Data Lake And Data Warehouse Pdf Data Warehouse Big Data In this paper, we present a comparative review of the existing data warehouse and data lake technology to highlight their strengths and weaknesses and propose the desired and necessary. This paper provides an overview on the evolution of analytical data platforms from data warehouses over data lakes to lakehouses and elaborates on the vision and characteristics of the latter. furthermore, it addresses the.
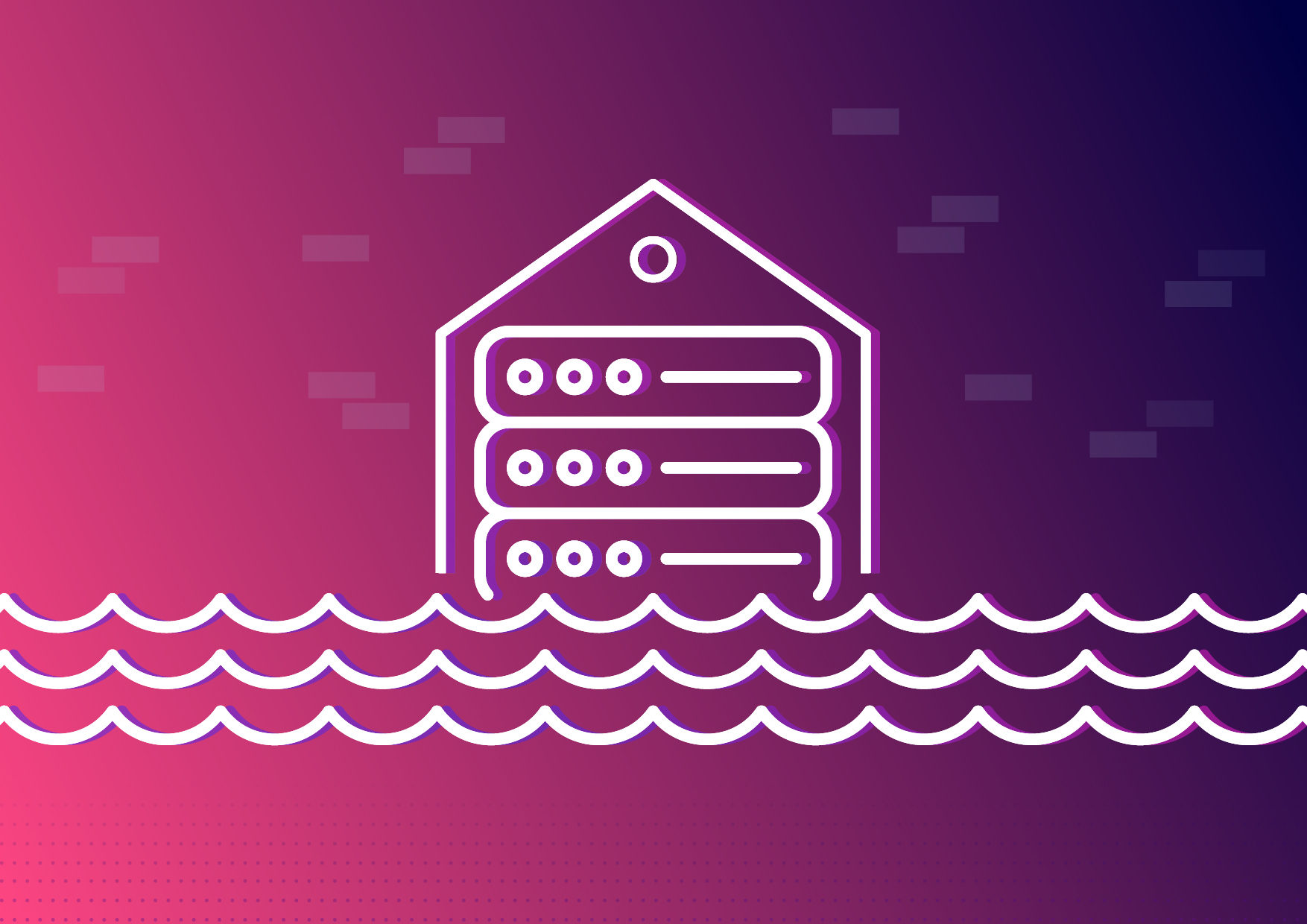
Unlocking Data Analytics Potential With A Data Lakehouse Proskale To address the ‘big data’ problems due to high volume, velocity, variety, and veracity, data management systems evolved from structured databases to big data storage systems, graph databases, data warehouses, and data lakes but each solution has its strengths and shortcomings. This new data architecture is a combination of governed and reliable data warehouses and flexible, scalable and cost effective data lakes. in this paper, we present how traditional approaches of spatial data management in the context of spatial big data have quickly shown their limits. First generation data platforms, known as data warehouses, were built to make integrated transactional data available for specific analytic purposes. within a decade, however, enterprise data had greatly increased in volume and variety, and data warehouses could no longer meet storage and management needs. This topic covers the evolution of data management practices from traditional rdbms to modern big data solutions like hadoop, spark, data lakes, and the emerging lakehouse.
