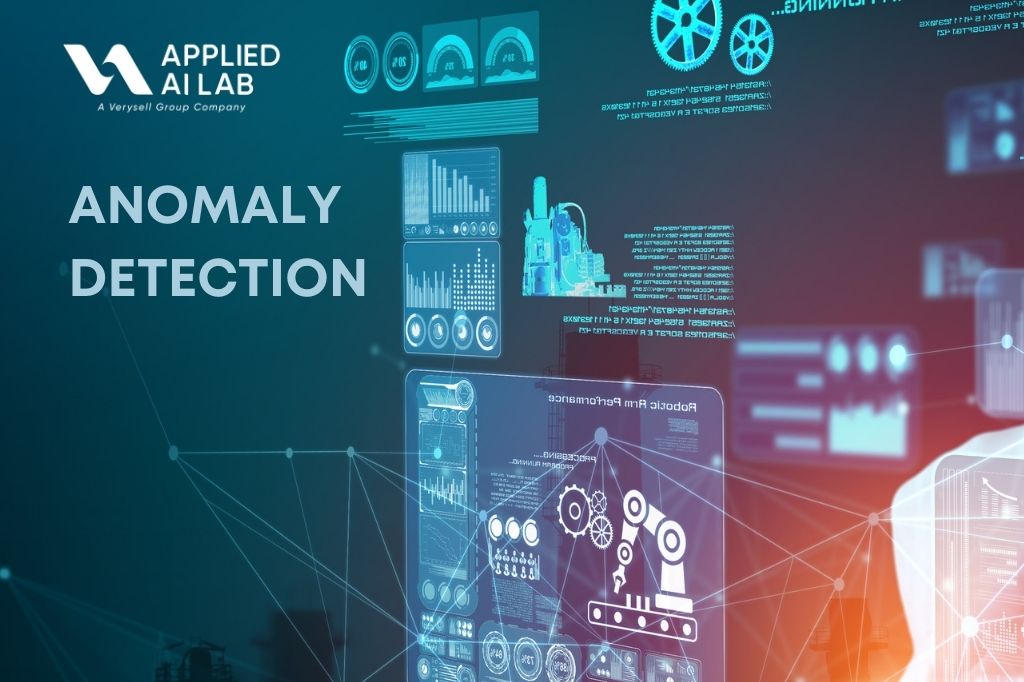
Anomaly Detection Verysell Group Applied Ai Lab We propose a novel visualization technique (eagle eye) for intrusion detection, which visualizes a host as a commu nity of system call traces in two dimensional space. the goal of eagleeye is to visually cluster the system call traces. About press copyright contact us creators advertise developers terms privacy policy & safety how works test new features nfl sunday ticket press copyright.
Github Guetye Anomaly Detection Test Video Of The Proposed Method In In this talk, the speaker talks about the high availability and highly scalable data pipelines that he built for the following use cases : * denial of service: a device in the network stops working. * data corruption : a device starts sending erroneous data. the above can be solved through anomaly detection models. Such anomalies can indicate a critical system failure, lead to a groundbreaking scientific discovery, or reveal unexpected changes in data distribution. we introduce eagleeye, an anomaly detection method to compare two multivariate datasets with the aim of identifying local density anomalies, namely over or under densities affecting only. In the eagleeye paper, we train a transformer model from scratch, on the process behavior dataset. the goal of the transformer is to predict whether a sequence of behavior events belongs to a benign or malicious computer application. 在darpa数据集上,以1%的误报率为基础,eagleeye检测到了约89%的所有恶意行为,优于两个最先进的解决方案,绝对差距为38.5%。 此外,我们展示了transformer的注意机制可以用来突出显示长序列中最可疑的事件,从而提供恶意软件警报的解释。 本论文旨在解决端点安全面临的挑战,即威胁和攻击的不断演变。 作者提出了eagleeye系统,旨在利用丰富的特征和transformer模型来识别恶意行为序列。 eagleeye系统的关键思路是利用丰富的特征从行为事件中提取长序列,并使用transformer模型对恶意行为进行分类。 这种方法相比当前领域的研究具有创新性。 论文使用了两个数据集进行评估,一个是来自企业环境的新数据集,另一个是公共的darpa数据集。.
