Rag Vs Fine Tuning Ai Digitalnews Rag enhances responses by retrieving relevant external data without changing the model itself, whereas fine tuning adjusts the model’s internal parameters for more customization. combining the two methods can often yield the best outcomes by striking a balance between efficiency, accuracy, and adaptability. Leveraging the full potential of llms requires choosing the right technique between retrieval augmented generation (rag) and fine tuning. let’s examines when to use rag versus fine tuning for.
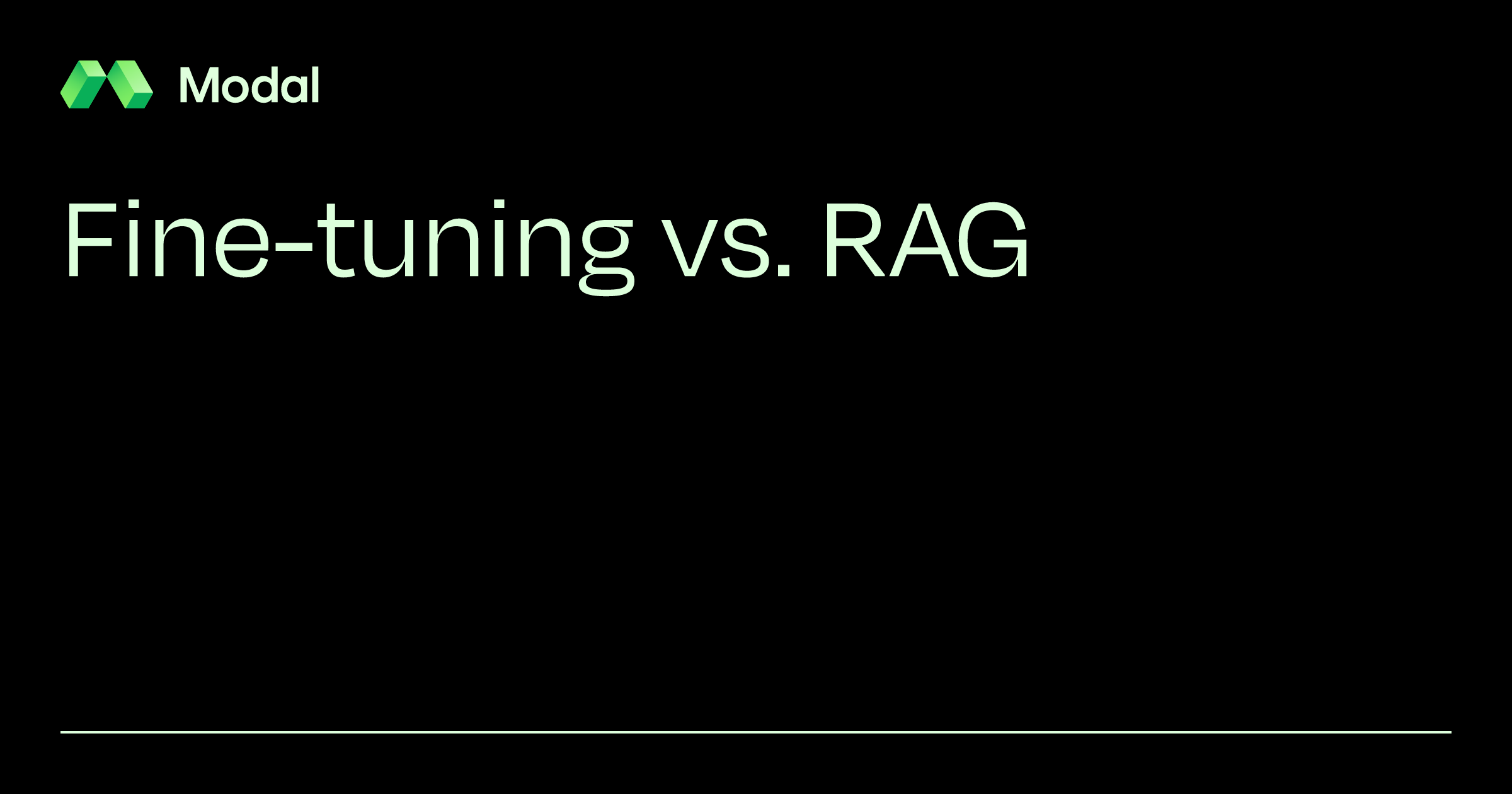
Fine Tuning Vs Rag Modal Blog How to choose between rag vs fine tuning. you should choose between rag vs fine tuning based on your use case and available resources. while rag is the preferred option for most use cases, that doesn’t mean that rag and fine tuning are mutually exclusive either. How to choose between rag vs fine tuning vs raft it all depends on your use case and available resources. if you are a startup with limited resources, then try building a rag proof of concept using open ai api and langchain framework. Rag: best for real time, dynamic tasks requiring frequent updates from external sources. fine tuning: ideal for static, high precision tasks that require deep domain expertise. performance: fine tuning offers faster inference at the cost of memory, while rag introduces latency due to retrieval steps. Fine tuning involves adapting a pre trained model to a particular field or problem by training it further on a targeted dataset. in this context, a “domain” refers to a specific area of application, such as healthcare, legal analysis, or financial forecasting.
