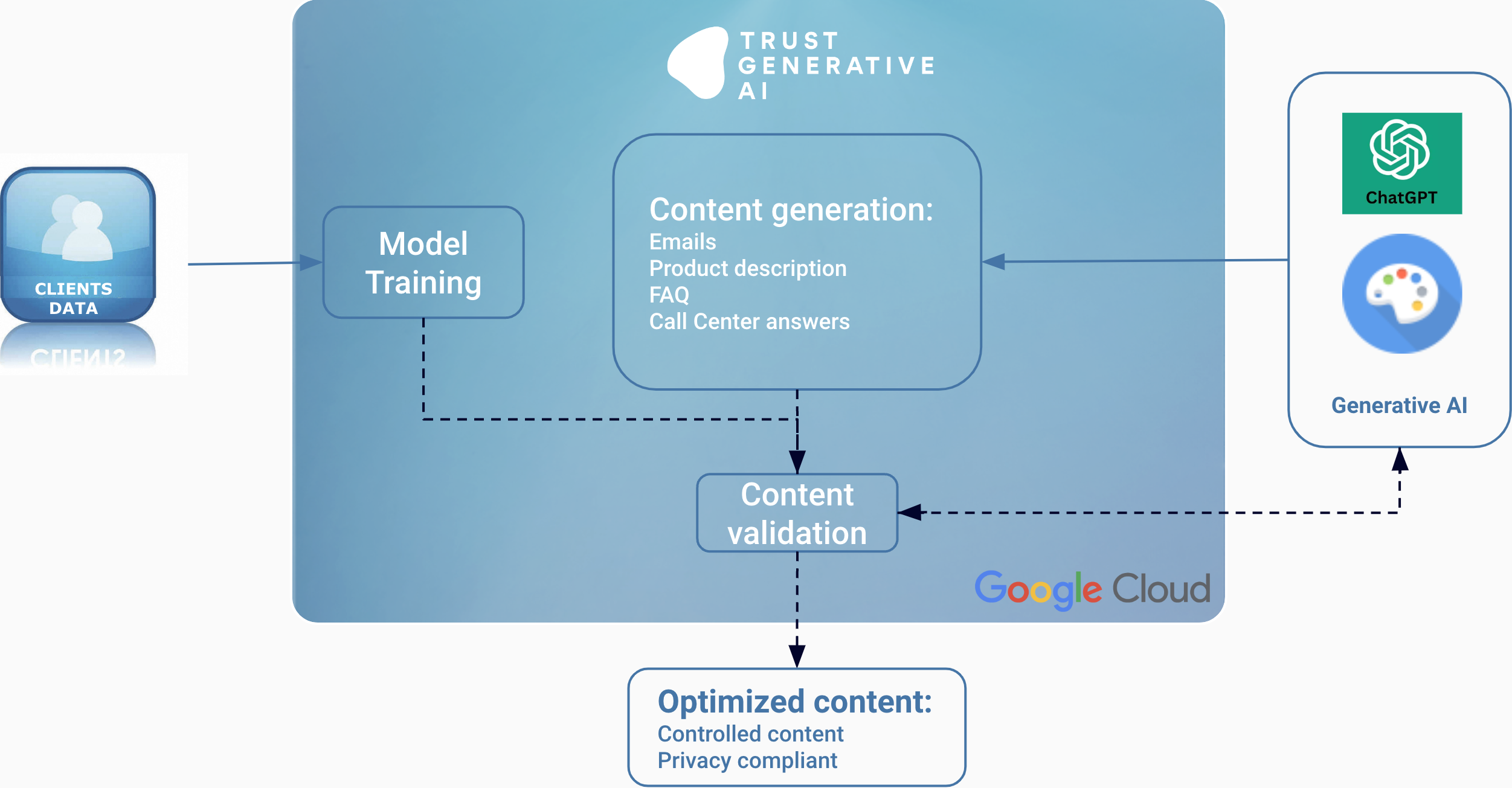
Trust Generative Ai Building trust in the generative artificial intelligence era: technology challenges and innovations explores how trust can be built, maintained, and evaluated in a world increasingly reliant on ai technologies. designed to be accessible to a broad audience, the book blends theoretical insights with practical approaches, offering readers a. We believe trust and transparency are key to unlocking learning potential, and we’ll always prioritize customer safety and satisfaction. learn more about how go1 is using ai to enhance learning here .
