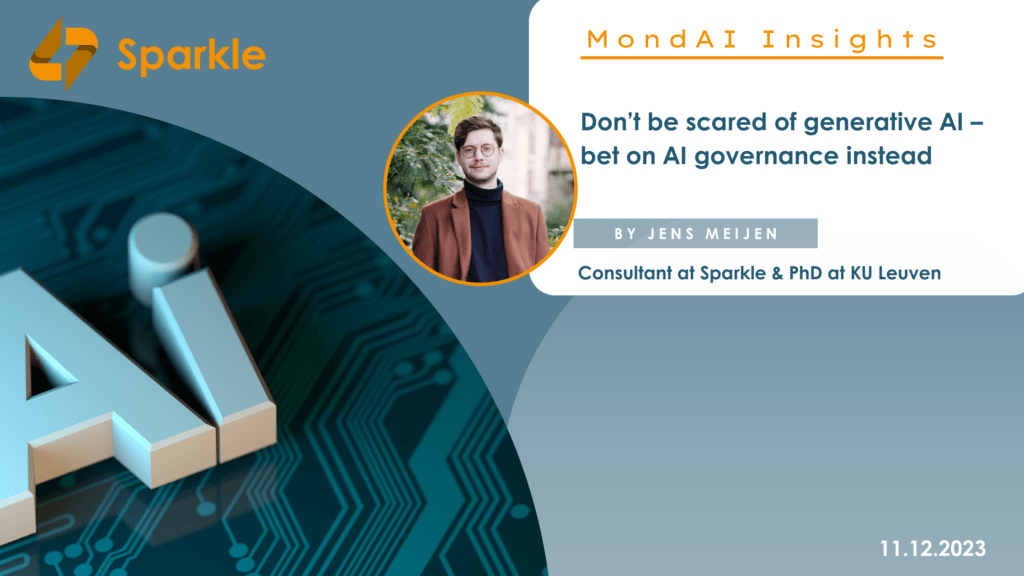
Mastering Generative Ai Governance Sparkle Data Consulting Looking for a career in data? over time, sparkle gradually evolved to 5 domains of expertise within the field of data, being: data platform modernization, data analytics, data governance, artificial intelligence & low code & automation. find out more about these domains by clicking on the circles. From gatekeeper to enabler: instead of simply restricting access and enforcing rules, data governance must evolve to empower innovation. this means ensuring data quality, transparency, and ethical use, fostering trust in ai generated outputs. data governance should be all about enabling creativity whilst minimising risk.

Data Governance And Generative Ai Dan Galavan Sparkle comprehends the nuanced challenges presented by the eu ai act, providing a unique blend of profound expertise in ai technology, governance, and compliance. we assist you in mitigating various ai risks, including those that may result in regulatory fines, as well as risks that these guidelines aim to prevent—such as potential harm to. Data governance is critical to enabling organizations to build enterprise generative ai applications. as enterprise use cases continue to evolve, there will be a need to expand the data infrastructure to govern and manage new, diverse, unstructured datasets to ensure alignment with privacy, security, and quality policies. This article, based on the analysis of privacy and personal data risk governance of generative ai from the perspective of china, proposes suggestions for enhancing privacy and personal data protection regulation in the era of generative ai. Consider the data management implications: there are five key areas to consider for using unstructured data management in ai tools, spanning security, privacy, lineage, ownership, and governance of unstructured data, or splog. consideration begins by gaining thorough visibility into file and object data across on premises, edge, and cloud storage.
