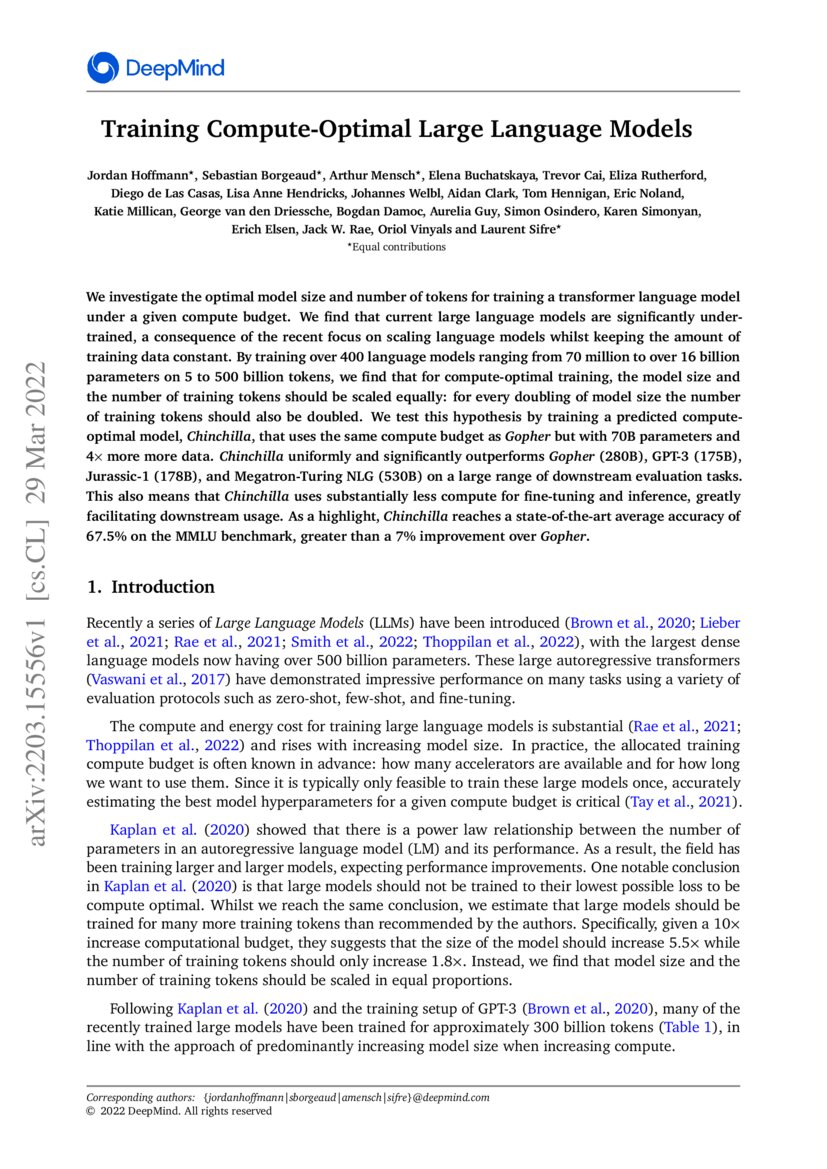
Training Compute Optimal Large Language Models Deepai We investigate the optimal model size and number of tokens for training a transformer language model under a given compute budget. we find that current large language models are significantly. We investigate the optimal model size and number of tokens for training a transformer language model under a given compute budget. we find that current large language models are significantly undertrained, a consequence of the recent focus on scaling language models whilst keeping the amount of training data constant.
