Github Mcnevesinf Causal Inference Adult Census Archaic census procedures based on the single 18th century term “enumeration” seem silly today — we merely need an accurate count of full time “residents”, but surely a measure of residents who can legally vote is fundamental to our system of government and nation state. Young voters drove record breaking turnout. 2020’s historic voter turnout gains were primarily driven by young voters. 18 29 year olds grew from 15% (2016) to 16% (2020) of the voting electorate, but the generational changes have been even more dramatic.
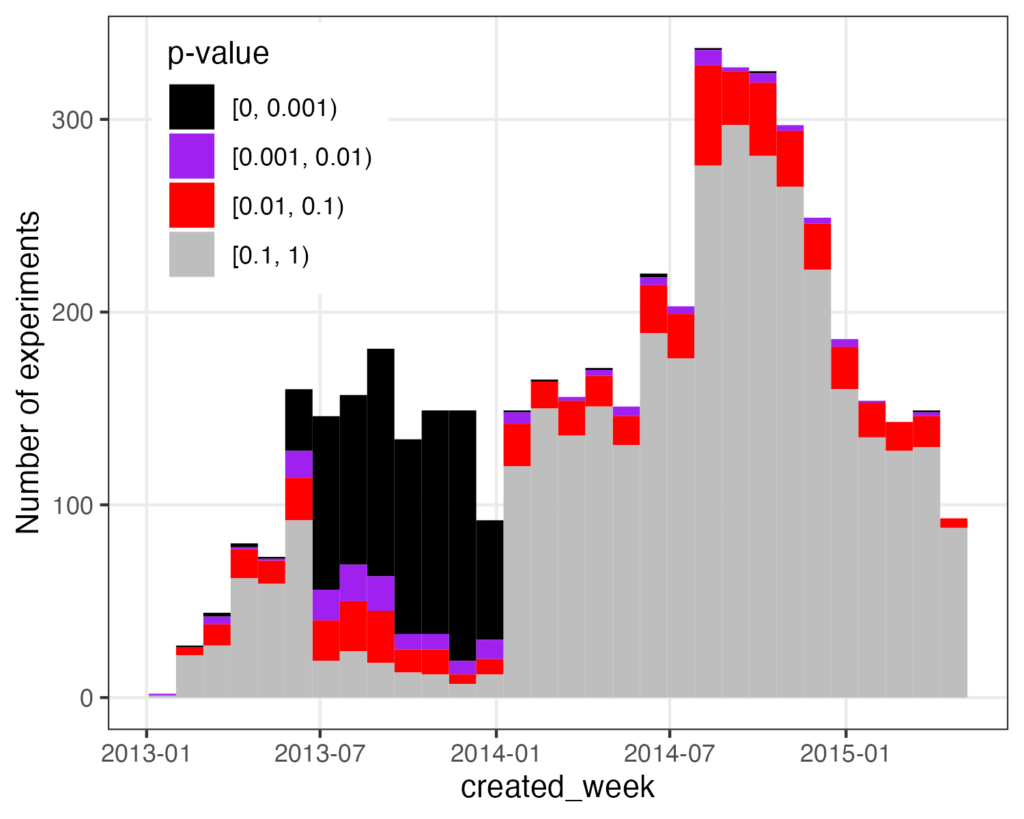
Causal Inference Statistical Modeling Causal Inference And Social Despite written guidance provided on the 2020 census on how to answer this question, doing so may be wrought with complexities and nuance from the pandemic. first, research reveals that respondents do not often read questionnaire instructions; they dive in and start answering. A review of the 2020 census experience, including rigorous assessment of the census bureau’s reconstruction reidentification studies under plausible scenarios of potential attack and comprehensive debriefing of users. To investigate whether this trade off is inevitable, we formulate a semiparametric model of causal inference with high dimensional corrupted data. we propose a procedure for data cleaning, estimation, and inference with data cleaning adjusted confidence intervals. Identify and develop statistical models (e.g., loglinear models, mixture models, and mixed effects models), associated methodologies, and computational tools for problems relevant to the census bureau.