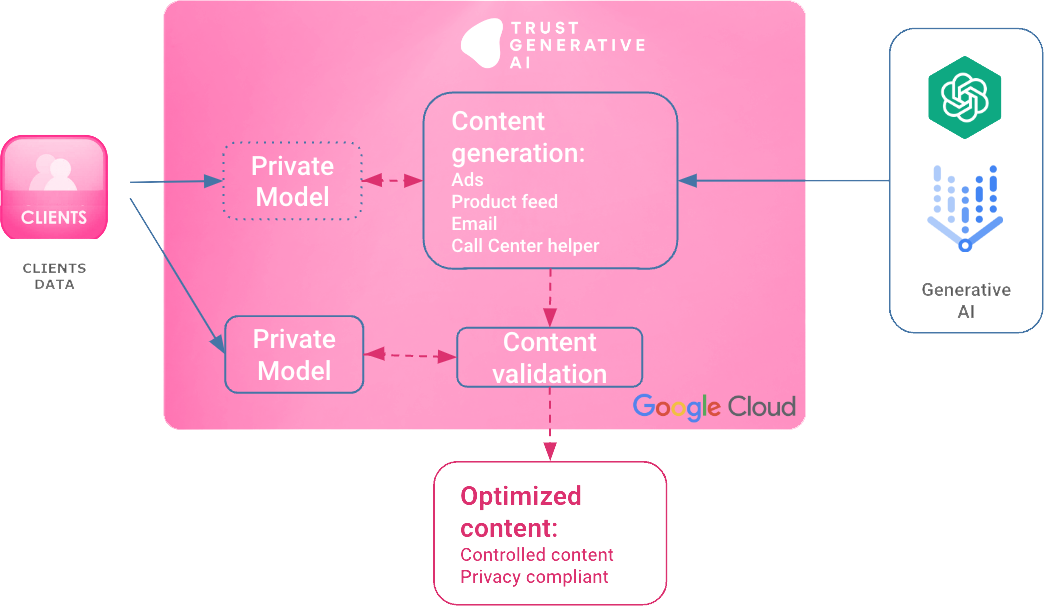
Trust Generative Ai This article delivers an elaborate exploration of the drivers affecting trust in ai systems deployed for educational purposes by carefully examining trustworthiness, perceived dangers, and. Data privacy and security are critical for building trust, with 66 percent of genai users in our sample citing them as crucial. developing robust frameworks, in collaboration with compliance, risk, and privacy teams, is essential for mitigating these risks. organizations must recognize that handling consumer data ethically is not optional. As artificial intelligence (ai) becomes increasingly integrated into critical sectors, the need for transparency and trust in ai systems has grown significantly. this paper presents a systematic review of explainable ai (xai) and its role in aligning ai development with human values, particularly addressing the ethical concerns surrounding fairness, accountability and bias. xai refers to a set. How to build trust and transparency in your generative ai implementation. as more businesses look to harness the power of generative ai technologies, our expert suggests they would be wise to package them with intelligent automation tools for trust purposes.
