Github Jofam Covid19trendanalysis Naive Trend Analysis For Covid 19 The research aims to use gis software to analyze covid 19's spatial trend distribution and temporal pattern analysis for human health. Monitoring, evaluating, and planning using geospatial analysis are essential for controlling the spread of covid 19 within the country. a new outbreak of the recent so called coronavirus.
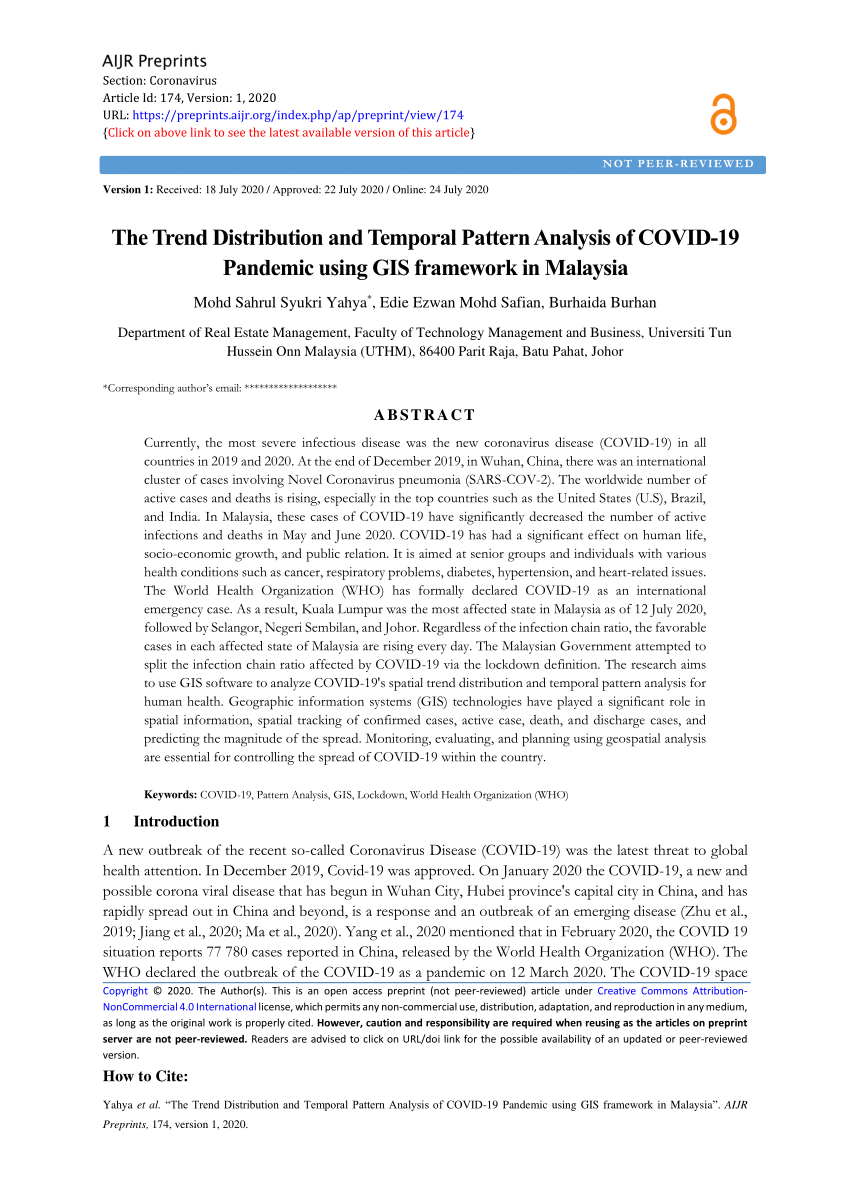
Pdf The Trend Distribution And Temporal Pattern Analysis Of Covid 19 This study seeks to examine and analyze the spatial and temporal patterns of covid‐19 outbreaks and identify the spatiotemporal distribution characteristics and changing trends of cases. The research aims to use gis software to analyze covid 19's spatial trend distribution and temporal pattern analysis for human health and finds that kuala lumpur was the most affected state as of 12 july 2020, followed by selangor, negeri sembilan, and johor. Draw a distribution map of the cumulative number of covid‐19 by inverse distance weighted interpolation; analyze the spatio‐temporal characteristics of the daily number of covid‐19 in. We included studies that incorporated a geospatial method to observe the spatial risk of covid 19 and studies that performed a spatial regression analysis to understand the association between covid 19 and socioeconomic, demographic, or climatic covariates.

Pdf Spatial And Temporal Analysis Of Covid 19 Cases Distribution In Draw a distribution map of the cumulative number of covid‐19 by inverse distance weighted interpolation; analyze the spatio‐temporal characteristics of the daily number of covid‐19 in. We included studies that incorporated a geospatial method to observe the spatial risk of covid 19 and studies that performed a spatial regression analysis to understand the association between covid 19 and socioeconomic, demographic, or climatic covariates. In this paper, three geographical models in arcgis 10.8.1 were utilized to analyze and evaluate covid 19, including geographic weight regression (gwr), ordinary least squares (ols), and spatial autocorrelation (moran i). In this paper, we study the trend pattern of the covid 19 series using an autoregressive model with a trend approximated by a linear spline function. iden tification of the number of knots and their locations are obtained using bayes factor and posterior probability, respectively. This paper shows the spatial distribution of the cumulative confirmed cases of covid 19, and it can be seen that the areas with more cases have an obvious continuous distribution trend, with hubei province as the center for diffusion, while the areas with fewer cases have a relatively stable coverage. This city level modeling study analyzed and visualized the spatio temporal distribution characteristics of covid 19 in mainland china from perspectives of time, space, and space–time, respectively, to explore the high risk hot spots and systematically discuss the space–time aggregation pattern of covid 19 at the city level across the.

Temporal Trend Of Daily Confirmed Covid 19 In Malaysia Part A To F In this paper, three geographical models in arcgis 10.8.1 were utilized to analyze and evaluate covid 19, including geographic weight regression (gwr), ordinary least squares (ols), and spatial autocorrelation (moran i). In this paper, we study the trend pattern of the covid 19 series using an autoregressive model with a trend approximated by a linear spline function. iden tification of the number of knots and their locations are obtained using bayes factor and posterior probability, respectively. This paper shows the spatial distribution of the cumulative confirmed cases of covid 19, and it can be seen that the areas with more cases have an obvious continuous distribution trend, with hubei province as the center for diffusion, while the areas with fewer cases have a relatively stable coverage. This city level modeling study analyzed and visualized the spatio temporal distribution characteristics of covid 19 in mainland china from perspectives of time, space, and space–time, respectively, to explore the high risk hot spots and systematically discuss the space–time aggregation pattern of covid 19 at the city level across the.