
Fine Tuning Large Language Models Llms Enkefalos Your Partner For Raft, which stands for retrieval augmented fine tuning, is a novel approach developed by a research team at uc berkeley to enhance the performance of large language models (llms) in domain specific tasks. this method combines the strengths of retrieval augmented generation (rag) and fine tuning to create a more effective training strategy for llms. The difference between rag and fine tuning is that rag augments a natural language processing (nlp) model by connecting it to an organization’s proprietary database, while fine tuning optimizes deep learning models for domain specific tasks. rag and fine tuning have the same intended outcome: enhancing a model’s performance to maximize.

How To Fine Tune Large Language Models Llms With Kili Technology When adapting large language models (llms) for the enterprise, there are typically two primary strategies to choose from: fine tuning and retrieval augmented generation (rag). while fine tuning focuses on shaping the model's responses and behavior, rag relies on integrating external data into the model's workflow. If the application requires real time or near real time responses, consider the latency introduced by each method. rag systems, which involve retrieving data before generating a response, might introduce more latency compared to a finetuned llm that generates responses based on internalised knowledge. maintenance and support. think about the. Large language models (llms) have revolutionized natural language processing, but their effectiveness can be further enhanced through specialized techniques. this article explores two such methods: retrieval augmented generation (rag) and fine tuning. by understanding these approaches, data scientists and ai practitioners can make informed. In this tutorial, we will explore rag and fine tuning, two distinct techniques used to improve llm responses. we will examine their differences and put theory into practice by evaluating results. additionally, we will dive into hybrid techniques that combine fine tuned models with rag systems to leverage the best of both worlds.
