Assessing Fine Tuning Efficacy In Llms A Case Study With Learning Get ready for a power packed nugget of wisdom from harpreet sahota as we talk about augmenting your generative ai model with new data on “what's the 𝘽𝙐𝙕?. When creating and training ai models, developers face the question of whether to use rag, fine tuning, or both. here’s a closer look at retrieval augmented generation vs. fine tuning and how to determine the best technique for your use case.
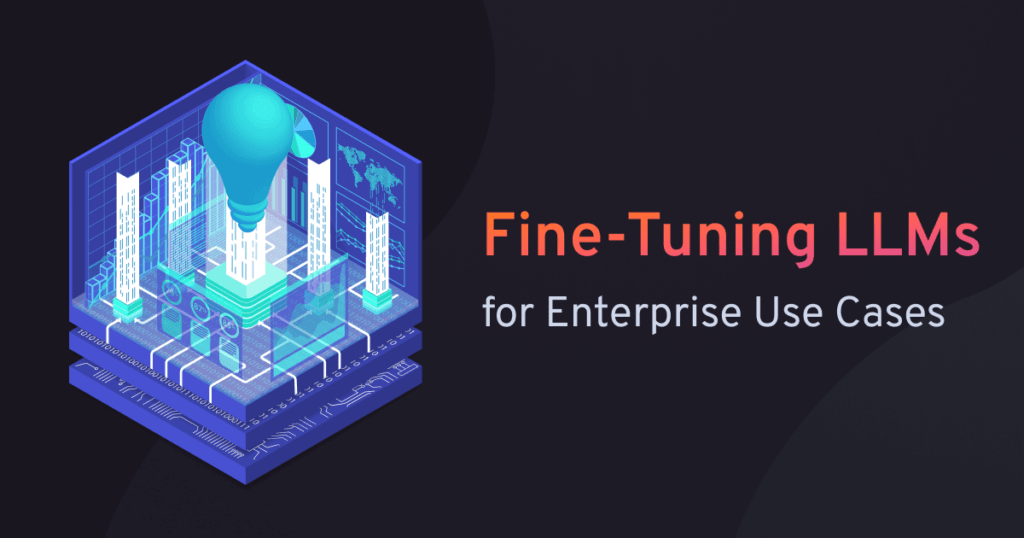
Fine Tuning Llms Methods And Use Cases In this article, i’ll explain what tuning an llm is, the different types of tuning, including few shot learning, their pros and cons, and when to use each type. i will end the article by. Anyone who has used chatgpt extensively or other llms in the market has seen that, especially for domain specific tasks, using llms straight out of the box can fall flat. here are some challenges and limitations of llms: perhaps the most common issue we encounter is hallucinations. What are the pros & cons of fine tuning llms? (guest: harpreet sahota) buff.ly 3rig84g via @ andreas.m.welschgmail #ai #digitaltransformation #generativeai …more. Fine tuning llms sounds great, but it’s costly, complex, and often unnecessary. discover why smarter alternatives like nventr ai can be more efficient and cost effective.
Thinkers360 On Linkedin What Are The Pros Cons Of Fine Tuning Llms What are the pros & cons of fine tuning llms? (guest: harpreet sahota) buff.ly 3rig84g via @ andreas.m.welschgmail #ai #digitaltransformation #generativeai …more. Fine tuning llms sounds great, but it’s costly, complex, and often unnecessary. discover why smarter alternatives like nventr ai can be more efficient and cost effective. In today’s fast paced ai landscape, developers face critical decisions when it comes to optimizing llms. a big one is determining when to fine tune and when to self host llm models as each option can significantly impact cost, data security, and performance. Fine tuning demands precise adjustment of multiple technical variables. key parameters include learning rates, batch sizes, and training epochs. for massive models exceeding 100 million parameters, specialized techniques like low rank adaptation (lora) offer efficient alternatives. Advantages of fine tuning llms. we will start off by discussing some of the main advantages of fine tuning llms. specifically, we will focus on advantages that fine tuning has over other methods like prompt engineering. lower latency. one of the largest advantages of fine tuning llms is not actually related to improving the predictive. Best practices: effective fine tuning involves several key considerations: high quality, domain specific data: this is crucial for achieving accurate and relevant results. careful hyperparameter selection: optimizing learning rate, batch size, and other parameters ensures efficient training.
Introduction To Fine Tuning Llms Fine Tune Your Llms Video Tutorial In today’s fast paced ai landscape, developers face critical decisions when it comes to optimizing llms. a big one is determining when to fine tune and when to self host llm models as each option can significantly impact cost, data security, and performance. Fine tuning demands precise adjustment of multiple technical variables. key parameters include learning rates, batch sizes, and training epochs. for massive models exceeding 100 million parameters, specialized techniques like low rank adaptation (lora) offer efficient alternatives. Advantages of fine tuning llms. we will start off by discussing some of the main advantages of fine tuning llms. specifically, we will focus on advantages that fine tuning has over other methods like prompt engineering. lower latency. one of the largest advantages of fine tuning llms is not actually related to improving the predictive. Best practices: effective fine tuning involves several key considerations: high quality, domain specific data: this is crucial for achieving accurate and relevant results. careful hyperparameter selection: optimizing learning rate, batch size, and other parameters ensures efficient training.

Fine Tuning Llms Project Image To U Advantages of fine tuning llms. we will start off by discussing some of the main advantages of fine tuning llms. specifically, we will focus on advantages that fine tuning has over other methods like prompt engineering. lower latency. one of the largest advantages of fine tuning llms is not actually related to improving the predictive. Best practices: effective fine tuning involves several key considerations: high quality, domain specific data: this is crucial for achieving accurate and relevant results. careful hyperparameter selection: optimizing learning rate, batch size, and other parameters ensures efficient training.