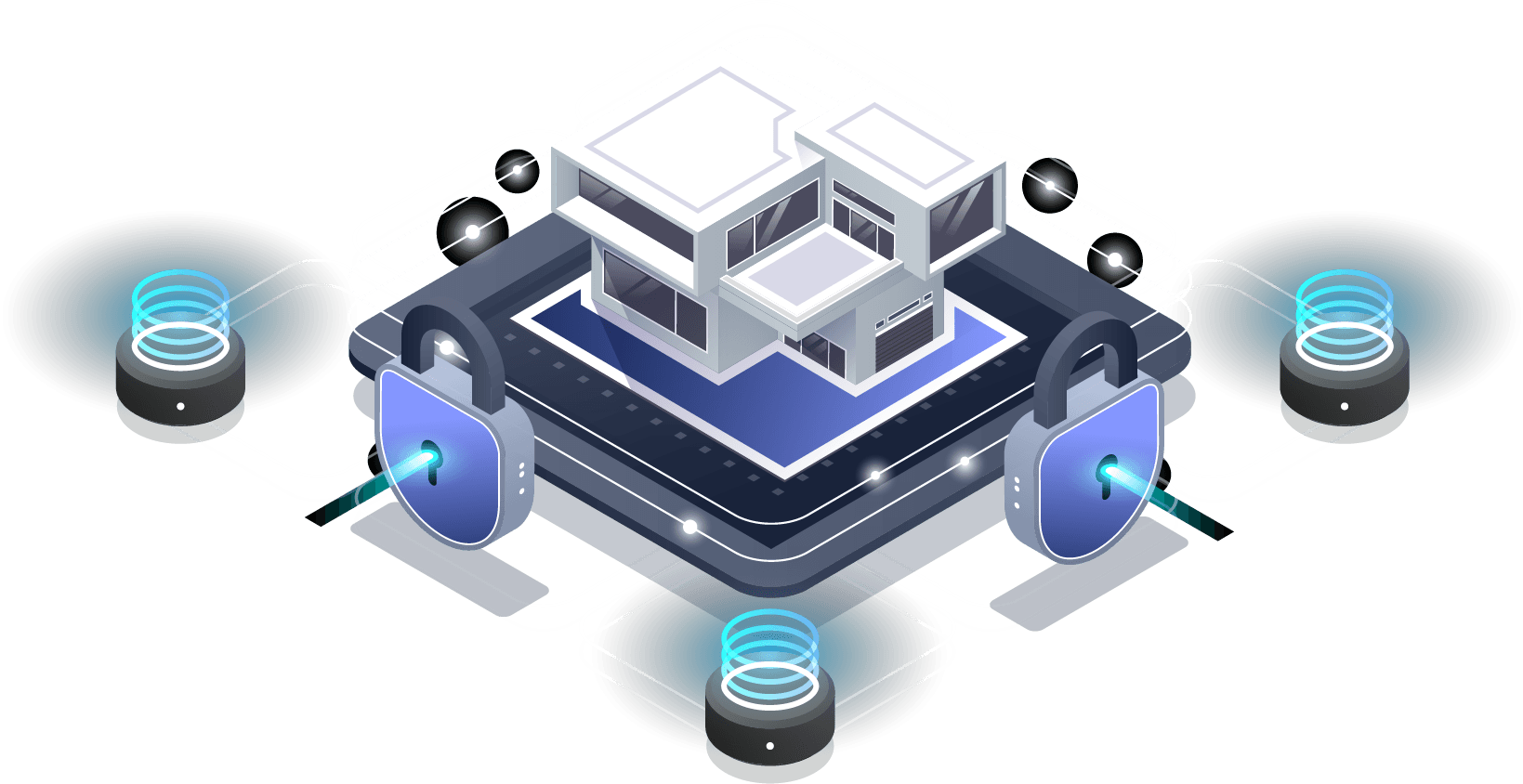
Understanding Data Lakehouse Architecture In this blog, we'll explore the nuances of data lakehouse architecture, and learn about its components, purposes, implementation strategies, advancements in 2023, and its imperative role in navigating the complexities of modern data management. A data lakehouse is a modern data architecture that seamlessly merges the strengths of two well known data storage paradigms: data lakes and data warehouses. at its core, a data lakehouse is designed to store vast amounts of data, be it structured, semi structured, or unstructured, much like a data lake.