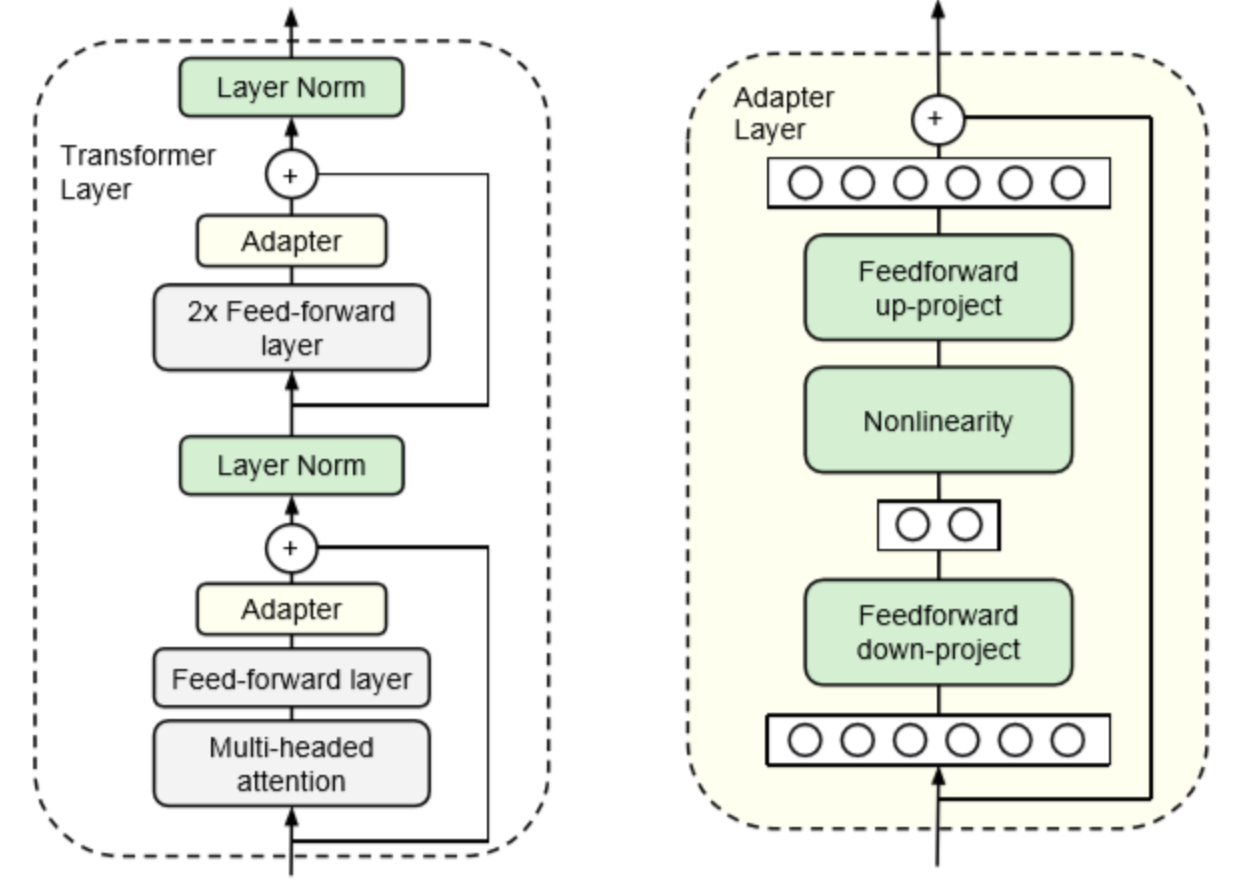
Finetuning Large Language Models Llms Large language models (llms) have revolutionized natural language processing, but their effectiveness can be further enhanced through specialized techniques. this article explores two such methods: retrieval augmented generation (rag) and fine tuning. by understanding these approaches, data scientists and ai practitioners can make informed. In this tutorial, we will explore rag and fine tuning, two distinct techniques used to improve llm responses. we will examine their differences and put theory into practice by evaluating results. additionally, we will dive into hybrid techniques that combine fine tuned models with rag systems to leverage the best of both worlds. When it comes to enhancing the capabilities of large language models (llms), two powerful techniques stand out: rag (retrieval augmented generation) and fine tuning. both methods have their strengths and are suited for different use cases, but choosing the right approach depends on your specific needs. Retrieval augmented generation (rag) and fine tuning are two effective techniques that enterprises can leverage to enhance the performance of large language models (llms). both approaches are designed to tailor llms for specific applications, yet the underlying methodologies behind each are quite distinct. There are two methods businesses can use to unlock the full potential of large language models (llms). they are the retrieval augmented generation (rag) and fine tuning. both these methods operate by customizing the llm for specific use cases. however, the methodologies that these two follow are completely different. # exploring fine tuning in llms. fine tuning is a pivotal technique in optimizing large language models (fine tuning). this method involves tailoring pre trained models to specific tasks or domains, enhancing their performance and adaptability. there are various approaches to fine tuning that cater to different needs and objectives.
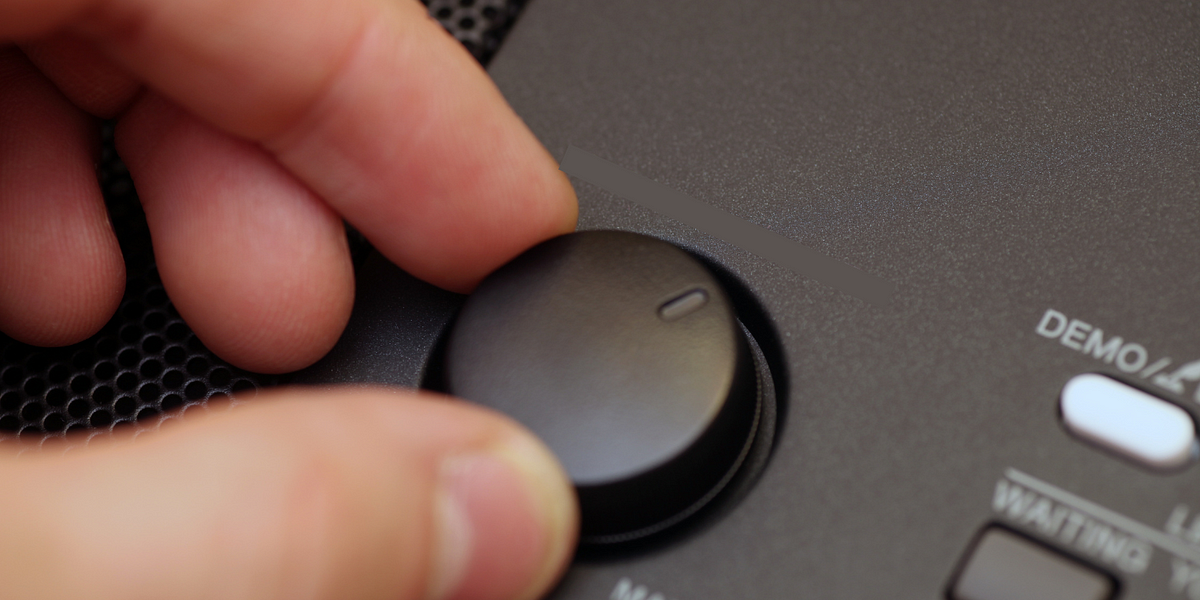