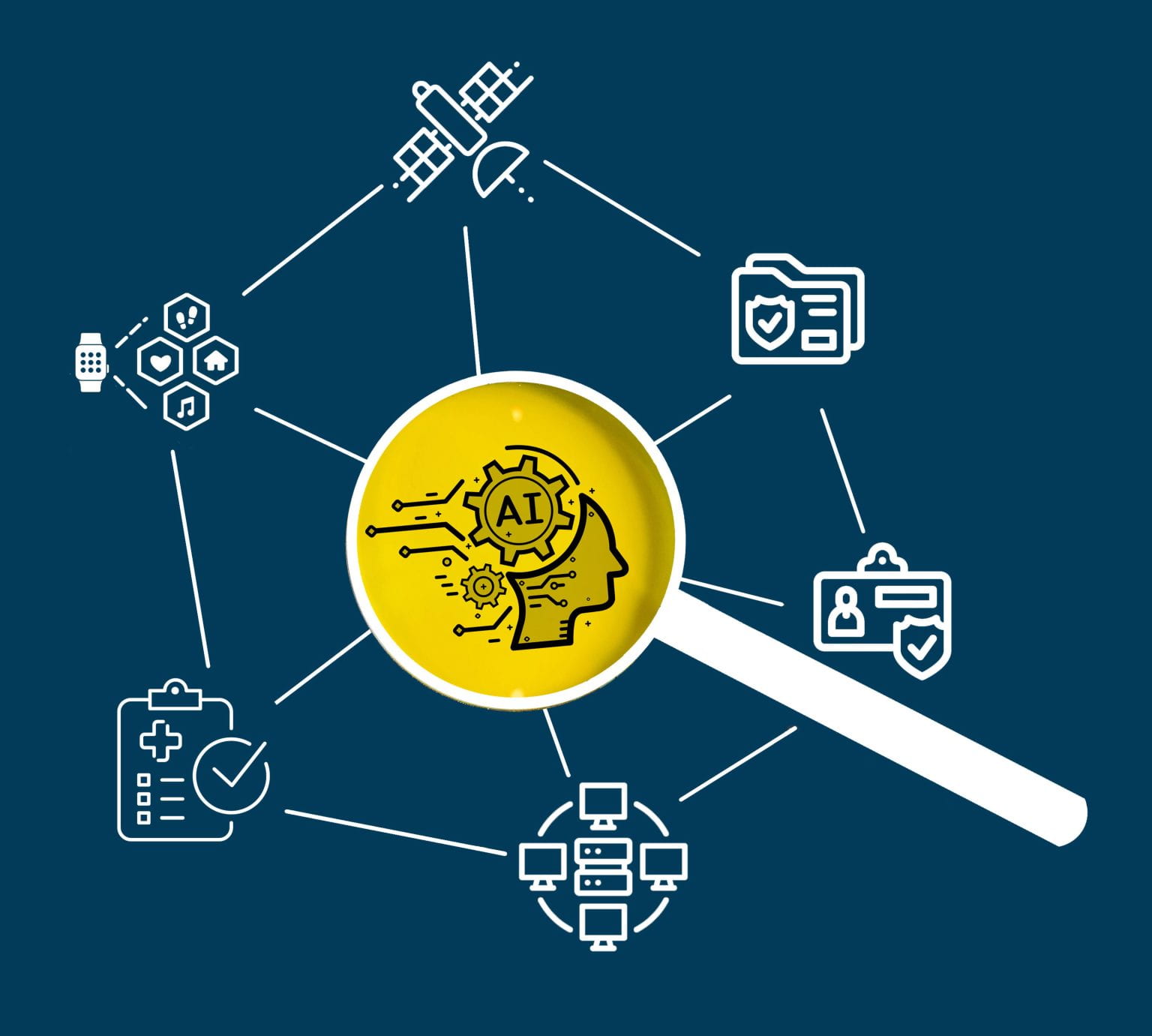
Conference Data Governance In The Age Of Generative Ai Media This article, based on the analysis of privacy and personal data risk governance of generative ai from the perspective of china, proposes suggestions for enhancing privacy and personal data protection regulation in the era of generative ai. Consider the data management implications: there are five key areas to consider for using unstructured data management in ai tools, spanning security, privacy, lineage, ownership, and governance of unstructured data, or splog. consideration begins by gaining thorough visibility into file and object data across on premises, edge, and cloud storage. Below are three near term considerations for making decisions responsibly regarding genai, preparing your organization for genai, and collaborating with operational functions to drive an organization’s genai ambition—whether that affects the individual (the worker), the functional (the team or capability), or the enterprise (the organization) le. The report offers a three step process that’s broadly applicable across industries: create a data moat—map out the data and its workflows and determine the costs needed to develop the system. With generative ai (genai) now capable of producing text, code, images, and videos, organizations must adopt robust governance frameworks to balance innovation, responsibility, and compliance. most companies can build on existing governance frameworks like risk management, it prioritization, and vendor assessments. Skip to content. challenges; solutions; careers; insights; about us; contact us.