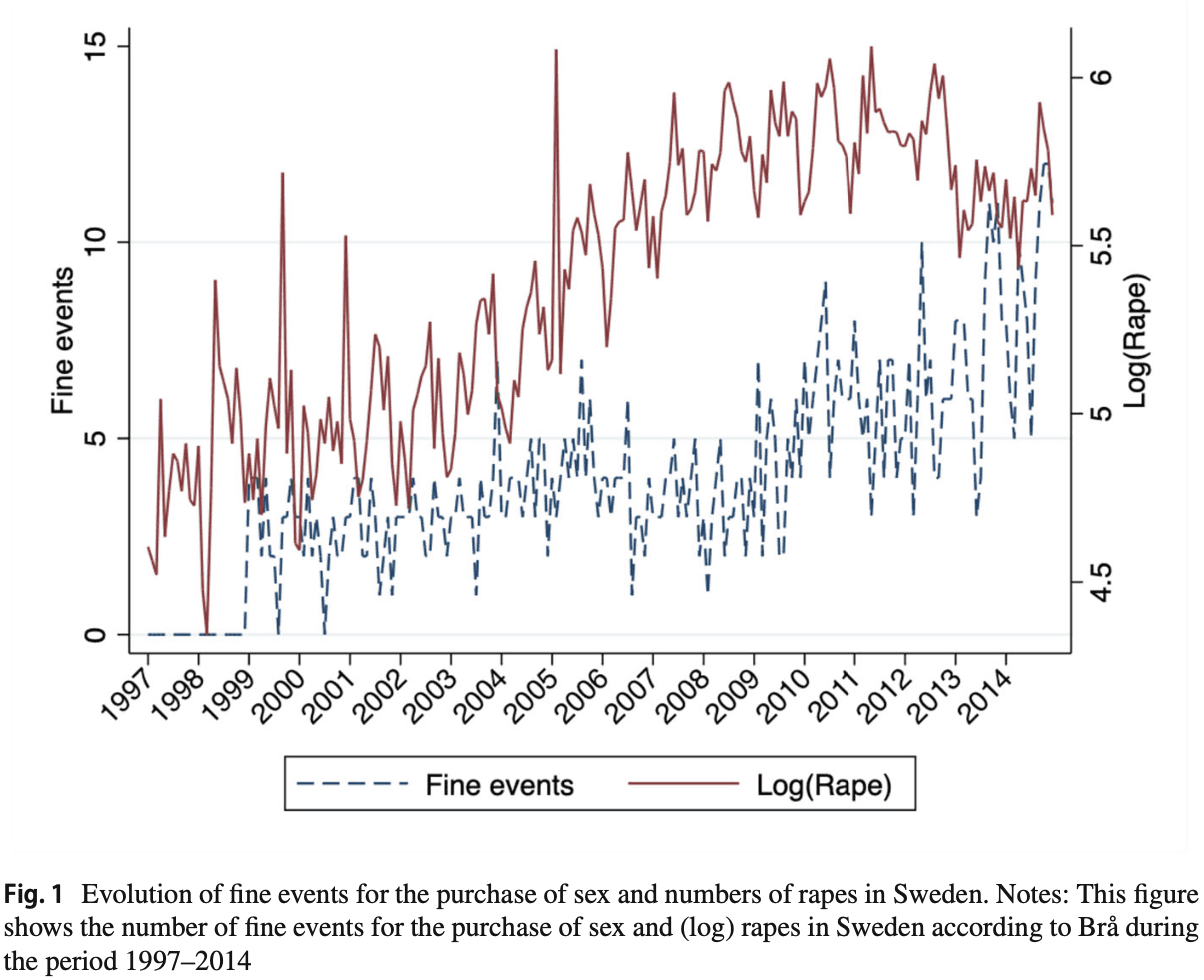
Causal Inference Statistical Modeling Causal Inference And Social To investigate whether this trade off is inevitable, we formulate a semiparametric model of causal inference with high dimensional corrupted data. we propose a procedure for data cleaning, estimation, and inference with data cleaning adjusted confidence intervals. Identify and develop statistical models (e.g., loglinear models, mixture models, and mixed effects models), associated methodologies, and computational tools for problems relevant to the census bureau. Statistical modeling (e.g., latent class models) for combining sample survey, census and or alternative source data. statistical techniques (e.g., classification methods, multiple imputation models) for using alternative data sources to augment or replace actual data collection. Survey sampling helps the census bureau assess the quality of each decennial census. estimates are produced by use of design based estimation techniques or model based estimation techniques. To motivate and introduce causal inference in introductory statistics or data science courses, we use simulated data and simple linear regression to show the effects of confounding and when one should or should not adjust for covariables. David a. freedman presents here a definitive synthesis of his approach to causal inference in the social sciences. he explores the foundations and limitations of statistical modeling, illustrating basic arguments with examples from political science, public policy, law, and epidemiology.