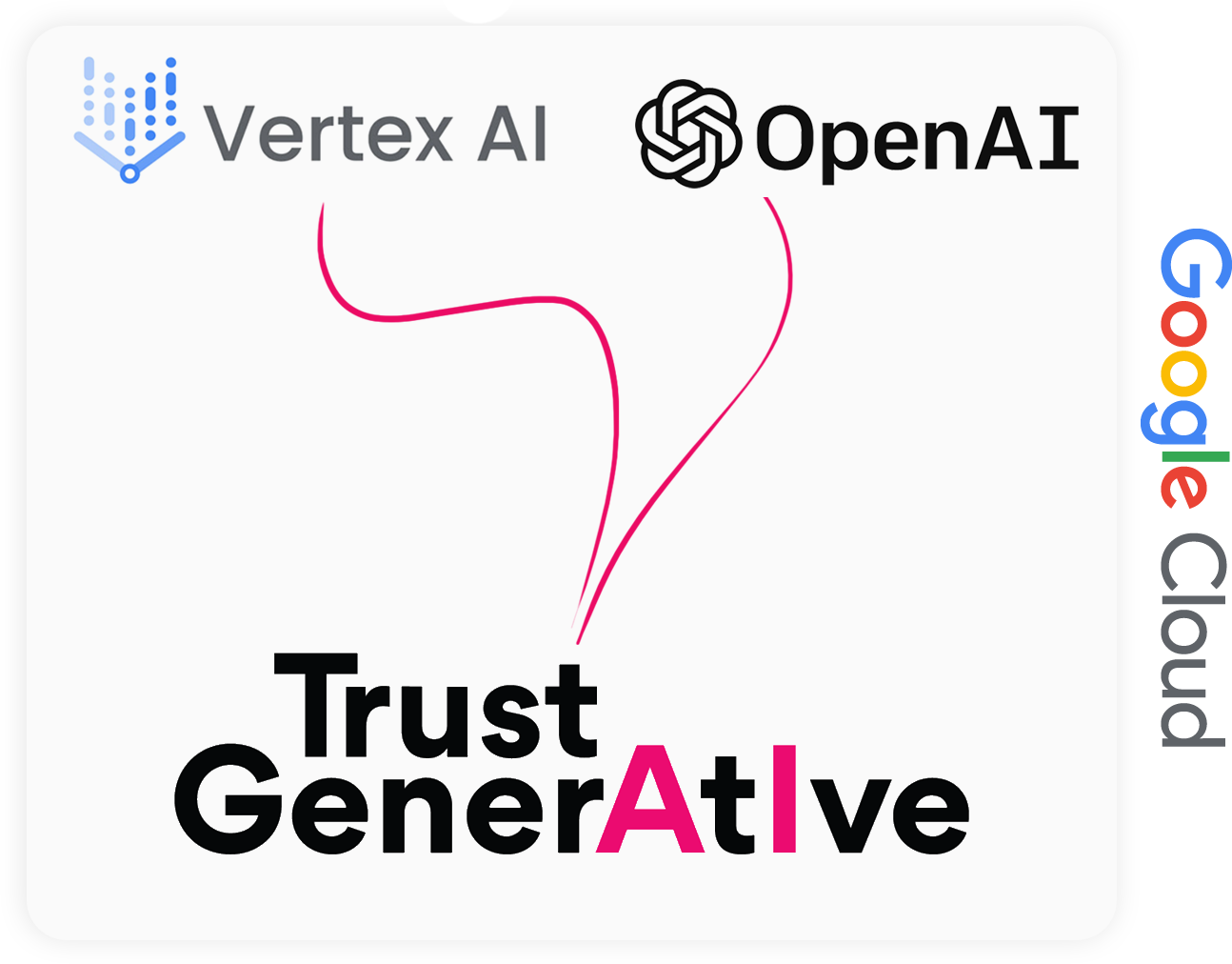
Trust Generative Ai As artificial intelligence (ai) becomes increasingly integrated into critical sectors, the need for transparency and trust in ai systems has grown significantly. this paper presents a systematic review of explainable ai (xai) and its role in aligning ai development with human values, particularly addressing the ethical concerns surrounding fairness, accountability and bias. xai refers to a set. How to build trust and transparency in your generative ai implementation. as more businesses look to harness the power of generative ai technologies, our expert suggests they would be wise to package them with intelligent automation tools for trust purposes. Now, in part ii, we turn our attention to one of the most critical aspects of ai deployment: building trust and transparency. this article explores essential strategies for enhancing model interpretability, addressing ai hallucinations, and fostering clear communication to improve user understanding and confidence. Ai observability: ensuring trust and transparency in machine learning pipelines. rambabu bandam. published 04 23 2025 looking ahead, future advancements may include greater automation, enhanced explainability, and closer integration of generative ai and observability platforms. continued innovation will align ai systems more closely with. Communicating limitations and appropriate use cases for the ai system; transparency helps build trust not by eliminating complexity, but by refusing to hide behind it. governed ai. transparent ai opens the curtains, but governed ai is what keeps the whole system on track. this layer is about putting in place the policies, processes, and. We have mapped out a roadmap for human centered research on ai transparency in the era of llms by reflecting on the unique challenges introduced by llms, synthesizing lessons learned from hci fate research on ai transparency, and exploring the applicability of existing transparency approaches—model reporting, publishing evaluation results.