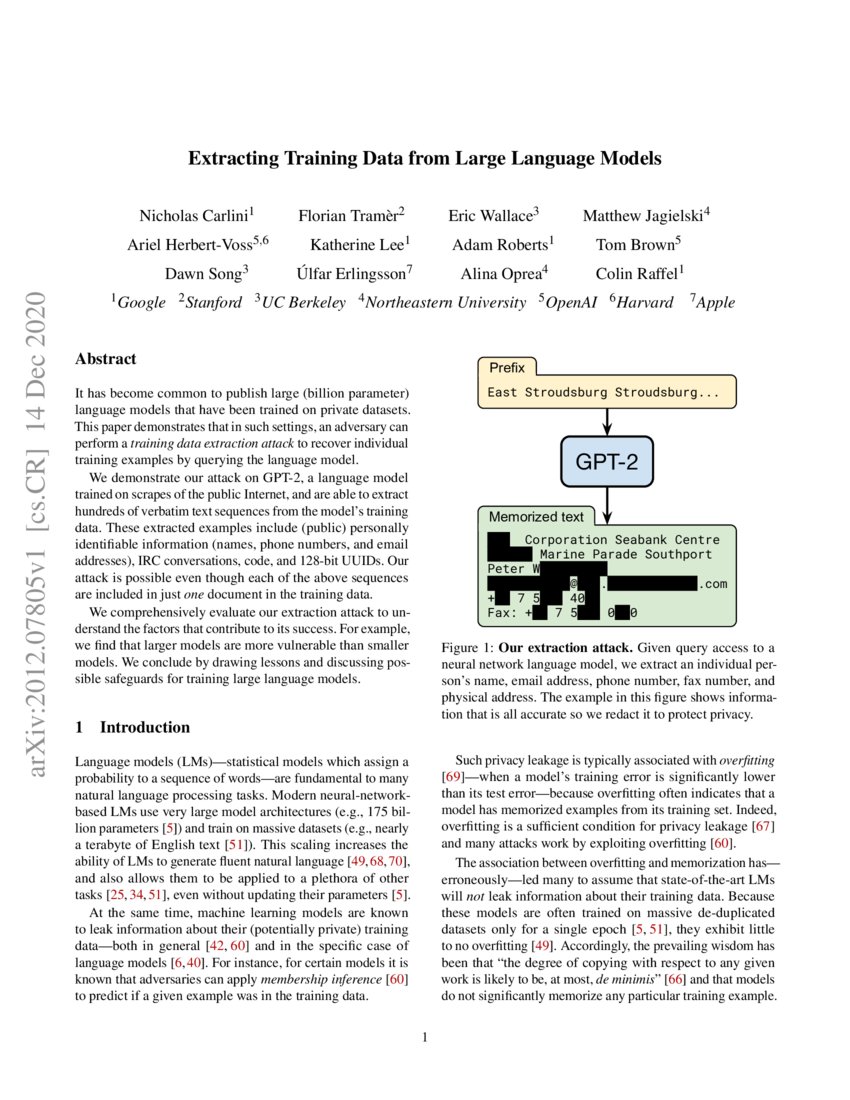
Extracting Training Data From Large Language Models Deepai We investigate the optimal model size and number of tokens for training a trans former language model under a given compute budget. we find that current large language models are significantly undertrained, a consequence of the recent focus on scaling language models whilst keeping the amount of training data constant. Large deep learning models have achieved state of the art performance across various natural language processing (nlp) tasks and demonstrated remarkable few shot learning performance. however, training them is often challenging and resource intensive. Language models have been getting bigger q1: why do we care about studying scaling law of llms? that’s a lot, but at least few shot means the model only has to be trained once? this may be true, but is increasing model size the most efficient way of improving performance? how should we allocate c to n and d?. We investigate the optimal model size and number of tokens for training a transformer language model under a given compute budget. we find that current large language models are significantly undertrained, a consequence of the recent focus on scaling language models whilst keeping the amount of training data constant.
