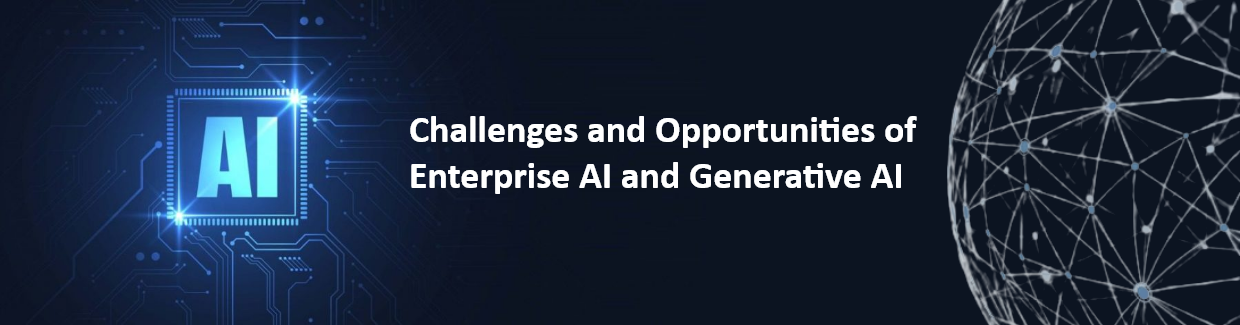
Enterprise Ai And Generative Ai Challenges Opportunities So, how can industry leaders navigate the world of enterprise ai without losing their footing and potentially harming their organizations? the answer lies in combining traditional business processes and quality management with cutting edge auxiliary technologies to mitigate the risks surrounding ai and its outputs. To help organizations navigate these challenges, microsoft has released the microsoft guide for securing the ai powered enterprise issue 1: getting started with ai applications—the first in a series of deep dives into ai security, compliance, and governance. this guide lays the groundwork for securing the ai tools teams are already exploring and provides guidance on how to manage the risks.
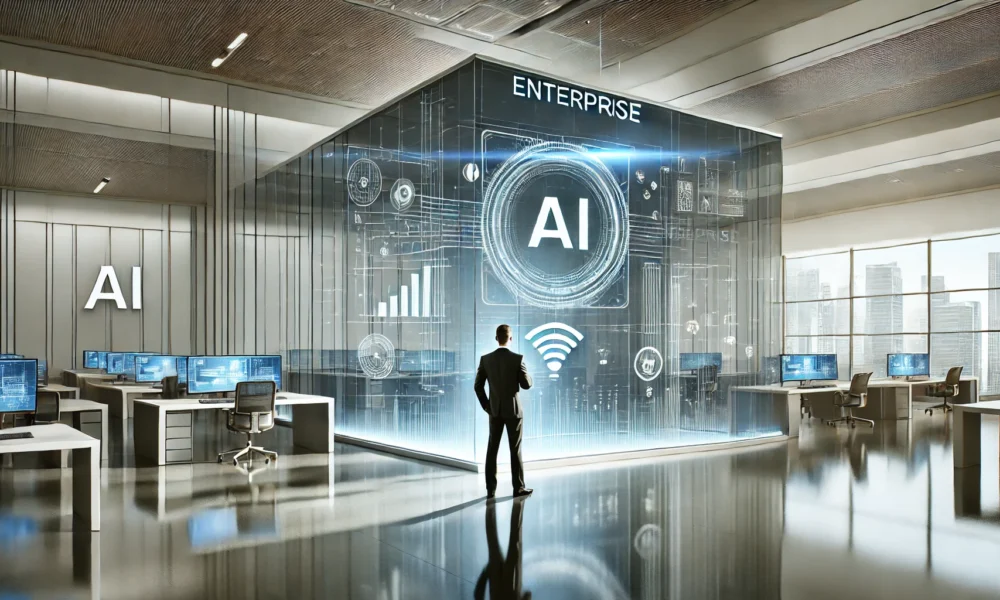
Navigating The 2025 Challenges Of Adopting Enterprise Ai Bard Ai Investment is increasing, but the clock is ticking to create value. the q3 edition of the state of generative ai in the enterprise, moving from potential to performance, reveals how organizations are navigating challenges and measuring value, focusing on two areas critical to scaling: data and governance, risk and compliance. view q3 report. Developing, training, and deploying ai solutions requires significant financial commitment in terms of infrastructure, software, and skilled talent. many enterprises face challenges in balancing this cost with measurable returns on investment (roi). identifying the right use cases for ai implementation is vital. Five key strategies for ai driven success. 1. develop a comprehensive ai agenda. aligning ai capabilities with business needs is critical. whether in automation, customer service, content generation, predictive analytics, or cybersecurity, a structured approach is essential. Enterprises must not only adopt the right technical solutions but also navigate cultural, operational, and ethical hurdles. in this article, we’ll break down the primary challenges organizations face when adopting ai ml and strategies to address them. 1. data quality and availability.