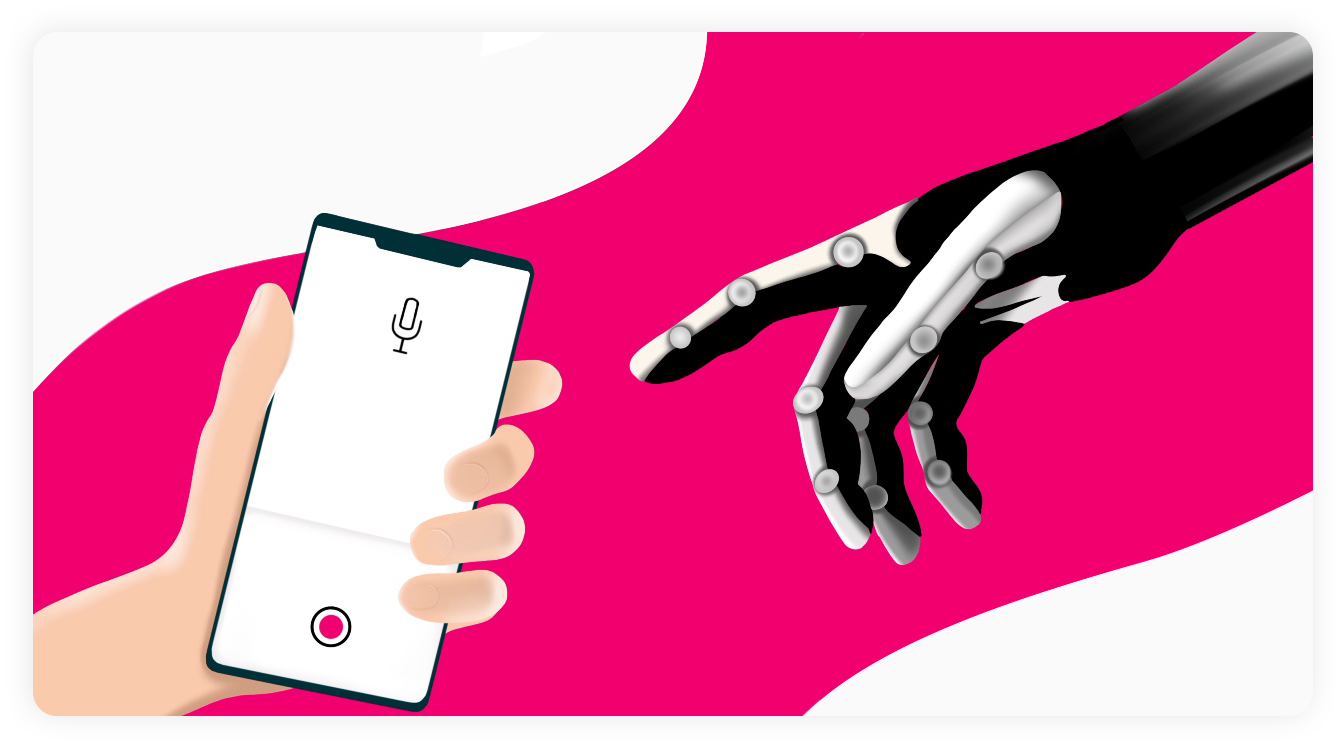
Trust Generative Ai Now, in part ii, we turn our attention to one of the most critical aspects of ai deployment: building trust and transparency. this article explores essential strategies for enhancing model interpretability, addressing ai hallucinations, and fostering clear communication to improve user understanding and confidence. Ai observability: ensuring trust and transparency in machine learning pipelines. rambabu bandam. published 04 23 2025 looking ahead, future advancements may include greater automation, enhanced explainability, and closer integration of generative ai and observability platforms. continued innovation will align ai systems more closely with. Communicating limitations and appropriate use cases for the ai system; transparency helps build trust not by eliminating complexity, but by refusing to hide behind it. governed ai. transparent ai opens the curtains, but governed ai is what keeps the whole system on track. this layer is about putting in place the policies, processes, and. We have mapped out a roadmap for human centered research on ai transparency in the era of llms by reflecting on the unique challenges introduced by llms, synthesizing lessons learned from hci fate research on ai transparency, and exploring the applicability of existing transparency approaches—model reporting, publishing evaluation results.
