. it contains the published research on xai modelling that were retrieved from scholarly databases using pertinent keyword searches. Recent advancements in explainable artificial intelligence (xai) aim to bridge the gap between complex artificial intelligence (ai) models and human understanding, fostering trust and usability in ai systems. The importance of explainable artificial intelligence and machine learning (xai xml) is increasingly being recognized, aiming to understand how information contributes to decisions, the method’s bias, or sensitivity to data pathologies. This book takes an in depth approach to present the fundamentals of interpretable and explainable ai through mathematical theory and practical use cases.