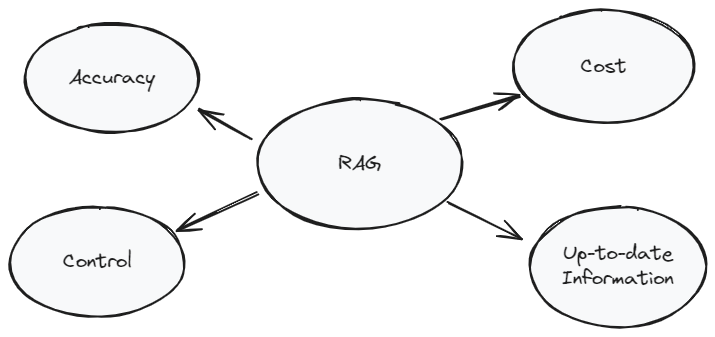
Prompt Engineering Vs Fine Tuning Vs Rag Rag: best for real time, dynamic tasks requiring frequent updates from external sources. fine tuning: ideal for static, high precision tasks that require deep domain expertise. performance: fine tuning offers faster inference at the cost of memory, while rag introduces latency due to retrieval steps. Fine tuning involves adapting a pre trained model to a particular field or problem by training it further on a targeted dataset. in this context, a “domain” refers to a specific area of application, such as healthcare, legal analysis, or financial forecasting. In the rapidly evolving field of artificial intelligence, two methods stand out for refining machine learning models: retrieval augmented generation (rag) and fine tuning. both approaches play a critical role in maximizing the efficiency of large language models (llms) and are essential for developing domain specific solutions. Both rag and fine tuning make generic ai models more useful in a particular field or for a specific use case. rag gives an llm access to a company’s internal data stores; that helps the llm provide more targeted responses, which is critical for use cases that rely on up to date information. When setting up a language model for specific tasks, two key approaches often come into play: retrieval augmented generation (rag) and fine tuning. each method brings distinct advantages, and choosing the right one is crucial for the model’s performance in targeted environments. in this article, we'll explore both rag and fine tuning in detail. However, advanced methods like retrieval augmented generation (rag) or fine tuning can help you build context aware apps that are easily steerable and more reliable once in production. this article compares rag and fine tuning to help you determine which approach—or possibly both—is best for your project.
