%2C such as OpenAI's GPT-4o and Google's PaliGemma%2C to address these challenges. By leveraging their Visual Question Answering capabilities and 0-shot Chain-of-Thought (CoT) reasoning%2C our goal is to provide clear%2C human-understandable explanations for model decisions. Our experiments on the CEDAR handwriting dataset demonstrate that VLMs offer enhanced interpretability%2C reduce the need for large training datasets%2C and adapt better to diverse handwriting styles. However%2C results show that the CNN-based ResNet-18 architecture outperforms the 0-shot CoT prompt engineering approach with GPT-4o (Accuracy: 70%25) and supervised fine-tuned PaliGemma (Accuracy: 71%25)%2C achieving an accuracy of 84%25 on the CEDAR AND dataset. These findings highlight the potential of VLMs in generating human-interpretable decisions while underscoring the need for further advancements to match the performance of specialized deep learning models.)
Vision Language Model Based Handwriting Verification Ai Research This paper explores using vision language models (vlms), such as openai's gpt 4o and google's paligemma, to address these challenges. by leveraging their visual question answering capabilities and 0 shot chain of thought (cot) reasoning, our goal is to provide clear, human understandable explanations for model decisions. This paper explores using vision language models (vlms), such as openai's gpt 4o and google's paligemma, to address these challenges. by leveraging their visual question answering capabilities and 0 shot chain of thought (cot) reasoning, our goal is to provide clear, human understandable explanations for model decisions.

Vision Language Model Based Handwriting Verification Ai Research Our study aims to use vlm in handwriting verification. we have chosen openai’s gpt 4o vlm for its strong visual question answering (vqa) capabilities. using their api, we prompt gpt 4o [gpt, 2024] with 0 shot chain of thought (cot) reasoning. In this paper, we study online handwriting recognition with vlms, going beyond naive ocr. we propose a novel tokenized representation of digital ink (online handwriting) that includes both a time ordered sequence of strokes as text, and as image. This repository provides implemantation of the experiments descibed in the paper vlm hv : vision language model based handwriting verification. authors: mihir chauhan, abhishek satbhai, mohammad abuzar shaikh, mir basheer ali, bina ramamurthy, mingchen gao, siwei lyu and sargur srihari. This paper explores using vision language models (vlms), such as openai’s gpt 4o and google’s paligemma, to address these challenges. by leveraging their visual question answering capabilities and 0 shot chain of thought (cot) reasoning, our goal is to provide clear, human understandable explanations for model decisions.
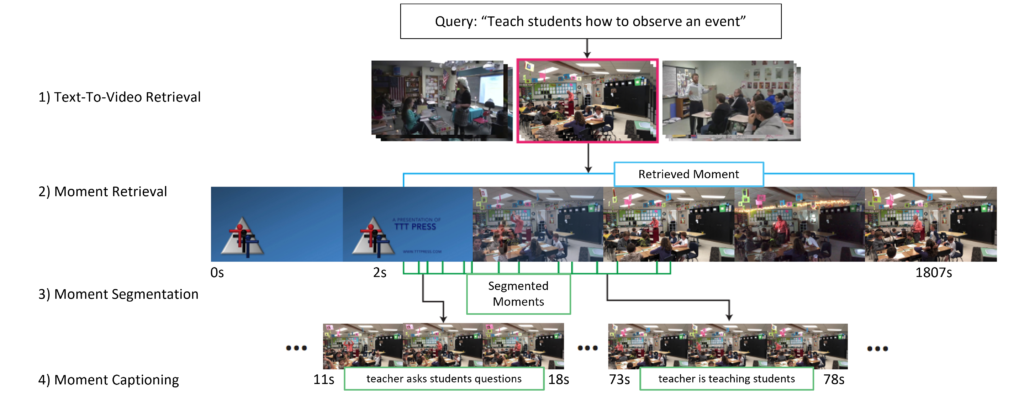
Classroom Video Summarization With Ai Based Vision Language Modeling This repository provides implemantation of the experiments descibed in the paper vlm hv : vision language model based handwriting verification. authors: mihir chauhan, abhishek satbhai, mohammad abuzar shaikh, mir basheer ali, bina ramamurthy, mingchen gao, siwei lyu and sargur srihari. This paper explores using vision language models (vlms), such as openai’s gpt 4o and google’s paligemma, to address these challenges. by leveraging their visual question answering capabilities and 0 shot chain of thought (cot) reasoning, our goal is to provide clear, human understandable explanations for model decisions. We propose a vision based system that recognizes handwriting in mid air. the system does not depend on sensors or markers attached to the users and allows unrestricted character and word input from any position. On the verge of this fluctuating drawback for control systems, this manuscript introduces a spatial variation dependent verification (svv) scheme using textural features (tf). the handwritten and digital signatures are first verified for their pixel intensities for identification point detection. To our knowledge, this research is the first to assess stroke based representations for online handwriting recognition within vlms. we show that our representation works effectively in fine tuning or efficient parameter tuning scenarios without needing adjustments to the model structure or vocabulary. This paper explores using vision language models (vlms), such as openai’s gpt 4o and google’s paligemma, to address these challenges. by leveraging their visual question answering capabilities and 0 shot chain of thought (cot) reasoning, our goal is to provide clear, human understandable explanations for model decisions.

Pdf Language Modelling For Handwriting Recognition We propose a vision based system that recognizes handwriting in mid air. the system does not depend on sensors or markers attached to the users and allows unrestricted character and word input from any position. On the verge of this fluctuating drawback for control systems, this manuscript introduces a spatial variation dependent verification (svv) scheme using textural features (tf). the handwritten and digital signatures are first verified for their pixel intensities for identification point detection. To our knowledge, this research is the first to assess stroke based representations for online handwriting recognition within vlms. we show that our representation works effectively in fine tuning or efficient parameter tuning scenarios without needing adjustments to the model structure or vocabulary. This paper explores using vision language models (vlms), such as openai’s gpt 4o and google’s paligemma, to address these challenges. by leveraging their visual question answering capabilities and 0 shot chain of thought (cot) reasoning, our goal is to provide clear, human understandable explanations for model decisions.
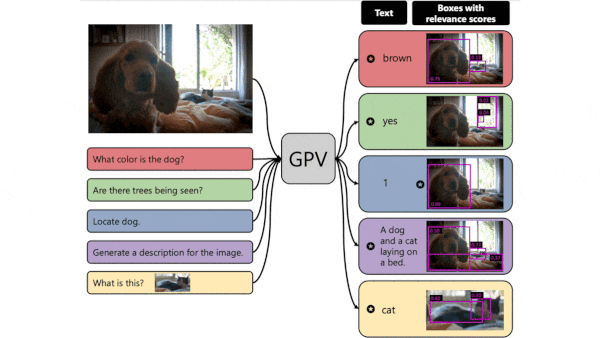
A General Purpose Ai For Vision And Language Tasks To our knowledge, this research is the first to assess stroke based representations for online handwriting recognition within vlms. we show that our representation works effectively in fine tuning or efficient parameter tuning scenarios without needing adjustments to the model structure or vocabulary. This paper explores using vision language models (vlms), such as openai’s gpt 4o and google’s paligemma, to address these challenges. by leveraging their visual question answering capabilities and 0 shot chain of thought (cot) reasoning, our goal is to provide clear, human understandable explanations for model decisions.

Overall Overview Of The Proposed Handwriting Recognition Model A