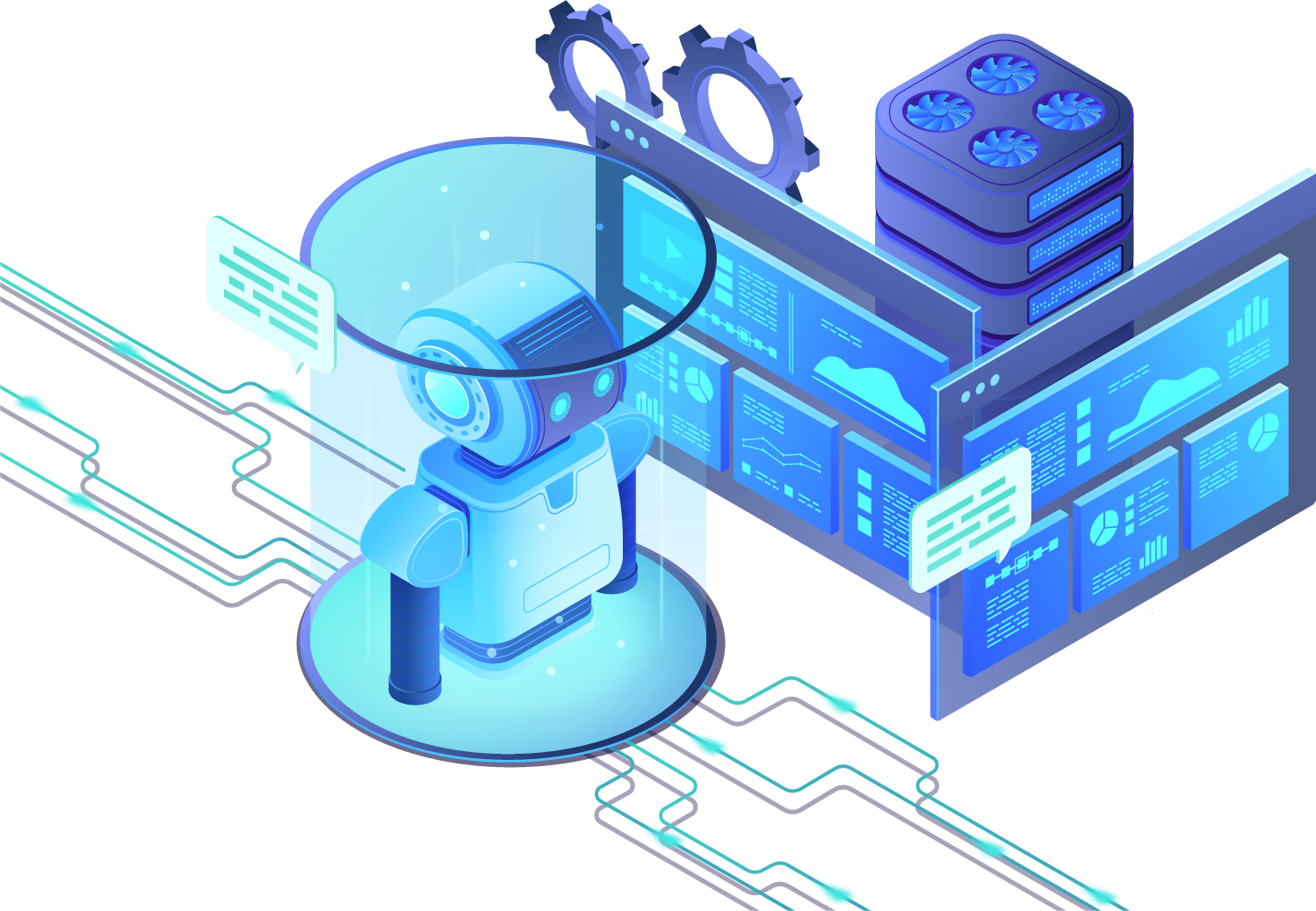
Streamlining Ml With Data Lakehouse Dview Io This paper argues that the data warehouse architecture as we know it today will wane in the coming years and be replaced by a new architectural pattern, which we refer to as the lakehouse, char acterized by (i) open direct access data formats, such as apache parquet and orc, (ii) first class support for machine learning and. The key concept of data lakehouse is to connect the data lake with all data services. the lake house architecture consists of 5 layers as follows: data lakehouse architecture (image by author). A new architecture concept, data lakehouse, has recently been introduced as a next step in the analytical data storage. the data lakehouse architecture should be able to deal with currently insurmountable challenges that both data warehouses and data lakes cannot overcome. in this article, a current state of the data lakehouse architecture and. The lakehouse architecture aims to overcome the drawbacks of traditional data lakes and data warehouses by offering a unified, flexible, and high performing platform. here is a deeper dive into.