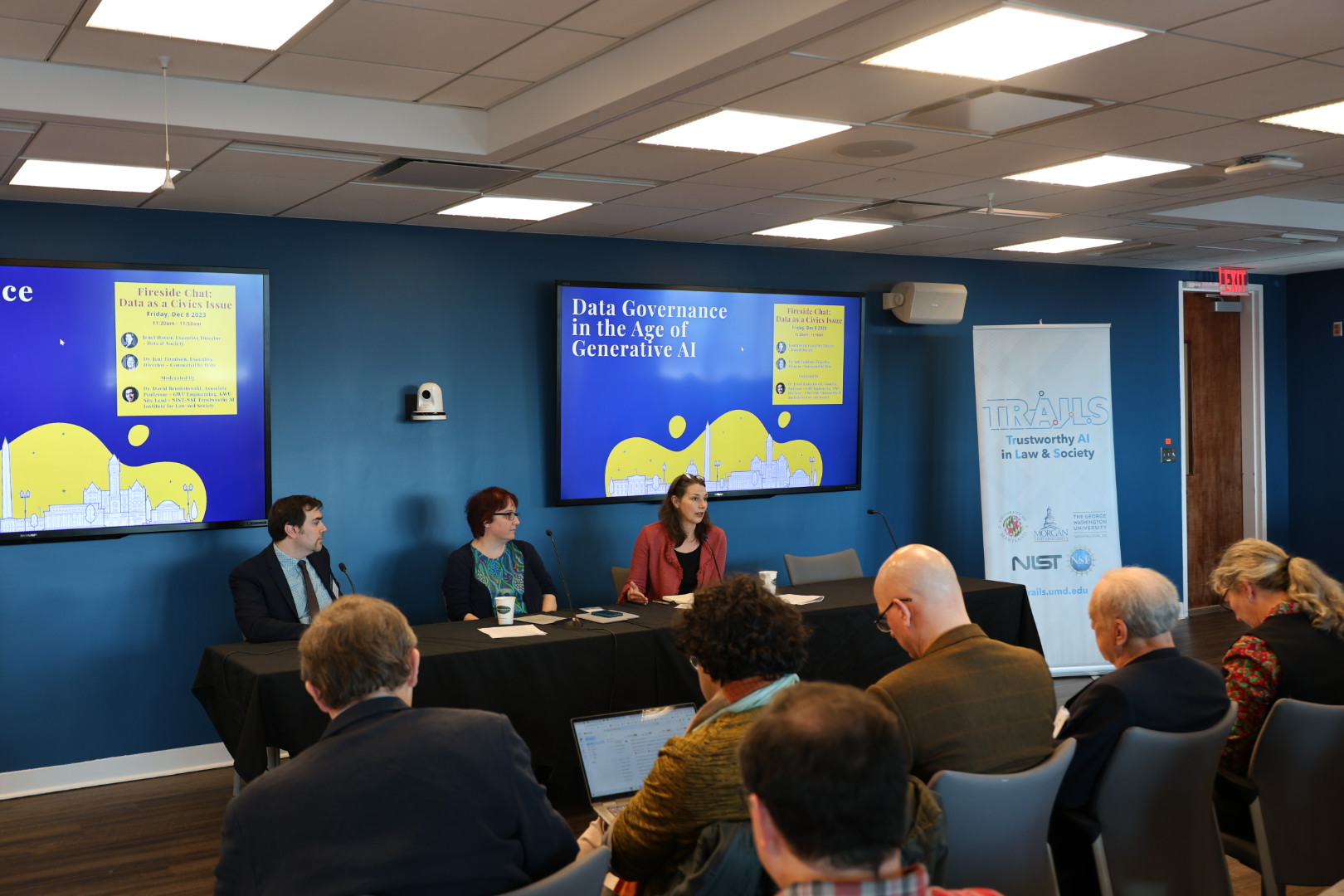
Addressing Gaps In Data Governance To Promote Trust In Generative Ai Below are three near term considerations for making decisions responsibly regarding genai, preparing your organization for genai, and collaborating with operational functions to drive an organization’s genai ambition—whether that affects the individual (the worker), the functional (the team or capability), or the enterprise (the organization) le. The report offers a three step process that’s broadly applicable across industries: create a data moat—map out the data and its workflows and determine the costs needed to develop the system. With generative ai (genai) now capable of producing text, code, images, and videos, organizations must adopt robust governance frameworks to balance innovation, responsibility, and compliance. most companies can build on existing governance frameworks like risk management, it prioritization, and vendor assessments. Skip to content. challenges; solutions; careers; insights; about us; contact us.