Github Meetrajput00 Anomaly Detection In the eagleeye paper, we train a transformer model from scratch, on the process behavior dataset. the goal of the transformer is to predict whether a sequence of behavior events belongs to a benign or malicious computer application. 在darpa数据集上,以1%的误报率为基础,eagleeye检测到了约89%的所有恶意行为,优于两个最先进的解决方案,绝对差距为38.5%。 此外,我们展示了transformer的注意机制可以用来突出显示长序列中最可疑的事件,从而提供恶意软件警报的解释。 本论文旨在解决端点安全面临的挑战,即威胁和攻击的不断演变。 作者提出了eagleeye系统,旨在利用丰富的特征和transformer模型来识别恶意行为序列。 eagleeye系统的关键思路是利用丰富的特征从行为事件中提取长序列,并使用transformer模型对恶意行为进行分类。 这种方法相比当前领域的研究具有创新性。 论文使用了两个数据集进行评估,一个是来自企业环境的新数据集,另一个是公共的darpa数据集。. Above: multimodal over densities (red) and under densities (purple) captured by $\texttt{eagleeye}$. eagleeye is a novel, density based anomaly detection method designed for comparing two datasets to identify deviations in the test dataset. by analyzing the local neighborhood of each point in the. We propose a novel graphical technique (svision) for intrusion detection, which pictures the network as a community of hosts independently roaming in a 3d space defined by the set of services. It discusses moving from basic network monitoring to a data mesh architecture enabling closed loop network operations. key developments include onboarding more platforms and metrics, anomaly detection, visualization, and collaborating with ietf on standards like bgp monitoring protocol, ipfix, and yang push notifications. Eagleeye can not only detect malware at high accuracy, but it can also explain why an application is malicious; in other words, it offers interpretability. to achieve this goal, we leverage the transformer model’s attention mechanism, which learns to put the highest attention on the most suspicious behavior events.
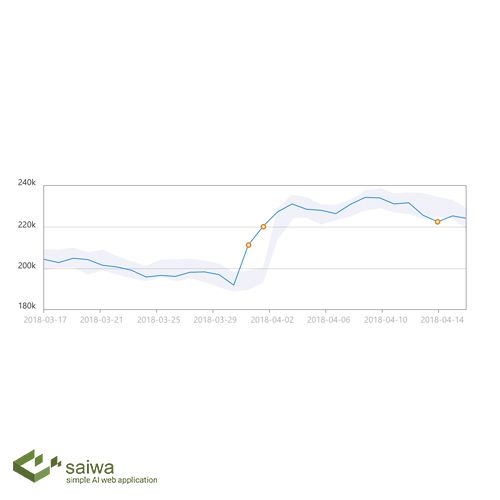
Anomaly Detection Online Demo Saiwa Above: multimodal over densities (red) and under densities (purple) captured by $\texttt{eagleeye}$. eagleeye is a novel, density based anomaly detection method designed for comparing two datasets to identify deviations in the test dataset. by analyzing the local neighborhood of each point in the. We propose a novel graphical technique (svision) for intrusion detection, which pictures the network as a community of hosts independently roaming in a 3d space defined by the set of services. It discusses moving from basic network monitoring to a data mesh architecture enabling closed loop network operations. key developments include onboarding more platforms and metrics, anomaly detection, visualization, and collaborating with ietf on standards like bgp monitoring protocol, ipfix, and yang push notifications. Eagleeye can not only detect malware at high accuracy, but it can also explain why an application is malicious; in other words, it offers interpretability. to achieve this goal, we leverage the transformer model’s attention mechanism, which learns to put the highest attention on the most suspicious behavior events.
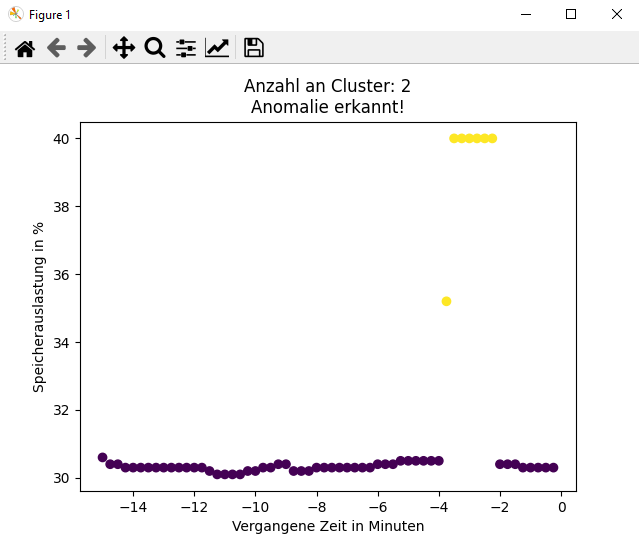
Github Codeleo99 Anomaly Detection It discusses moving from basic network monitoring to a data mesh architecture enabling closed loop network operations. key developments include onboarding more platforms and metrics, anomaly detection, visualization, and collaborating with ietf on standards like bgp monitoring protocol, ipfix, and yang push notifications. Eagleeye can not only detect malware at high accuracy, but it can also explain why an application is malicious; in other words, it offers interpretability. to achieve this goal, we leverage the transformer model’s attention mechanism, which learns to put the highest attention on the most suspicious behavior events.