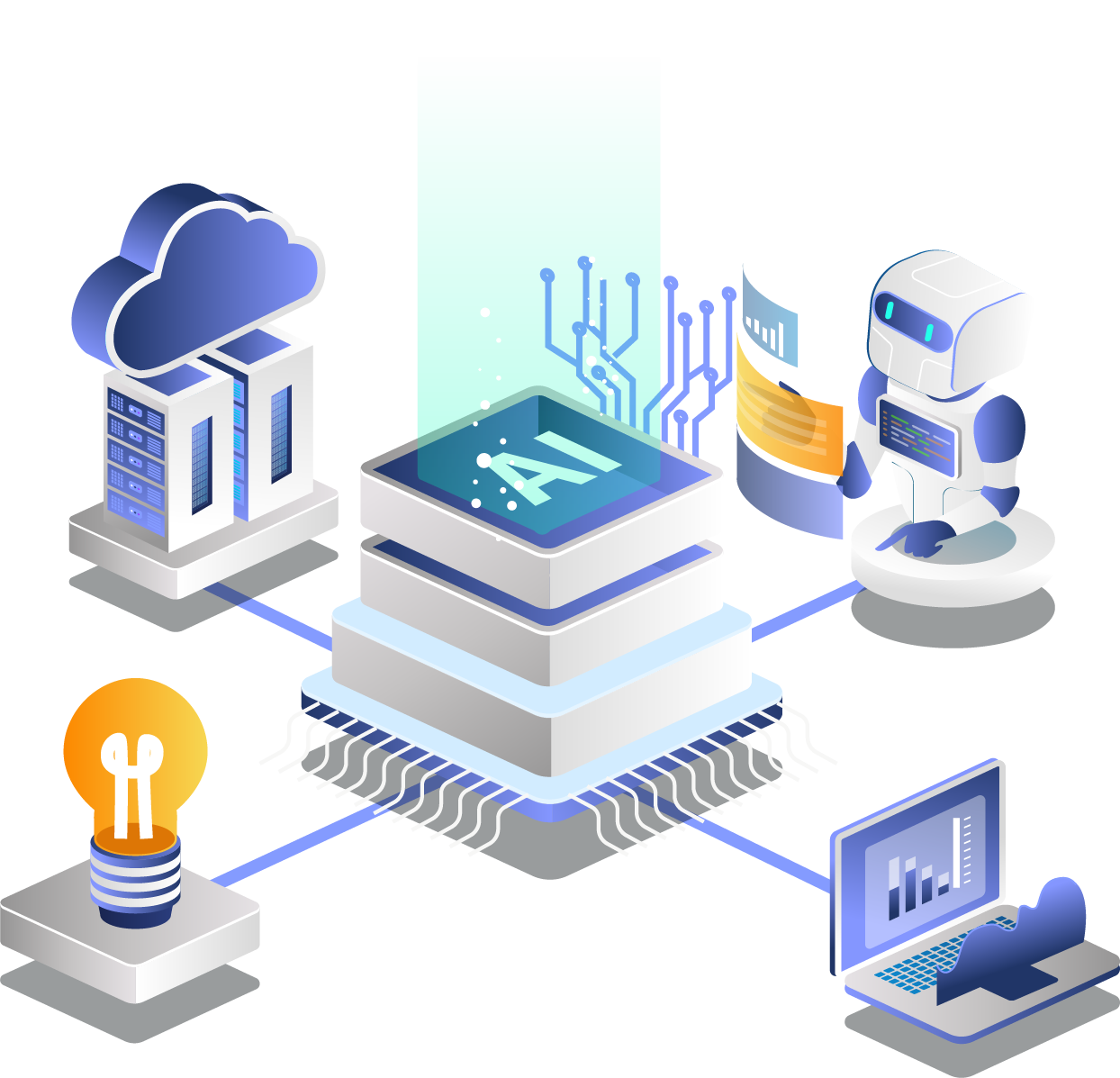
Ai Data Lakehouse The Future Of Real Time Analytics Dview Io A new architecture concept, data lakehouse, has recently been introduced as a next step in the analytical data storage. the data lakehouse architecture should be able to deal with currently insurmountable challenges that both data warehouses and data lakes cannot overcome. in this article, a current state of the data lakehouse architecture and. The lakehouse architecture aims to overcome the drawbacks of traditional data lakes and data warehouses by offering a unified, flexible, and high performing platform. here is a deeper dive into. At its core, a data lakehouse architecture consists of three layers: storage, compute, and catalog. the storage layer stores raw structured and unstructured data without any modifications. the compute layer enables processing and analysis capabilities on top of this stored information by leveraging various engines like apache spark or presto. Data lakehouse architecture, with its blend of structured and unstructured data handling capabilities, are poised to redefine the standard for ml workflows. their ability to offer real time analytics, coupled with centralized data storage, makes them an attractive proposition for businesses aiming for agile decision making. With data becoming more diverse and complex, the data lakehouse architecture may be the best means for managing that data and helping fuel data driven decisions. speaking on july 18 during a webinar hosted by transforming data with intelligence (tdwi), fern halper, the research and advisory firm's senior research director for advanced analytics. In this article, we’ll peel back the 5 layers that make up data lakehouse architecture: data ingestion, data storage, metadata, api, and data consumption, understand the expanded opportunities a data lakehouse opens up for generative ai, and how to maintain data quality throughout the pipeline with data observability.