Fine Tuning Large Language Models Llms By Shaw Talebi Towards When it comes to enhancing the capabilities of large language models (llms), two powerful techniques stand out: rag (retrieval augmented generation) and fine tuning. both methods have their strengths and are suited for different use cases, but choosing the right approach depends on your specific needs. Retrieval augmented generation (rag) and fine tuning are two effective techniques that enterprises can leverage to enhance the performance of large language models (llms). both approaches are designed to tailor llms for specific applications, yet the underlying methodologies behind each are quite distinct. There are two methods businesses can use to unlock the full potential of large language models (llms). they are the retrieval augmented generation (rag) and fine tuning. both these methods operate by customizing the llm for specific use cases. however, the methodologies that these two follow are completely different. # exploring fine tuning in llms. fine tuning is a pivotal technique in optimizing large language models (fine tuning). this method involves tailoring pre trained models to specific tasks or domains, enhancing their performance and adaptability. there are various approaches to fine tuning that cater to different needs and objectives.
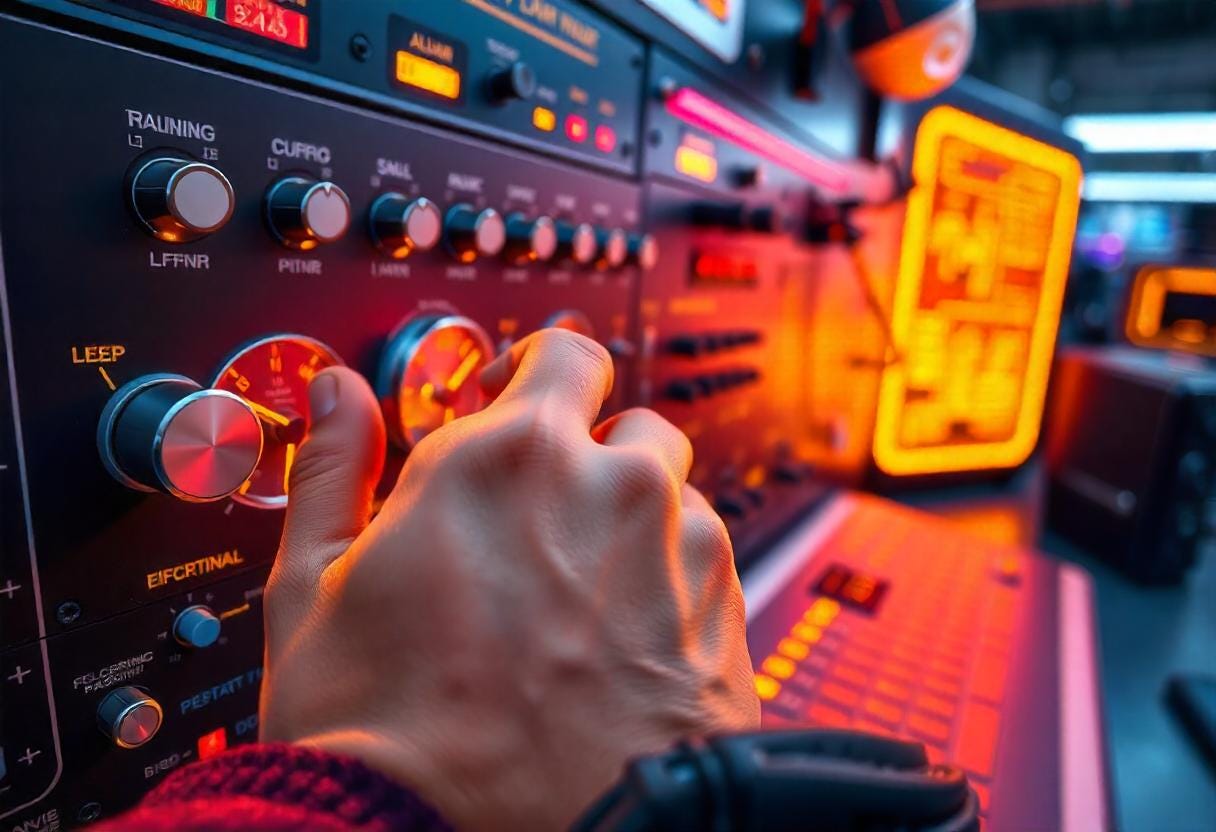
Fine Tuning Large Language Models Llms By Shaw Talebi Towards There are two methods businesses can use to unlock the full potential of large language models (llms). they are the retrieval augmented generation (rag) and fine tuning. both these methods operate by customizing the llm for specific use cases. however, the methodologies that these two follow are completely different. # exploring fine tuning in llms. fine tuning is a pivotal technique in optimizing large language models (fine tuning). this method involves tailoring pre trained models to specific tasks or domains, enhancing their performance and adaptability. there are various approaches to fine tuning that cater to different needs and objectives.