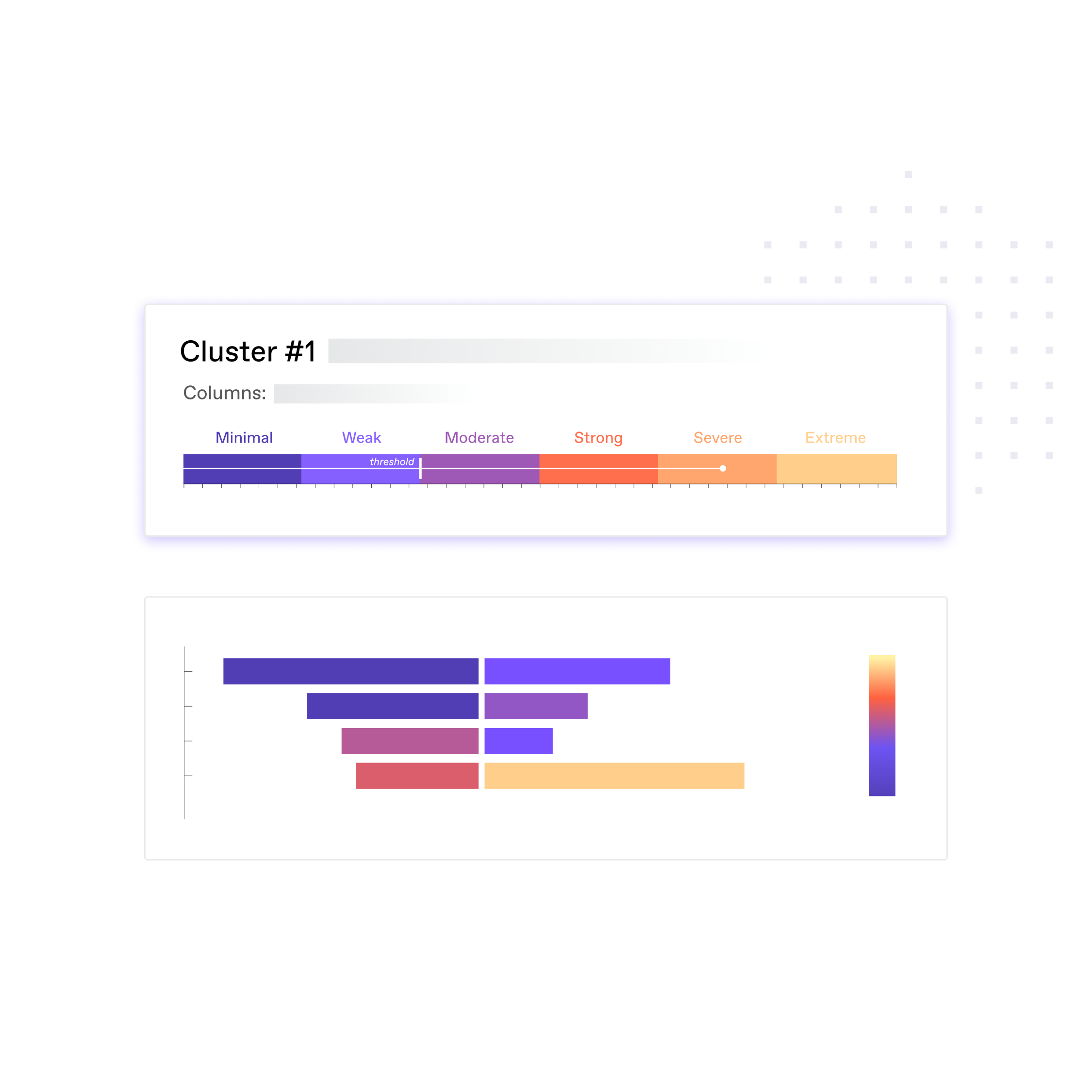
Automated Anomaly Detection Software Anomalo