Crime Rate Prediction Using Machine Learning And Data Mining Pdf The study provides access to the datasets used for crime prediction by researchers and analyzes prominent approaches applied in machine learning and deep learning algorithms to predict crime, offering insights into different trends and factors related to criminal activities. When applied in the context of law enforcement and intelligence analysis, j48 holds the promise of mollifying crime rates and is considered the most efficient ml algorithm for the prediction of crime data in the related literature.
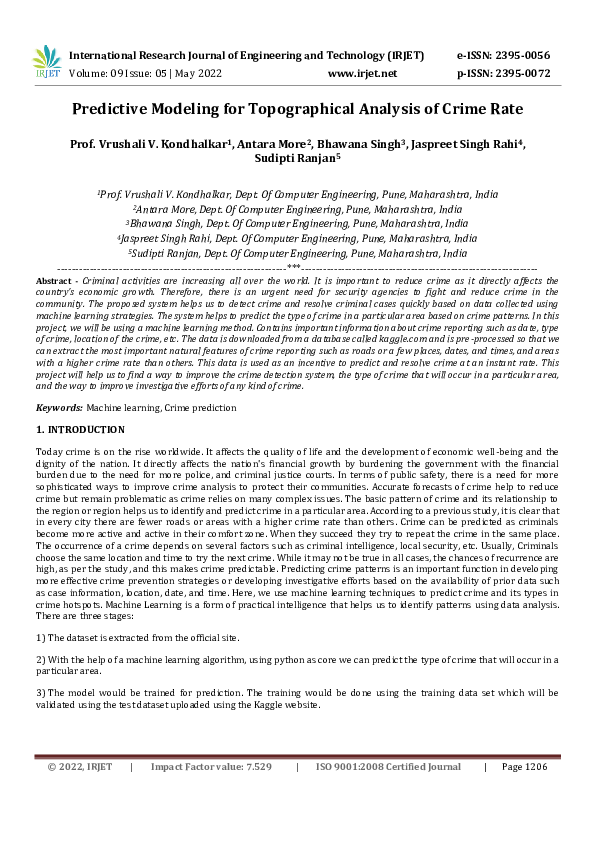
Pdf Predictive Modeling For Topographical Analysis Of Crime Rate Crime rate prediction using machine learning is a critical undertaking in today’s society to handle the ever present concern of criminal activities. this paper presents a comprehensive approach to predicting crime rates by leveraging machine learning algorithms and data analysis techniques. Our review evaluates the models from numerous points of view, including the crime analysis type, crimes studied, prediction technique, performance metrics and evaluations, strengths and weaknesses of the proposed method, and limitations and future directions. Exploratory data analysis predicts more than 35 crime types and suggests a yearly decline in chicago crime rate, and a slight increase in los angeles crime rate; with fewer crimes occurred in february as compared to other months. The findings reveal that the predictive model based on the random forests algorithm delivers the highest accuracy for predicting crime rates, highlighting the potential of machine learning techniques as a valuable tool to aid law enforcement agencies and policymakers in making informed decisions to prevent and reduce crime rates.

Pdf An Innovative Approach To Crime Forecasting Predicting And Exploratory data analysis predicts more than 35 crime types and suggests a yearly decline in chicago crime rate, and a slight increase in los angeles crime rate; with fewer crimes occurred in february as compared to other months. The findings reveal that the predictive model based on the random forests algorithm delivers the highest accuracy for predicting crime rates, highlighting the potential of machine learning techniques as a valuable tool to aid law enforcement agencies and policymakers in making informed decisions to prevent and reduce crime rates. This research proposes a random forest algorithm based machine learning method for crime prediction, leveraging historical crime data to train a predictive model that identifies high crime areas and forecasts future crime incidents. Abstract: this study investigates the application of deep learning techniques and the random forest algorithm in predictive crime analytics, aiming to enhance crime forecasting and prevention strategies. Hfg commissioned three leading criminologists to address this deficit by developing a predictive model of national violent and property crime rates. the researchers identified a small set of demographic, economic, and criminal justice variables whose changes over nearly four decades closely track trends in crime.