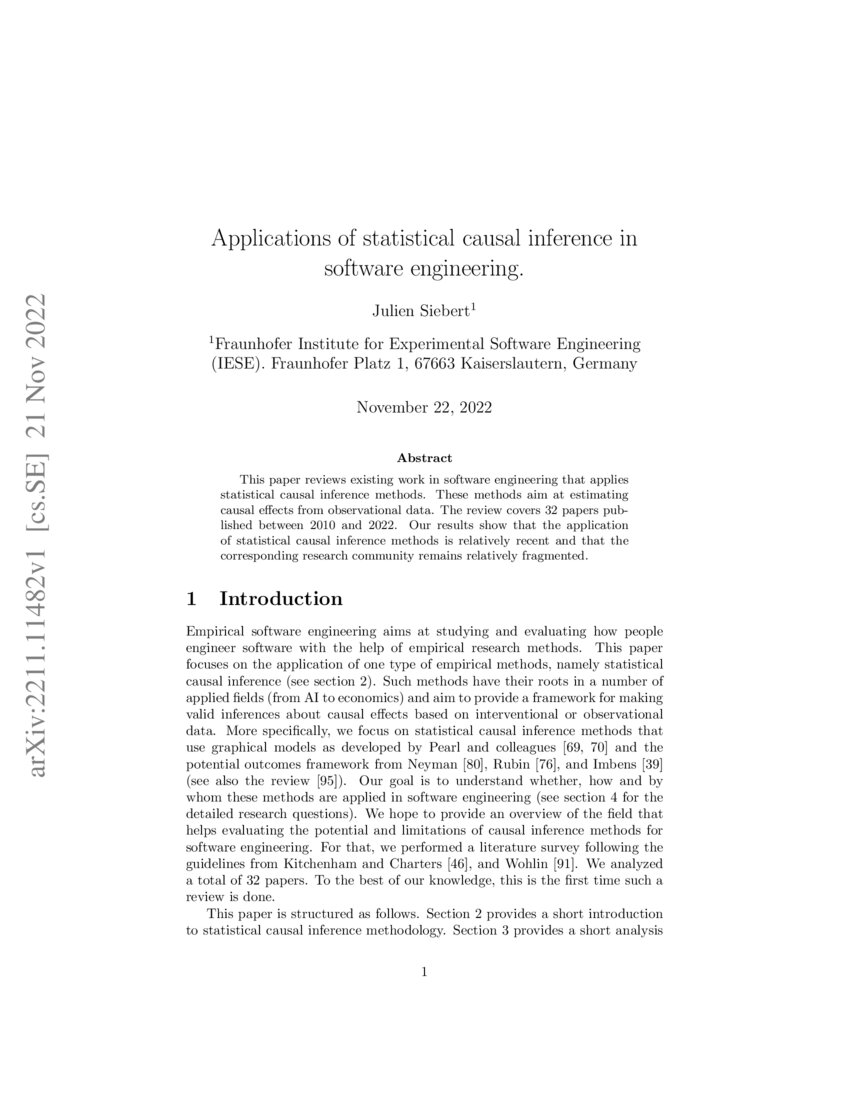
Applications Of Statistical Causal Inference In Software Engineering Statistical modeling (e.g., latent class models) for combining sample survey, census and or alternative source data. statistical techniques (e.g., classification methods, multiple imputation models) for using alternative data sources to augment or replace actual data collection. Survey sampling helps the census bureau assess the quality of each decennial census. estimates are produced by use of design based estimation techniques or model based estimation techniques. To motivate and introduce causal inference in introductory statistics or data science courses, we use simulated data and simple linear regression to show the effects of confounding and when one should or should not adjust for covariables. David a. freedman presents here a definitive synthesis of his approach to causal inference in the social sciences. he explores the foundations and limitations of statistical modeling, illustrating basic arguments with examples from political science, public policy, law, and epidemiology.
