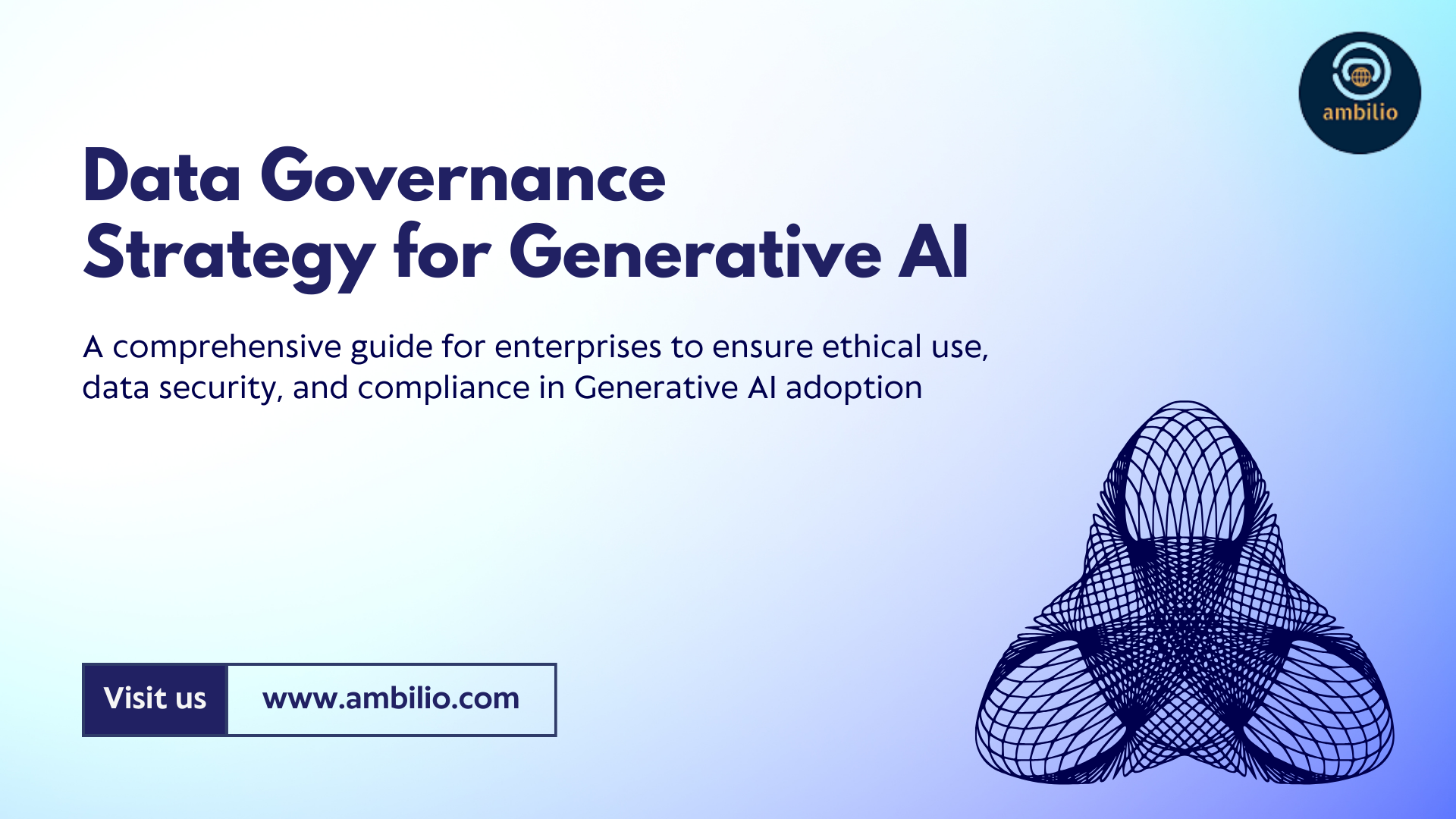
Data Governance Strategy For Generative Ai Adoption Introducing the deloitte ai governance roadmap the deloitte ai governance roadmap (“roadmap”) is designed to help boards of directors (“boards”) understand their role and provide them with guiding questions to support effective oversight of ai. the roadmap applies the deloitte governance framework (“framework”) to ai. the framework, illustrated below, provides an end to end view of. A well structured data governance strategy for generative ai ensures the responsible and ethical use of data, which underpins the success of ai models. enterprises must address various challenges in data quality , security , privacy , and compliance while leveraging genai’s potential .

Data Governance And Generative Ai Dan Galavan Widespread adoption and demand for the use of ai in business are showing no signs of slowing down. amid rising investments in and focus on ai applications, particularly with the rise of generative ai, companies are facing increasing pressure to adopt and implement ai quickly in their business models.but in the race to adopt ai, you can’t overlook the necessity of establishing strong ai. Effective data governance rests on four pillars: data visibility: clarify available data assets to inform decision making. access control: balance accessibility with security. quality assurance: ensure data reliability for accurate analytics. With the emergence of generative ai, organisations are facing new challenges and opportunities in managing their data assets. this paper (over two parts) explores the intersection of data governance and generative ai, examining the traditional data governance model and its evolution to support generative ai. Investment is increasing, but the clock is ticking to create value. the q3 edition of the state of generative ai in the enterprise, moving from potential to performance, reveals how organizations are navigating challenges and measuring value, focusing on two areas critical to scaling: data and governance, risk and compliance. view q3 report.