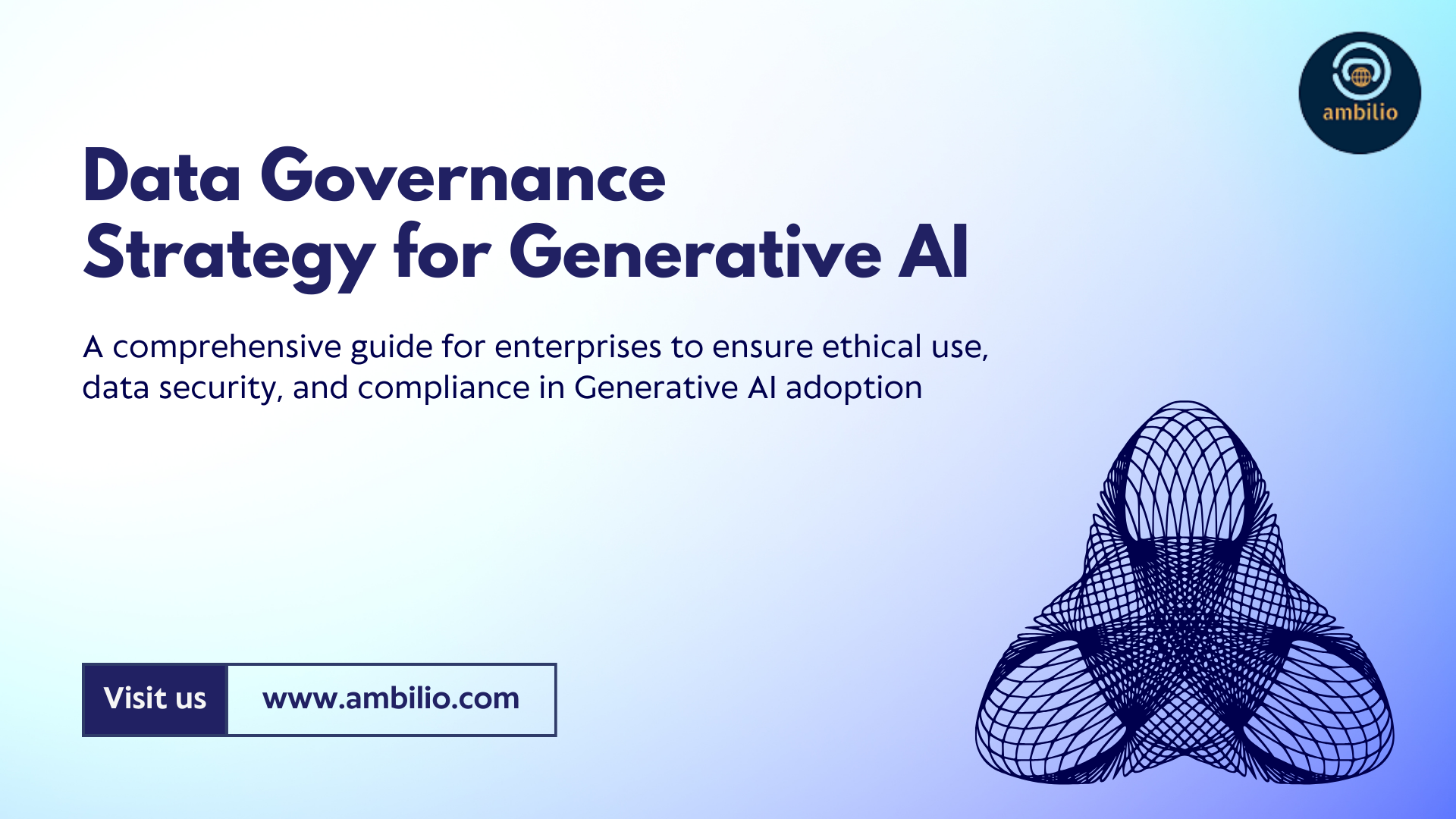
Data Governance Strategy For Generative Ai Adoption With generative ai (genai) now capable of producing text, code, images, and videos, organizations must adopt robust governance frameworks to balance innovation, responsibility, and compliance. most companies can build on existing governance frameworks like risk management, it prioritization, and vendor assessments. Skip to content. challenges; solutions; careers; insights; about us; contact us.