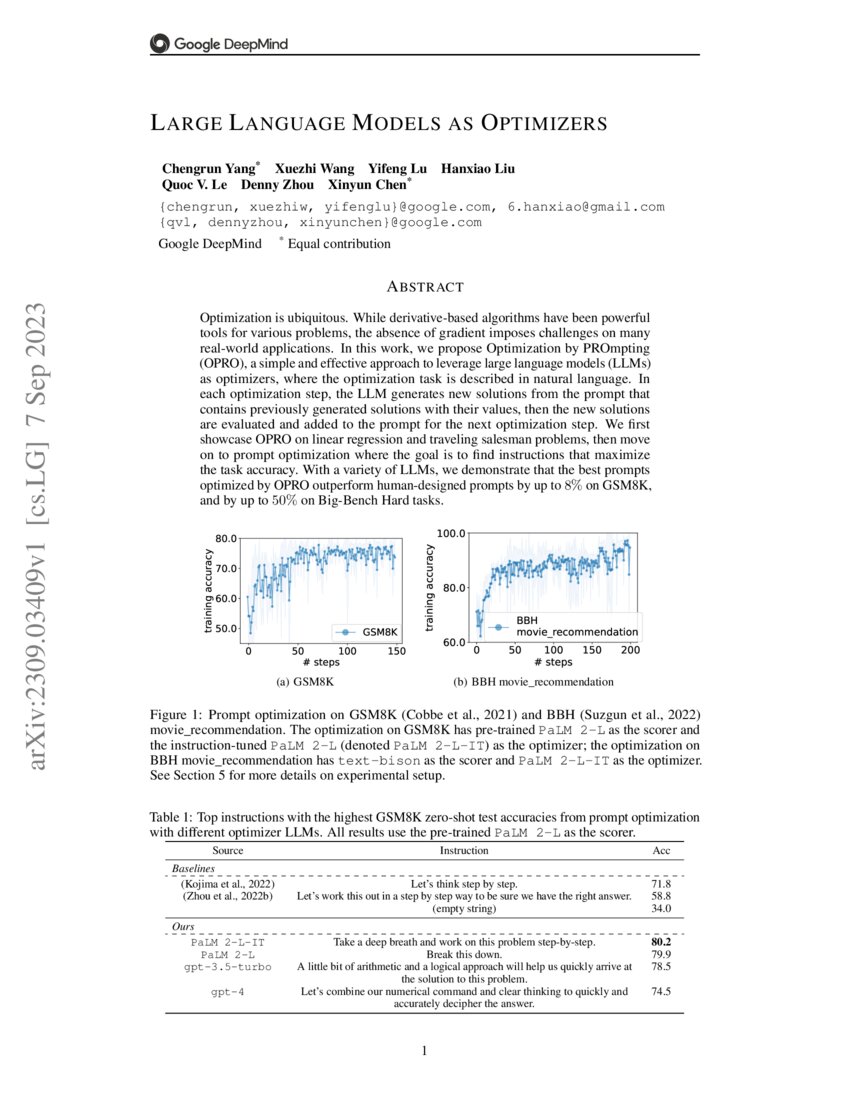
Large Language Models As Optimizers Deepai Language models have been getting bigger q1: why do we care about studying scaling law of llms? that’s a lot, but at least few shot means the model only has to be trained once? this may be true, but is increasing model size the most efficient way of improving performance? how should we allocate c to n and d?. We investigate the optimal model size and number of tokens for training a transformer language model under a given compute budget. we find that current large language models are significantly undertrained, a consequence of the recent focus on scaling language models whilst keeping the amount of training data constant. We investigate the optimal model size and number of tokens for training a transformer language model under a given compute budget. we find that current large language models are significantly undertrained, a consequence of the recent focus on scaling language models whilst keeping the amount of training data constant. We study recent research advances that improve large language models through efficient pre training and scaling, and open datasets and tools. we combine these advances to introduce cerebras gpt, a family of open compute optimal language models scaled from 111m to 13b parameters. This paper challenges the well established paradigm for building any to any networks for training large language models (llms). we show that llms exhibit a unique communication pattern where only small groups of gpus require high bandwidth any to any communication within them, to achieve near optimal training performance. Given a fixed flops budget, how should one trade off model size and the number of training tokens? this paper investigates the optimal model size and number of tokens for training a transformer language model under a given compute budget.