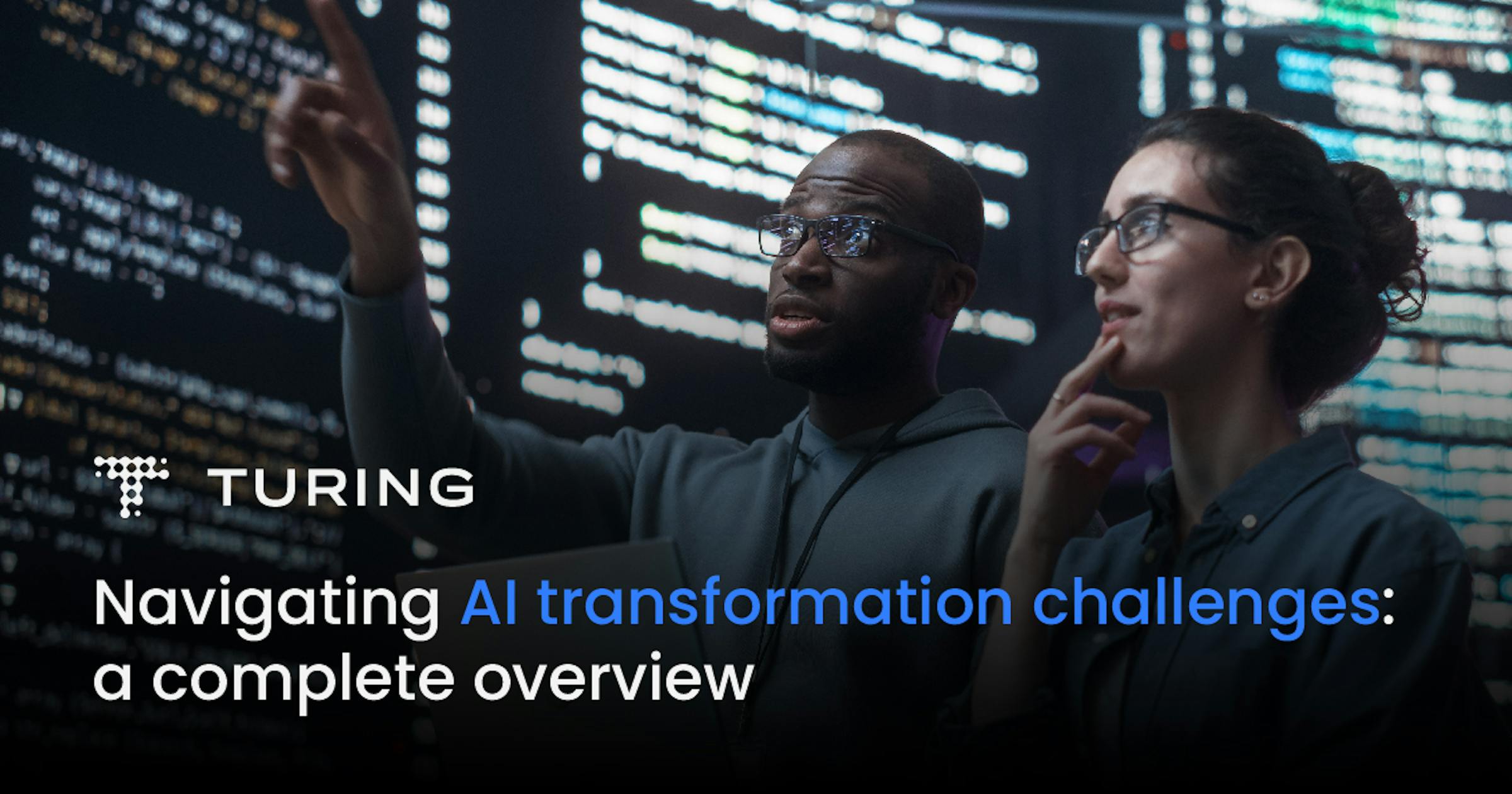
How To Navigate Top 5 Challenges In Ai Transformation Five key strategies for ai driven success. 1. develop a comprehensive ai agenda. aligning ai capabilities with business needs is critical. whether in automation, customer service, content generation, predictive analytics, or cybersecurity, a structured approach is essential. Enterprises must not only adopt the right technical solutions but also navigate cultural, operational, and ethical hurdles. in this article, we’ll break down the primary challenges organizations face when adopting ai ml and strategies to address them. 1. data quality and availability. The success of ai adoption hinges on how an enterprise navigates these challenges and presents strategies to surmount them, ensuring a seamless transition into the ai driven future. Key challenges in enterprise ai adoption – data privacy & security – ensuring compliance with regulations (gdpr, ccpa) and protecting sensitive business data from ai training models. – model reliability & bias – generative ai models can produce inaccurate or biased outputs, impacting trust and usability. Here's a roadmap to help modern enterprises chart a successful course through the ai landscape: before diving into the technical intricacies of ai, it's crucial to define clear business objectives. what specific challenges are you trying to solve? how can ai help you achieve your strategic goals?. Enterprise ai is the focused application of artificial intelligence to improve efficiency, enhance performance, and drive innovation across teams. in the backdrop of general purpose ai’s large scale models, enterprise ai is increasingly pulling ahead in favor of hyper focused, task specific models (tsms).