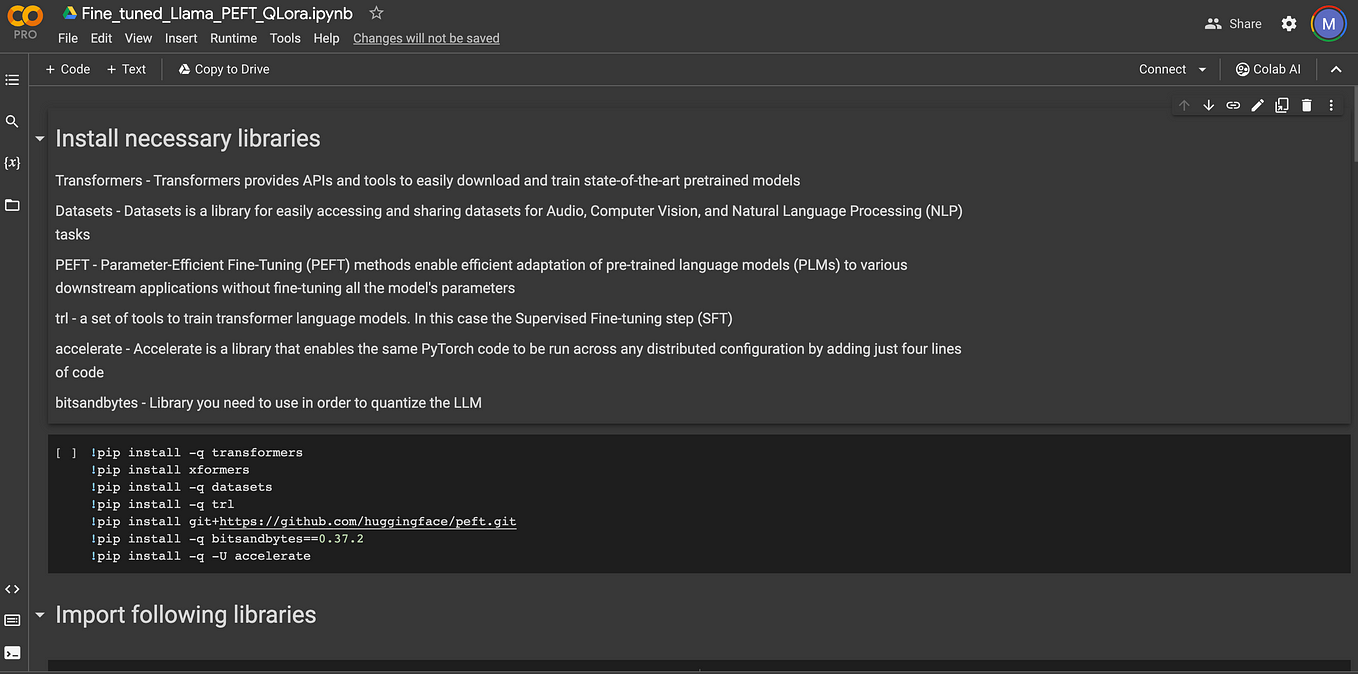
Fine Tuning Large Language Models Llms By Shawhin Talebi Towards