Comparison Of Three Deep Learning Technique For Detection Iot Malware Deep learning has shown promise in effectively detecting and preventing cyberattacks on iot devices. although ids is vital for safeguarding sensitive information by identifying and mitigating suspicious activities, conventional ids solutions grapple with challenges in the iot context. In this study, we explore the integration of explainable artificial intelligence (xai) with deep learning models to enhance the detection of advanced persistent threats (apts). apts are prolonged and targeted cyberattacks in which an intruder gains access to a network and remains undetected for an extended period.

Pdf Cyber Security Threats Detection In Internet Of Things Using Deep Our predictive model, developed using advanced machine learning and deep learning techniques, forecasts the frequency of cyber attacks within specific time windows, demonstrating over a 15% improvement in accuracy compared to conventional baseline models. This research explores the use of deep learning and machine learning techniques in cybersecurity to detect and mitigate cyber threats. specifically, this study focuses on long short term memory (lstm) and graph neural networks (gnn) to analyse network traffic and system logs for patterns, anomalies, and malicious activities. We have suggested a hybrid deep learning (dl) strategy in this study to identify malware infected programs and files that have been illegally distributed over the iot environment. This repository contains the implementation of a deep learning model designed to detect cyber threats using the beth dataset, which simulates real world logs. by successfully developing this model, we aim to enhance cybersecurity measures and contribute to organizational security.
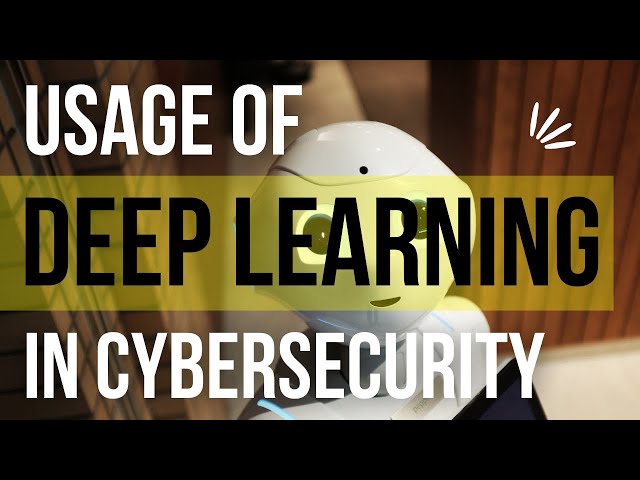
How Deep Learning Is Changing Cyber Security Reason Town We have suggested a hybrid deep learning (dl) strategy in this study to identify malware infected programs and files that have been illegally distributed over the iot environment. This repository contains the implementation of a deep learning model designed to detect cyber threats using the beth dataset, which simulates real world logs. by successfully developing this model, we aim to enhance cybersecurity measures and contribute to organizational security. Supervised learning and unsupervised learning algorithms together with the deep learning models, for instance, cnn and rnns are used to identify patterns and monitor and detect anomalies in real. The efficacy of the deep learning model can be evaluated using the study for identification of different types of cyber security threats such as dos, ddos, ransomware, password hacking and information threat. the proposed model identifies and mitigates the threat in less time and it was tested with two different data sets bot and ton. To stay ahead of the emerging threat of ai powered cyberattacks, we’ve adapted tried and tested cybersecurity evaluation frameworks, such as mitre att&ck. these frameworks enabled us to evaluate threats across the end to end cyber attack chain, from reconnaissance to action on objectives, and across a range of possible attack scenarios. Deep learning can be seen as a step up in the fight against cyber threats. utilizing these neural networks enable organization to react quickly and accurately to threats in ways previously unfathomable.
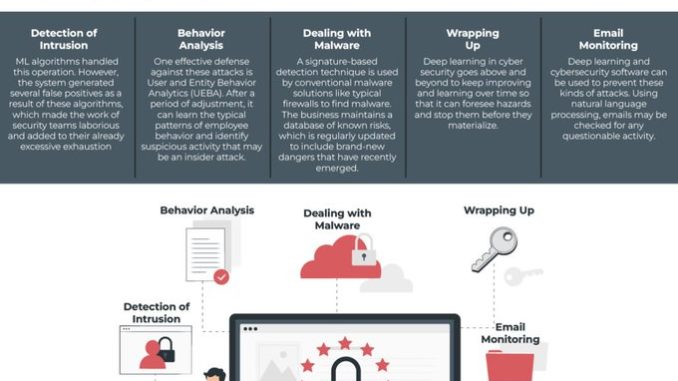
How Deep Learning Has Proved To Be Useful For Cyber Security La Supervised learning and unsupervised learning algorithms together with the deep learning models, for instance, cnn and rnns are used to identify patterns and monitor and detect anomalies in real. The efficacy of the deep learning model can be evaluated using the study for identification of different types of cyber security threats such as dos, ddos, ransomware, password hacking and information threat. the proposed model identifies and mitigates the threat in less time and it was tested with two different data sets bot and ton. To stay ahead of the emerging threat of ai powered cyberattacks, we’ve adapted tried and tested cybersecurity evaluation frameworks, such as mitre att&ck. these frameworks enabled us to evaluate threats across the end to end cyber attack chain, from reconnaissance to action on objectives, and across a range of possible attack scenarios. Deep learning can be seen as a step up in the fight against cyber threats. utilizing these neural networks enable organization to react quickly and accurately to threats in ways previously unfathomable.

Pdf Cyber Attack Detection Using Deep Learning To stay ahead of the emerging threat of ai powered cyberattacks, we’ve adapted tried and tested cybersecurity evaluation frameworks, such as mitre att&ck. these frameworks enabled us to evaluate threats across the end to end cyber attack chain, from reconnaissance to action on objectives, and across a range of possible attack scenarios. Deep learning can be seen as a step up in the fight against cyber threats. utilizing these neural networks enable organization to react quickly and accurately to threats in ways previously unfathomable.