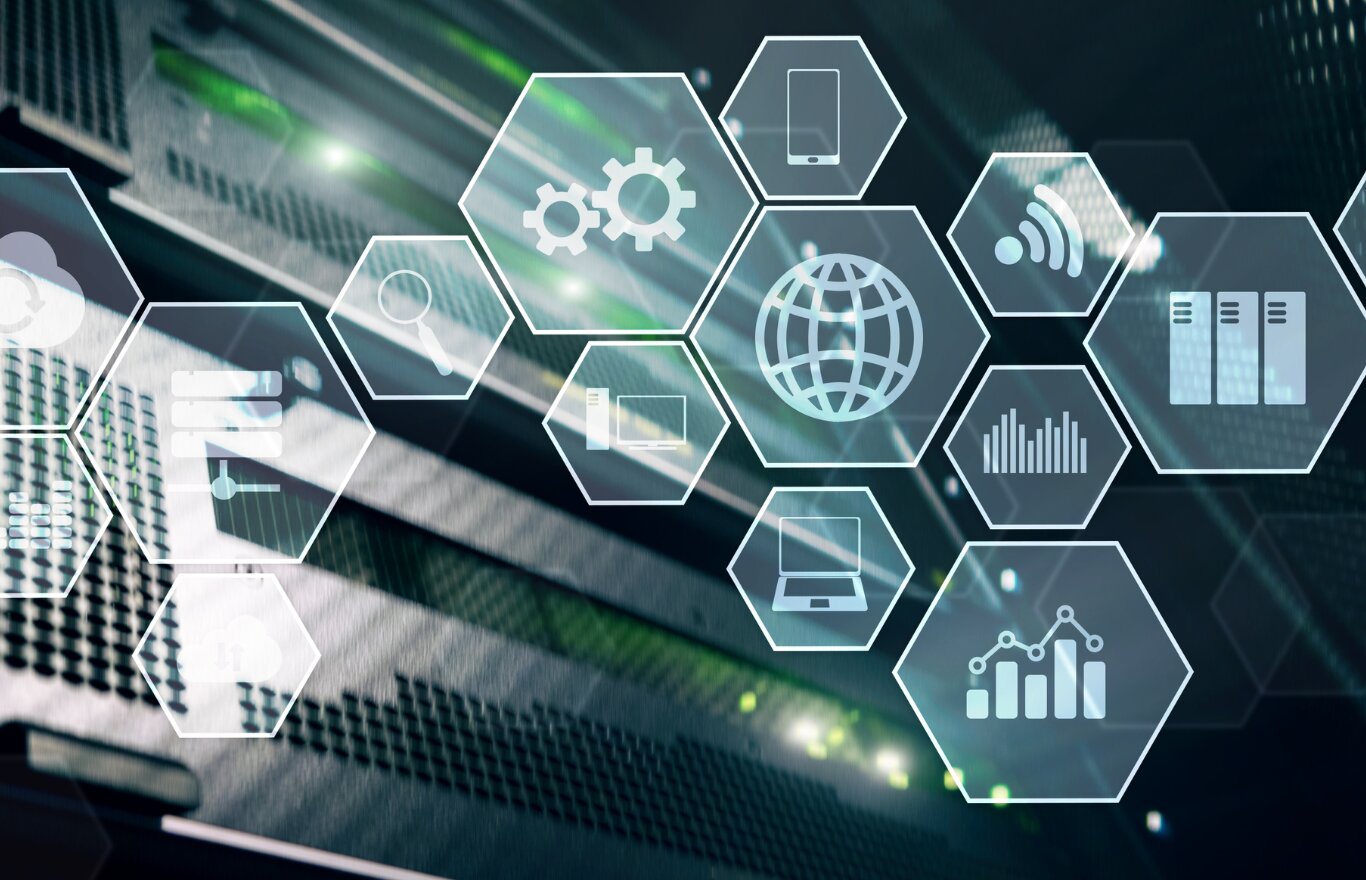
Enterprise Ai The success of ai adoption hinges on how an enterprise navigates these challenges and presents strategies to surmount them, ensuring a seamless transition into the ai driven future. Key challenges in enterprise ai adoption – data privacy & security – ensuring compliance with regulations (gdpr, ccpa) and protecting sensitive business data from ai training models. – model reliability & bias – generative ai models can produce inaccurate or biased outputs, impacting trust and usability. Here's a roadmap to help modern enterprises chart a successful course through the ai landscape: before diving into the technical intricacies of ai, it's crucial to define clear business objectives. what specific challenges are you trying to solve? how can ai help you achieve your strategic goals?. Enterprise ai is the focused application of artificial intelligence to improve efficiency, enhance