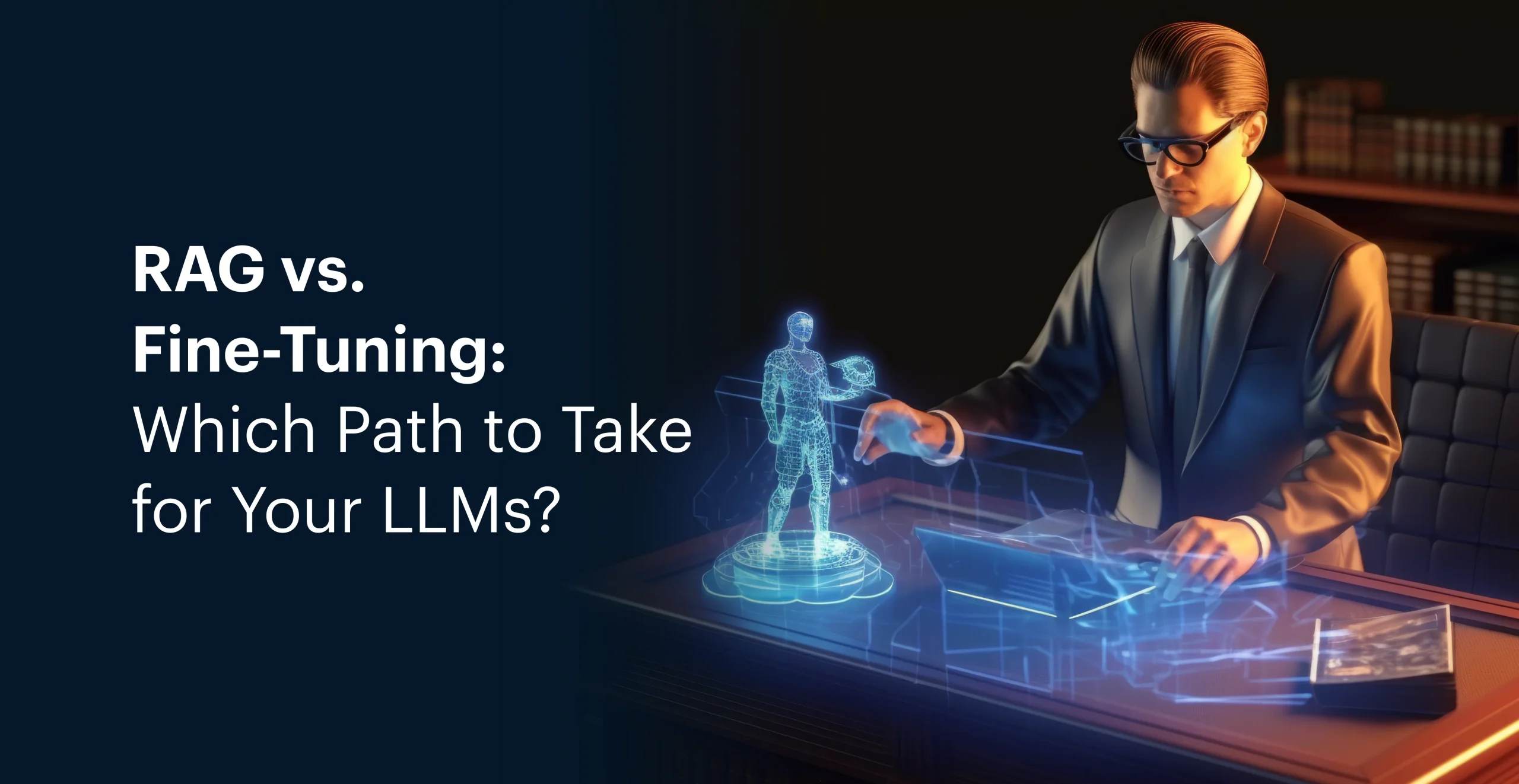
Rag Vs Fine Tuning Which Ai Model Approach Is Best Openxcell In the rapidly evolving field of artificial intelligence, two methods stand out for refining machine learning models: retrieval augmented generation (rag) and fine tuning. both approaches play a critical role in maximizing the efficiency of large language models (llms) and are essential for developing domain specific solutions. Both rag and fine tuning make generic ai models more useful in a particular field or for a specific use case. rag gives an llm access to a company’s internal data stores; that helps the llm provide more targeted responses, which is critical for use cases that rely on up to date information. When setting up a language model for specific tasks, two key approaches often come into play: retrieval augmented generation (rag) and fine tuning. each method brings distinct advantages, and choosing the right one is crucial for the model’s performance in targeted environments. in this article, we'll explore both rag and fine tuning in detail. However, advanced methods like retrieval augmented generation (rag) or fine tuning can help you build context aware apps that are easily steerable and more reliable once in production. this article compares rag and fine tuning to help you determine which approach—or possibly both—is best for your project.