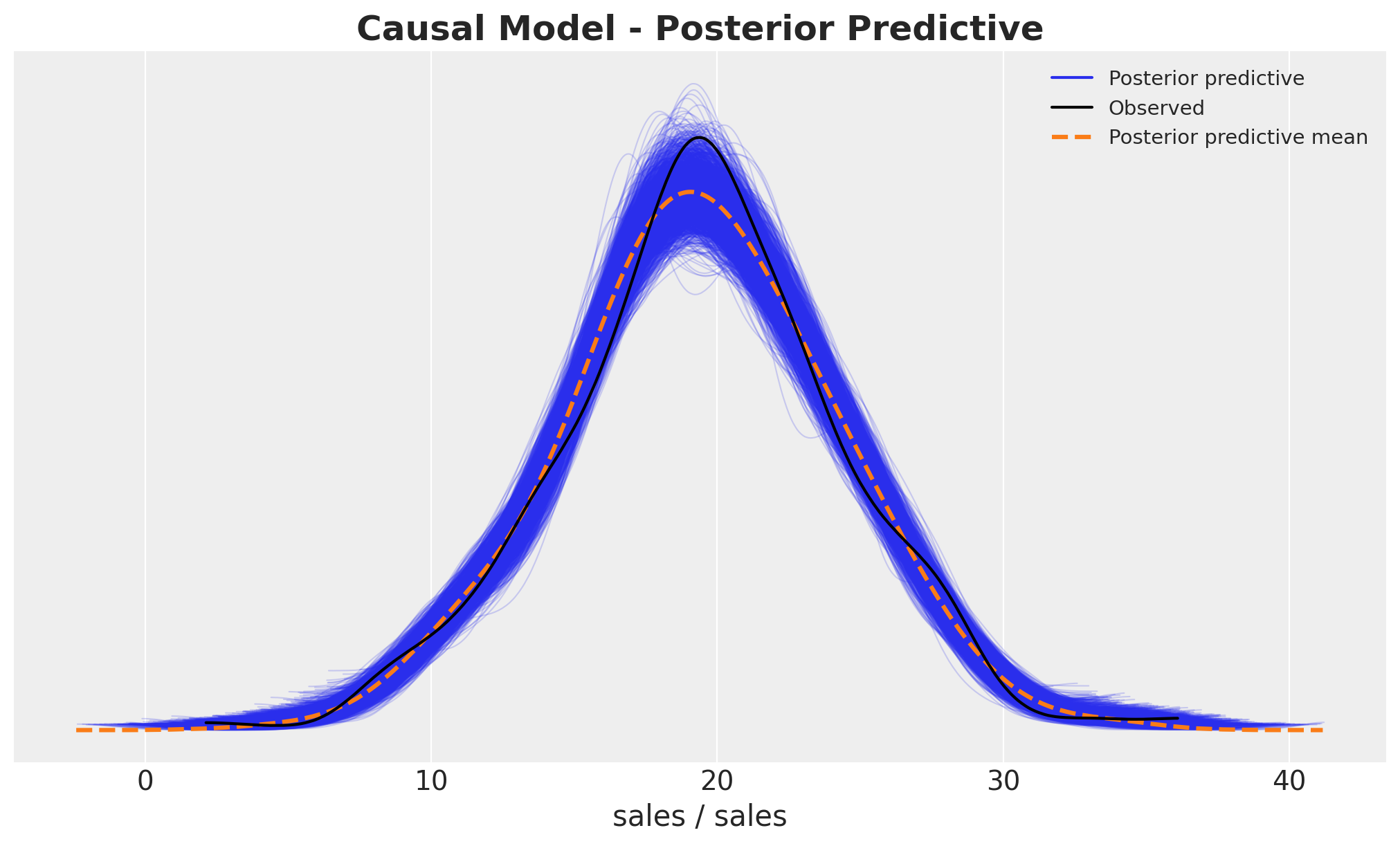
Using Data Science For Bad Decision Making A Case Study Dr Juan To motivate and introduce causal inference in introductory statistics or data science courses, we use simulated data and simple linear regression to show the effects of confounding and when one should or should not adjust for covariables. David a. freedman presents here a definitive synthesis of his approach to causal inference in the social sciences. he explores the foundations and limitations of statistical modeling, illustrating basic arguments with examples from political science, public policy, law, and epidemiology.