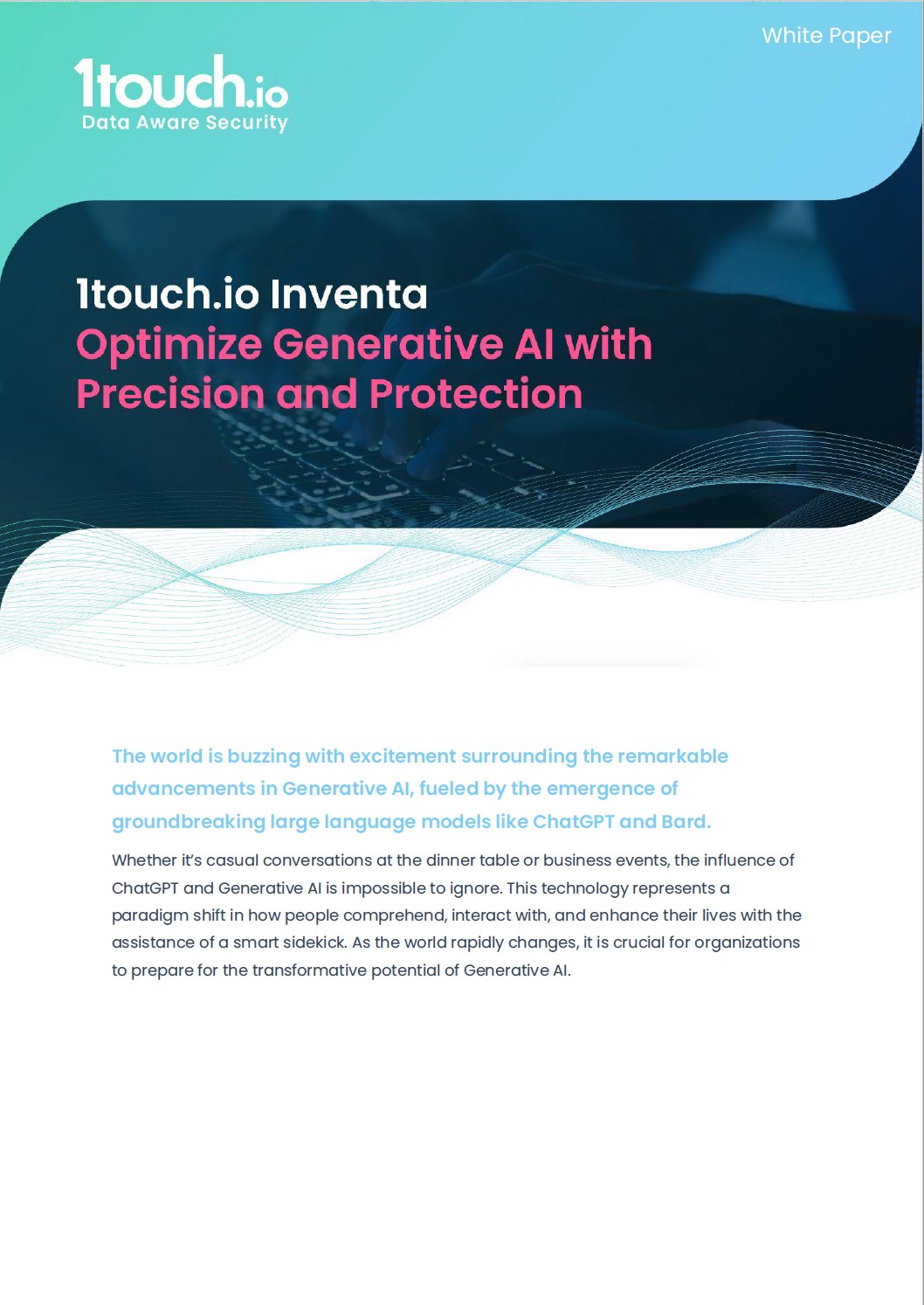
Generative Ai Sensitive Data Protection 1touch Io With the emergence of generative ai, organisations are facing new challenges and opportunities in managing their data assets. this paper (over two parts) explores the intersection of data governance and generative ai, examining the traditional data governance model and its evolution to support generative ai. Investment is increasing, but the clock is ticking to create value. the q3 edition of the state of generative ai in the enterprise, moving from potential to performance, reveals how organizations are navigating challenges and measuring value, focusing on two areas critical to scaling: data and governance, risk and compliance. view q3 report. Gen ai represents new challenges in data governance, but also new requirements to existing data quality and data engineering and management functions. To truly harness generative ai, microsoft identifies five key drivers of ai value that separate industry leaders from laggards. a strong business strategy is the foundation of successful generative ai adoption. organisations should: define the business problems ai will solve. identify high impact use cases. develop a roadmap for ai investment. Embracing unstructured data: generative ai thrives on unstructured data like text, images, and code. data governance frameworks must adapt to effectively manage and secure these diverse data types, moving beyond the structured databases of the past. Generative ai enhances data governance by overcoming historical challenges, such as poor data quality and the complexity of large systems, making data management simpler and more efficient.