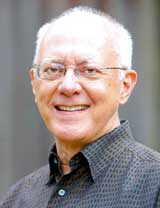
Kimball Vs Inmon In Data Warehouse Architecture For designing, there are two most common architectures named kimball and inmon but the question is which one is better, and which one serves users at low redundancy. let us compare both on some factors. 1. kimball: kimball’s approach to designing a dataware house was introduced by ralph kimball. this approach starts with recognizing the. Kimball and inmon are two methodologies for designing data warehouse architectures. kimball focuses on a bottom up approach with dimensional modeling, while inmon advocates a top down approach with normalized modeling. kimball approach, developed by ralph kimball, emphasizes the dimensional modeling technique.

Differences Between Kimball And Inmon Data Warehouse Architectures By While the kimball approach prioritizes simplicity, flexibility, and user accessibility, the inmon methodology emphasizes data integrity, consistency, and scalability. when deciding between kimball and inmon, consider factors such as your organization’s data architecture, business goals, resource constraints, and long term scalability needs. The two main approaches to designing the data warehouse architecture are the inmon approach vs the kimball approach. in this article, we will dive deeper into what each author proposed, showcase the relative tradeoffs of each approach, and finally contrast and compare the two approaches to make your data architect’s life easier. Difference between kimball vs inmon. the following article provides an outline of kimball vs inmon. there are different ways in which we can align different components of a data warehouse, and these components are an essential part of a data warehouse. for example, the data source helps us identify where the data is coming from. Kimball and inmon are the data architectures that are used to make a data warehouse. ralph kimball introduced the kimball architecture which is easy to use. bottom up implementation is followed by kimball and individual business areas are focused. bill inmon developed the inmon model which supports bottom up implementation.

Differences Between Kimball And Inmon Data Warehouse Architectures By Difference between kimball vs inmon. the following article provides an outline of kimball vs inmon. there are different ways in which we can align different components of a data warehouse, and these components are an essential part of a data warehouse. for example, the data source helps us identify where the data is coming from. Kimball and inmon are the data architectures that are used to make a data warehouse. ralph kimball introduced the kimball architecture which is easy to use. bottom up implementation is followed by kimball and individual business areas are focused. bill inmon developed the inmon model which supports bottom up implementation. In this exploration, we’ll skip the widely available definitions and jump straight into the heart of the matter: the fundamental distinctions between kimball and inmon methodologies for data. Drawing from the early trivaids best practice architecture (as detailed in data warehouse blueprints business intelligence in der praxis (dani schnider, claus jordan, peter welker, joachim wehner)), i’ve found that leveraging the strengths of both inmon and kimball’s methods can be highly effective. However, there are some differences in the data warehouse architectures of both experts: kimball uses the dimensional model such as star schemas or snowflakes to organize the data in dimensional data warehouse while inmon uses er model in enterprise data warehouse. inmon only uses dimensional model for data marts only while kimball uses it for. The kimball vs. inmon debate is not a contest to declare a winner but an exploration of two distinct approaches to data warehousing, each with its merits and ideal use cases. organizations should view these methodologies not as rivals but as complementary tools that can be combined to create a robust and adaptable data ecosystem.
Kimball Vs Inmon Comparing Data Warehouse Methodologies In this exploration, we’ll skip the widely available definitions and jump straight into the heart of the matter: the fundamental distinctions between kimball and inmon methodologies for data. Drawing from the early trivaids best practice architecture (as detailed in data warehouse blueprints business intelligence in der praxis (dani schnider, claus jordan, peter welker, joachim wehner)), i’ve found that leveraging the strengths of both inmon and kimball’s methods can be highly effective. However, there are some differences in the data warehouse architectures of both experts: kimball uses the dimensional model such as star schemas or snowflakes to organize the data in dimensional data warehouse while inmon uses er model in enterprise data warehouse. inmon only uses dimensional model for data marts only while kimball uses it for. The kimball vs. inmon debate is not a contest to declare a winner but an exploration of two distinct approaches to data warehousing, each with its merits and ideal use cases. organizations should view these methodologies not as rivals but as complementary tools that can be combined to create a robust and adaptable data ecosystem.