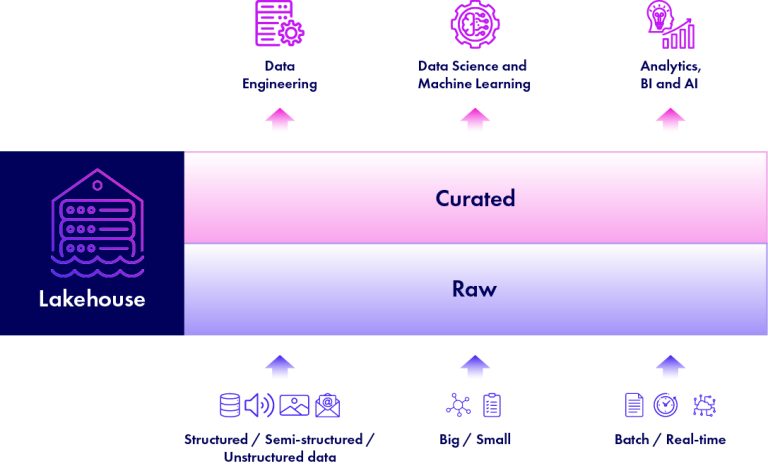
Unlocking Data Analytics Potential With A Data Lakehouse Proskale First generation data platforms, known as data warehouses, were built to make integrated transactional data available for specific analytic purposes. within a decade, however, enterprise data had greatly increased in volume and variety, and data warehouses could no longer meet storage and management needs. This topic covers the evolution of data management practices from traditional rdbms to modern big data solutions like hadoop, spark, data lakes, and the emerging lakehouse. By the end of the 2010s, as we transitioned from data 1.0 to data 2.0, two dominant architectural paradigms had emerged: the cloud data warehouse and the data lake. while powerful, each of these have limitations (both in terms of capabilities as well as user experience). In 2020, databrick introduced a significant paper on data lakehouse as a novel approach to data management. this approach combines both data warehouse and data lake into a single system, leveraging the cost effectiveness of cloud storage services while operating in a more ‘warehouse’ manner. In the end, the roi of a data warehouse versus a lakehouse depends on your organization's unique data needs, analytical goals, and growth plans. by aligning your data strategy with your business objectives—and maybe getting some insights from statsig—you can make a decision that maximizes the value of your data investments. Our journey through the history of data lakehouses will shed light on how these technologies have evolved. by understanding their origins and the needs they address, we’ll better understand why.
Proskale On Linkedin From Data Warehouse To Data Lakehouse The By the end of the 2010s, as we transitioned from data 1.0 to data 2.0, two dominant architectural paradigms had emerged: the cloud data warehouse and the data lake. while powerful, each of these have limitations (both in terms of capabilities as well as user experience). In 2020, databrick introduced a significant paper on data lakehouse as a novel approach to data management. this approach combines both data warehouse and data lake into a single system, leveraging the cost effectiveness of cloud storage services while operating in a more ‘warehouse’ manner. In the end, the roi of a data warehouse versus a lakehouse depends on your organization's unique data needs, analytical goals, and growth plans. by aligning your data strategy with your business objectives—and maybe getting some insights from statsig—you can make a decision that maximizes the value of your data investments. Our journey through the history of data lakehouses will shed light on how these technologies have evolved. by understanding their origins and the needs they address, we’ll better understand why.