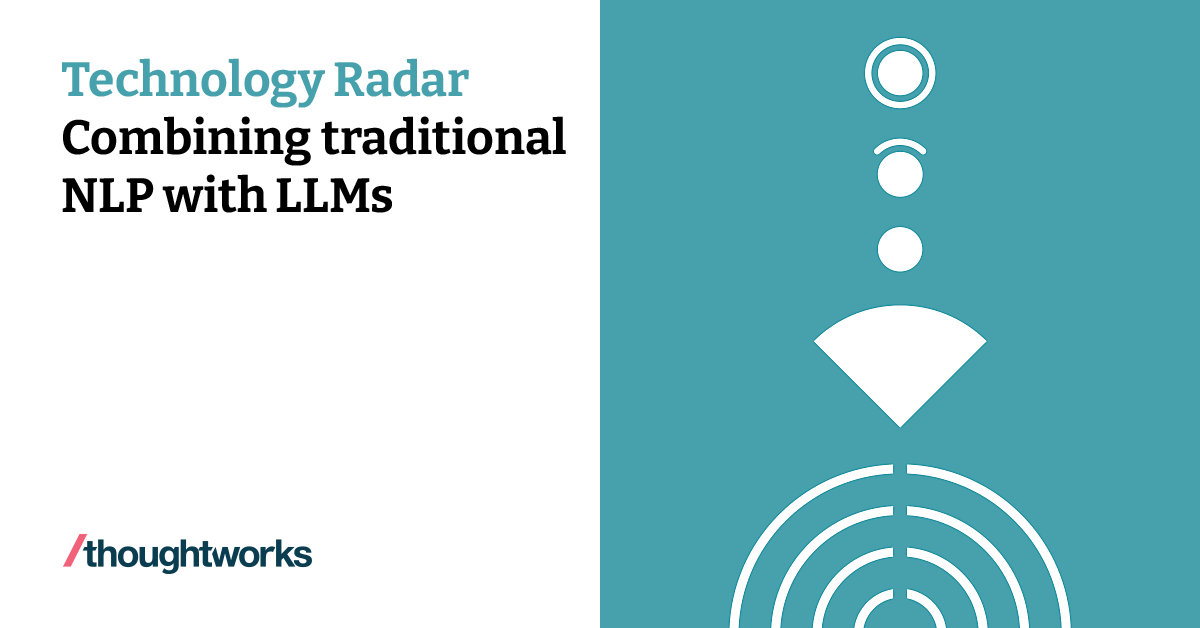
Combining Traditional Nlp With Llms Technology Radar Thoughtworks The field of nlp has changed with the rise of llms, but nlp still has a role to play. apply nlp techniques to scraped data and learn about tokenization, stemming, lemmatization, removing stop words, and more nlp techniques. In particular, the emergence of large language models (llms) like gpt 3 has transformed many nlp tasks. however, traditional nlp techniques still have an important role to play. in this post, we‘ll explore some foundational nlp techniques and examine how they compare and contrast with modern llms.

Buy Mastering Nlp From Foundations To Llms Applying Advanced With the rise of deep learning, neural language models (nlms) emerged, introducing more efficient ways to understand and generate language. techniques like word2vec revolutionized how words. It’s 2025, and we’re deep into the llm era where nlp isn’t just about rule based techniques or even classical deep learning models. we’re working with llms, embeddings, retrieval augmented generation (rag), and ai agents that can reason, retrieve, and respond with great accuracy 🚀 (& some hallucinations). Natural language processing (nlp) and large language models (llms) are two key milestones in this journey. the advent of transformer technology has marked a clear “before and after” moment, revolutionizing ai’s ability to understand and generate language. Llms completely changed the scope of language understanding and generation. this article reveals the differences between llms and traditional nlp based on their structures, functionalities, domains of application and weaknesses. the future of nlp: a hybrid approach?.