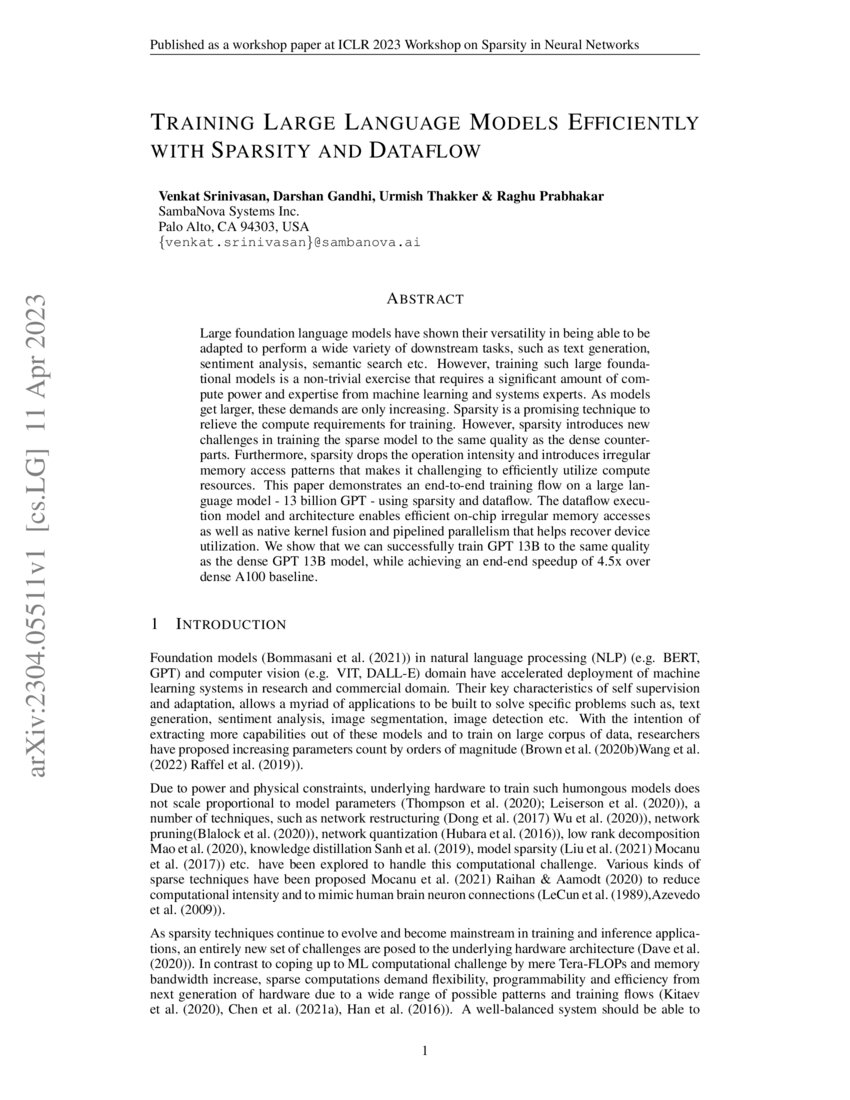
Training Large Language Models Efficiently With Sparsity And Dataflow We investigate the optimal model size and number of tokens for training a transformer language model under a given compute budget. we find that current large language models are significantly undertrained, a consequence of the recent focus on scaling language models whilst keeping the amount of training data constant. We study recent research advances that improve large language models through efficient pre training and scaling, and open datasets and tools. we combine these advances to introduce cerebras gpt, a family of open compute optimal language models scaled from 111m to 13b parameters. This paper challenges the well established paradigm for building any to any networks for training large language models (llms). we show that llms exhibit a unique communication pattern where only small groups of gpus require high bandwidth any to any communication within them, to achieve near optimal training performance. Given a fixed flops budget, how should one trade off model size and the number of training tokens? this paper investigates the optimal model size and number of tokens for training a transformer language model under a given compute budget.

Training Compute Optimal Large Language Models Deepmind S 70b This paper challenges the well established paradigm for building any to any networks for training large language models (llms). we show that llms exhibit a unique communication pattern where only small groups of gpus require high bandwidth any to any communication within them, to achieve near optimal training performance. Given a fixed flops budget, how should one trade off model size and the number of training tokens? this paper investigates the optimal model size and number of tokens for training a transformer language model under a given compute budget.

Training Compute Optimal Large Language Models Papers With Code