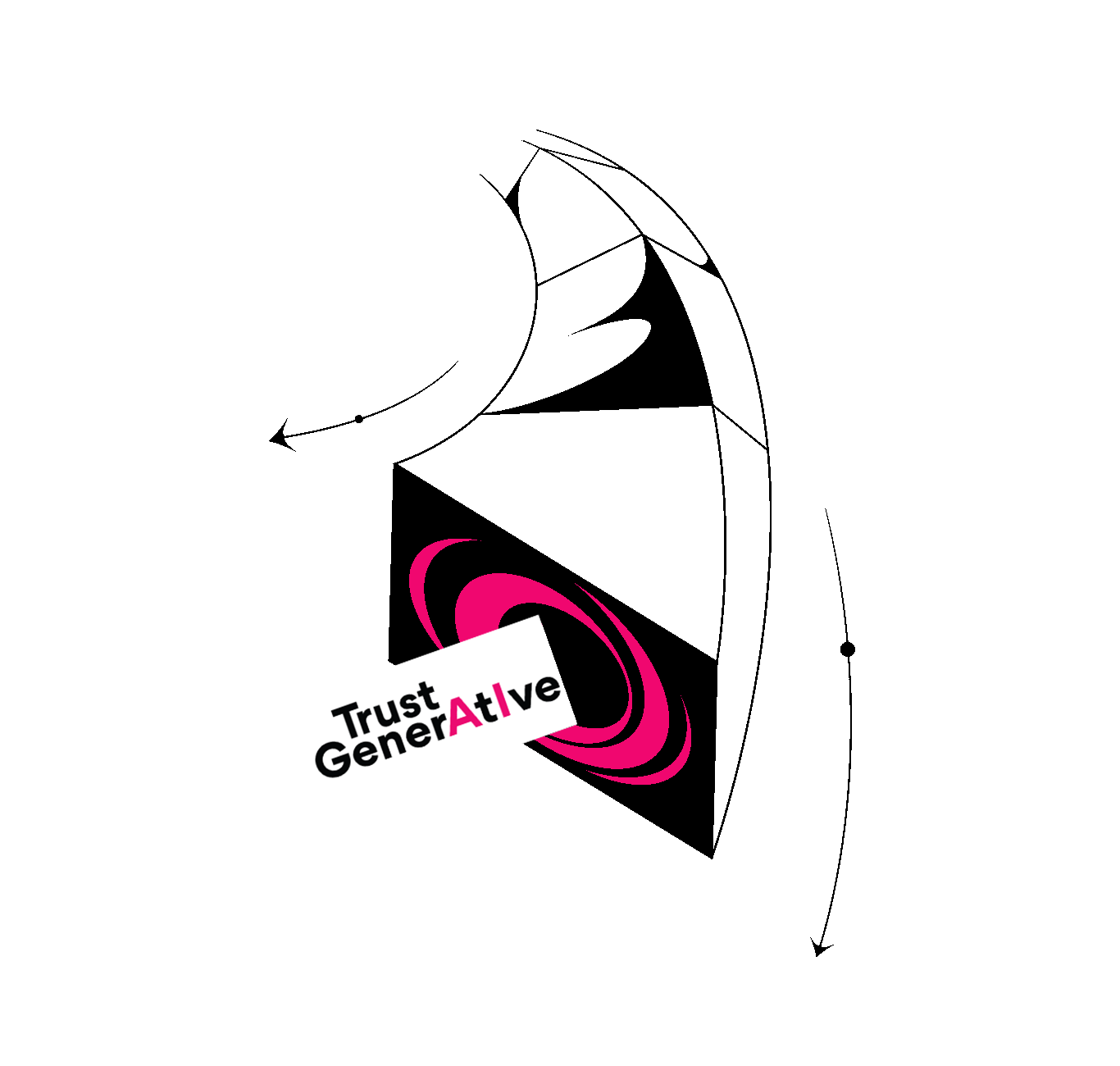
Trust Generative Ai Communicating limitations and appropriate use cases for the ai system; transparency helps build trust not by eliminating complexity, but by refusing to hide behind it. governed ai. transparent ai opens the curtains, but governed ai is what keeps the whole system on track. this layer is about putting in place the policies, processes, and. We have mapped out a roadmap for human centered research on ai transparency in the era of llms by reflecting on the unique challenges introduced by llms, synthesizing lessons learned from hci fate research on ai transparency, and exploring the applicability of existing transparency approaches—model reporting, publishing evaluation results.