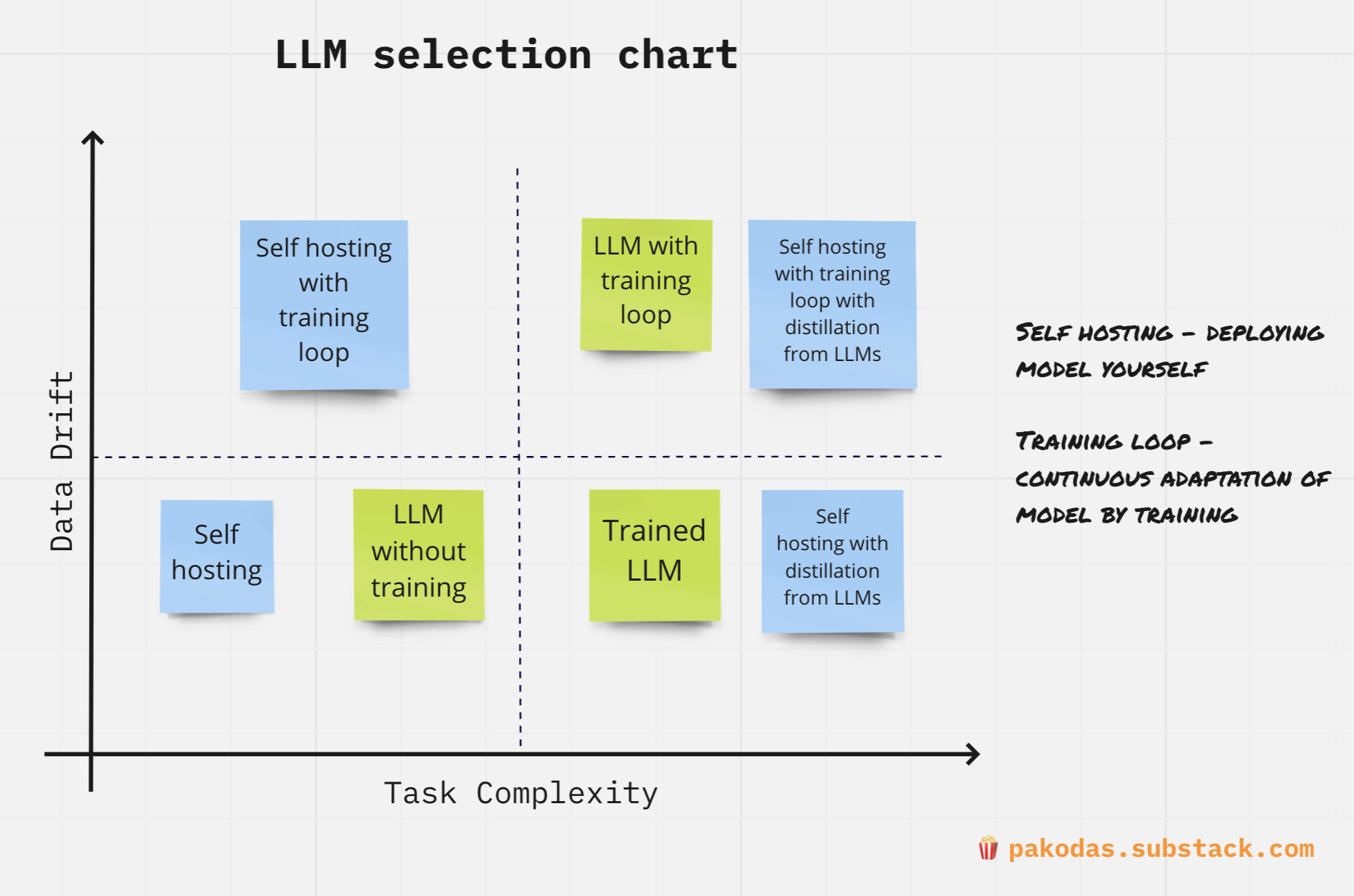
Llm Chronicles 1 Will Llms Make Nlp Scientists Jobless Natural language processing (nlp) and large language models (llms) are two key milestones in this journey. the advent of transformer technology has marked a clear “before and after” moment, revolutionizing ai’s ability to understand and generate language. Llms completely changed the scope of language understanding and generation. this article reveals the differences between llms and traditional nlp based on their structures, functionalities, domains of application and weaknesses. the future of nlp: a hybrid approach?. Traditional language models refer to the earlier generations of natural language processing (nlp) models that were designed to understand and generate human language. traditional lms, often based on n gram models and statistical techniques, laid the foundation for many nlp applications. From rudimentary techniques like n grams to the sophisticated large language models (llms) of today, the journey of nlp is a testament to the rapid advancements in artificial intelligence and. Traditionally, nlp has been broken down into smaller, manageable problems such as text classification, named entity recognition (ner), and summarization. each of these tasks requires specialized models and extensive training data, making the process labor intensive and time consuming. Large language models (llms) have revolutionized the field of natural language processing (nlp) by offering capabilities that traditional nlp techniques struggle to match. this section delves into the comparative strengths and weaknesses of llms versus traditional methods, providing a detailed analysis of their functionalities and applications.

Llms Vs Traditional Language Models A Comparative Analysis Traditional language models refer to the earlier generations of natural language processing (nlp) models that were designed to understand and generate human language. traditional lms, often based on n gram models and statistical techniques, laid the foundation for many nlp applications. From rudimentary techniques like n grams to the sophisticated large language models (llms) of today, the journey of nlp is a testament to the rapid advancements in artificial intelligence and. Traditionally, nlp has been broken down into smaller, manageable problems such as text classification, named entity recognition (ner), and summarization. each of these tasks requires specialized models and extensive training data, making the process labor intensive and time consuming. Large language models (llms) have revolutionized the field of natural language processing (nlp) by offering capabilities that traditional nlp techniques struggle to match. this section delves into the comparative strengths and weaknesses of llms versus traditional methods, providing a detailed analysis of their functionalities and applications.