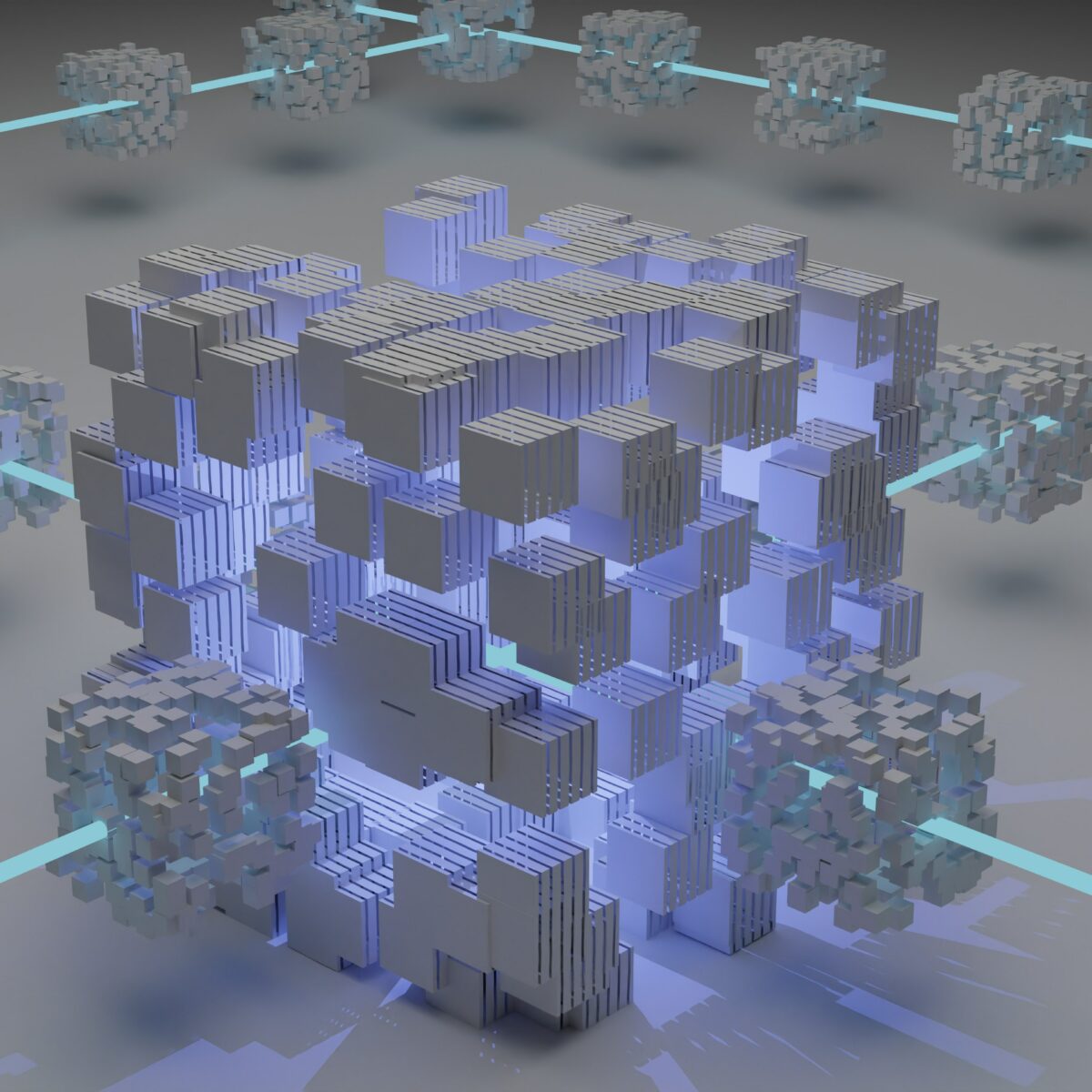
The Rise Of Data Lakehouses Architecture Concepts Advantages At its core, a data lakehouse architecture consists of three layers: storage, compute, and catalog. the storage layer stores raw structured and unstructured data without any modifications. the compute layer enables processing and analysis capabilities on top of this stored information by leveraging various engines like apache spark or presto. Data lakehouse architecture, with its blend of structured and unstructured data handling capabilities, are poised to redefine the standard for ml workflows. their ability to offer real time analytics, coupled with centralized data storage, makes them an attractive proposition for businesses aiming for agile decision making. With data becoming more diverse and complex, the data lakehouse architecture may be the best means for managing that data and helping fuel data driven decisions. speaking on july 18 during a webinar hosted by transforming data with intelligence (tdwi), fern halper, the research and advisory firm's senior research director for advanced analytics. In this article, we’ll peel back the 5 layers that make up data lakehouse architecture: data ingestion, data storage, metadata, api, and data consumption, understand the expanded opportunities a data lakehouse opens up for generative ai, and how to maintain data quality throughout the pipeline with data observability.