performance, and drive innovation across teams. in the backdrop of general purpose ai’s large scale models, enterprise ai is increasingly pulling ahead in favor of hyper focused, task specific models (tsms).

Elevate Excellence Navigating Ai Startup Challenges Here's a roadmap to help modern enterprises chart a successful course through the ai landscape: before diving into the technical intricacies of ai, it's crucial to define clear business objectives. what specific challenges are you trying to solve? how can ai help you achieve your strategic goals?. Enterprise ai is the focused application of artificial intelligence to improve efficiency, enhance performance, and drive innovation across teams. in the backdrop of general purpose ai’s large scale models, enterprise ai is increasingly pulling ahead in favor of hyper focused, task specific models (tsms).
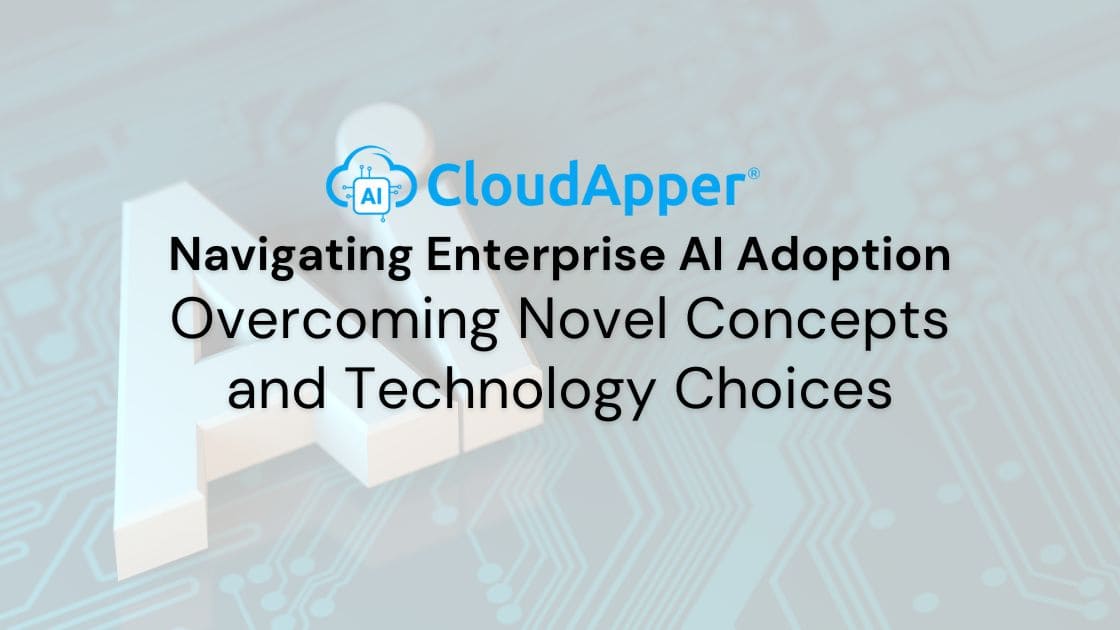
Navigating Enterprise Ai Adoption