Data Lakehouse Game Changing Impact For Data Democracy Dview Posted With data becoming more diverse and complex, the data lakehouse architecture may be the best means for managing that data and helping fuel data driven decisions. speaking on july 18 during a webinar hosted by transforming data with intelligence (tdwi), fern halper, the research and advisory firm's senior research director for advanced analytics. In this article, we’ll peel back the 5 layers that make up data lakehouse architecture: data ingestion, data storage, metadata, api, and data consumption, understand the expanded opportunities a data lakehouse opens up for generative ai, and how to maintain data quality throughout the pipeline with data observability.
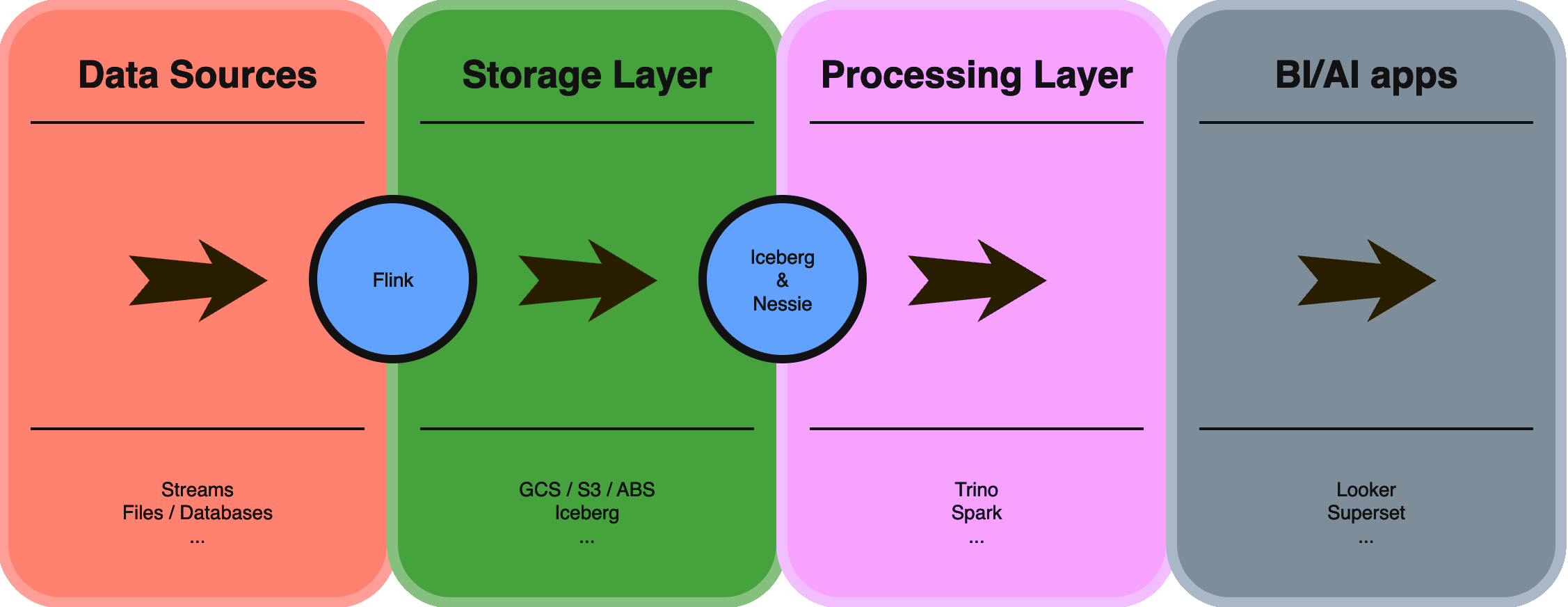
Data Lakehouse Architecture With Flink Sql